Visualising uncertainty in brightfield to fluorescent image inference with BFNet
biorxiv(2020)
摘要
Predicting fluorescently labelled cellular structures from brightfield images is a recent application of convolutional neural networks and has already proven a valuable tool in biological imaging studies. These methods reduce the need for time-consuming manual annotations for supervised datasets, potentially reduce the costs in high-throughput imaging screens, and open up a number of potentially novel analyses. However as with any prediction there can be sources of error and uncertainty. Here we present BFNet, a method to visualise the uncertainty in predicted images by applying Monte Carlo dropout during inference and calculating per-pixel variance as an uncertainty map. Our method demonstrates the ability to highlight regions of an image where prediction is difficult or impossible due to imaging artefacts such as occlusions or out-of-focus images, as well as more general uncertainty when a trained model is applied to new data from different imaging of experimental settings. We have provided a python implementation of the method which is available at .
更多查看译文
关键词
fluorescent image inference,brightfield,uncertainty
AI 理解论文
溯源树
样例
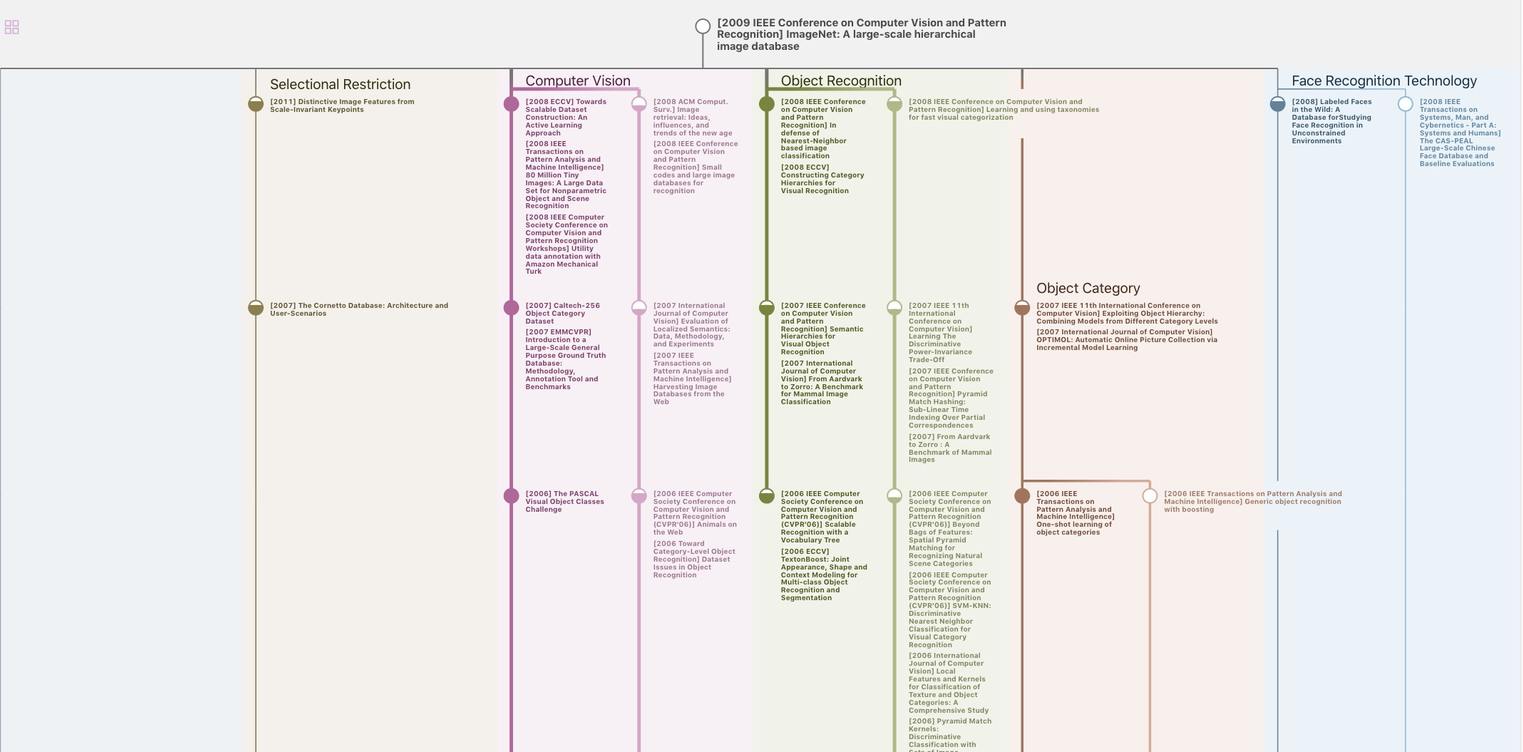
生成溯源树,研究论文发展脉络
Chat Paper
正在生成论文摘要