Leveraging non-structural data to predict structures of protein–ligand complexes
biorxiv(2020)
摘要
Structure-based drug design depends on the ability to predict the three-dimensional structure of ligands bound to their targets, as does understanding the molecular mechanisms of many essential biological processes. Dozens of computational docking methods have been developed to address this binding pose prediction problem, but they frequently produce inaccurate results. Here we present a method that substantially improves the accuracy of binding pose prediction by exploiting a widely available source of non-structural information: a list of other ligands that bind the same target. Our method, ComBind, quantifies and leverages the chemist’s intuition that even very different ligands tend to form similar interactions with a target protein. We demonstrate that ComBind consistently increases pose prediction accuracy across all major families of drug targets. We also illustrate its use by predicting previously unknown binding poses of antipsychotics and validating these results experimentally.
更多查看译文
AI 理解论文
溯源树
样例
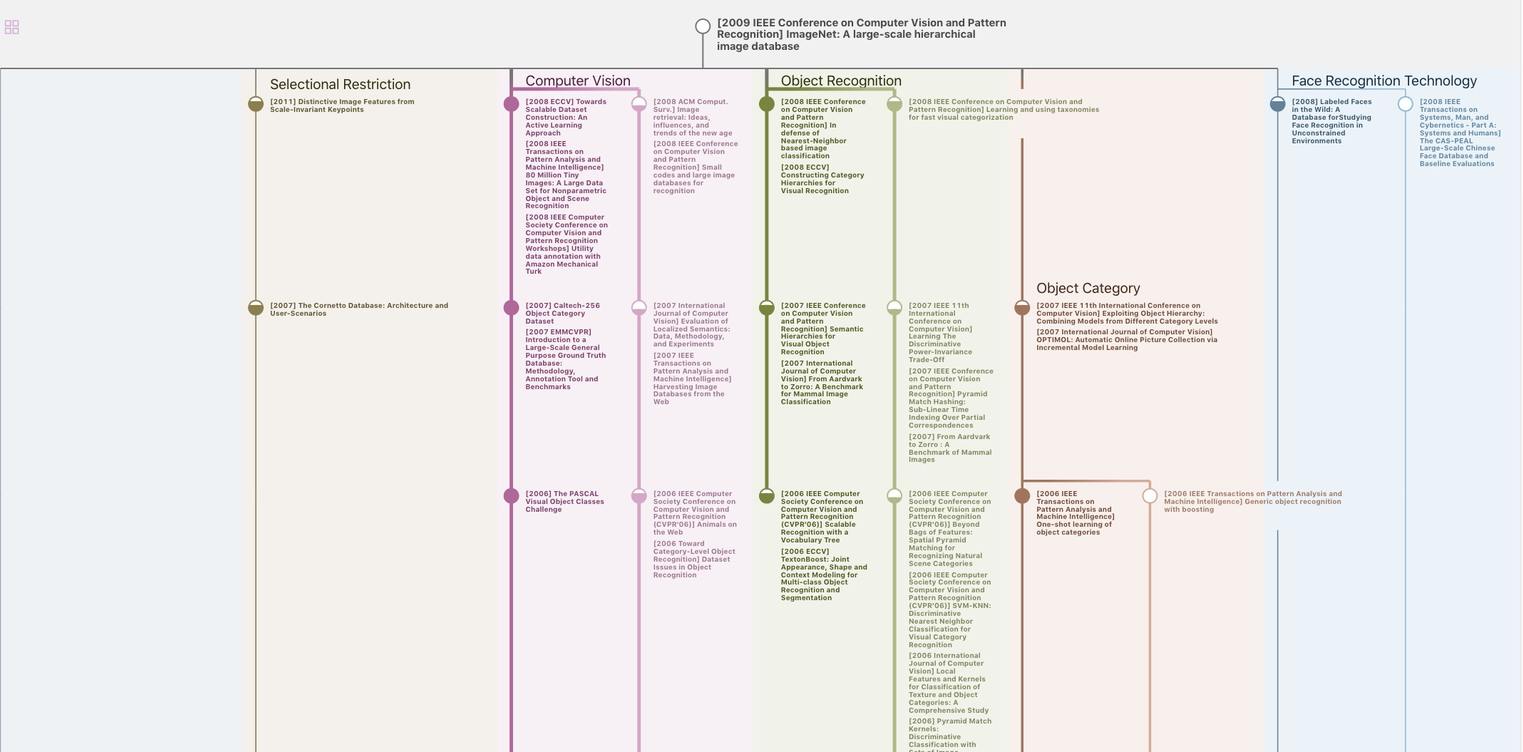
生成溯源树,研究论文发展脉络
Chat Paper
正在生成论文摘要