A consistent evaluation of miRNA-disease association prediction models
biorxiv(2020)
摘要
Motivation A variety of machine learning based approaches have been applied to predicting miRNA-disease association. Although promising, the evaluation set up to measure prediction performance is inconsistent making it difficult to assess the actual progress. A more acute problem is that most of the models overlook the problem of data leakage due to the use of precomputed miRNA and disease similarity features.
Results We unearth a crucial problem of data leakage in evaluation of machine learning models for miRNA-disease association prediction. In particular, information from test set, in the form of precomputed input features for miRNA and disease, is used during training of the model. Moreover, we point out problems in the widely used performance metrics used in model evaluation. While resolving the issues of data leakage and model evaluation, we perform an indepth study of 3 recent models along with our proposed 9 variants of these models. Our proposed variants have resulted in improvements in Average Precision scores (as compared to original models) by approximately 287.7% and 36.7% on HMDDv2.0 (AP:0.504) and HMDDv3.0 (AP: 0.216) datasets respectively.
Availability and Implementation We release a unified evaluation framework including all models and datasets at [https://git.l3s.uni-hannover.de/dong/simplifying\_mirna\_disease][1].
### Competing Interest Statement
The authors have declared no competing interest.
[1]: https://git.l3s.uni-hannover.de/dong/simplifying_mirna_disease
更多查看译文
AI 理解论文
溯源树
样例
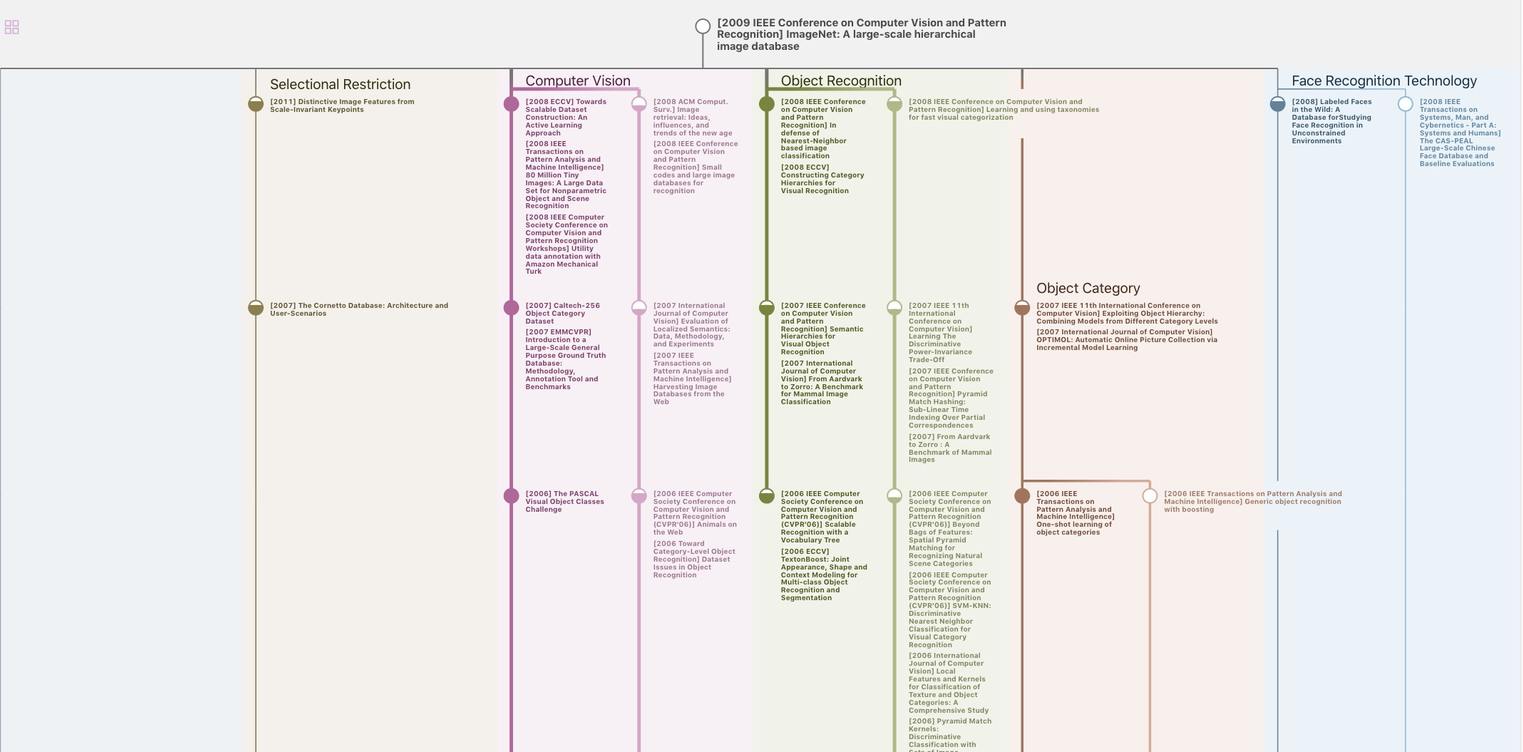
生成溯源树,研究论文发展脉络
Chat Paper
正在生成论文摘要