Neural state space alignment for magnitude generalization in humans and recurrent networks
Neuron(2021)
摘要
A prerequisite for intelligent behavior is to understand how stimuli are related and to generalize this knowledge across contexts. Generalization can be challenging when relational patterns are shared across contexts but exist on different physical scales. Here, we studied neural representations in humans and recurrent neural networks performing a magnitude comparison task, for which it was advantageous to generalize concepts of “more” or “less” between contexts. Using multivariate analysis of human brain signals and of neural network hidden unit activity, we observed that both systems developed parallel neural “number lines” for each context. In both model systems, these number state spaces were aligned in a way that explicitly facilitated generalization of relational concepts (more and less). These findings suggest a previously overlooked role for neural normalization in supporting transfer of a simple form of abstract relational knowledge (magnitude) in humans and machine learning systems.
更多查看译文
关键词
magnitude,alignment,representation,number,parietal cortex,generalization,normalization,neural network
AI 理解论文
溯源树
样例
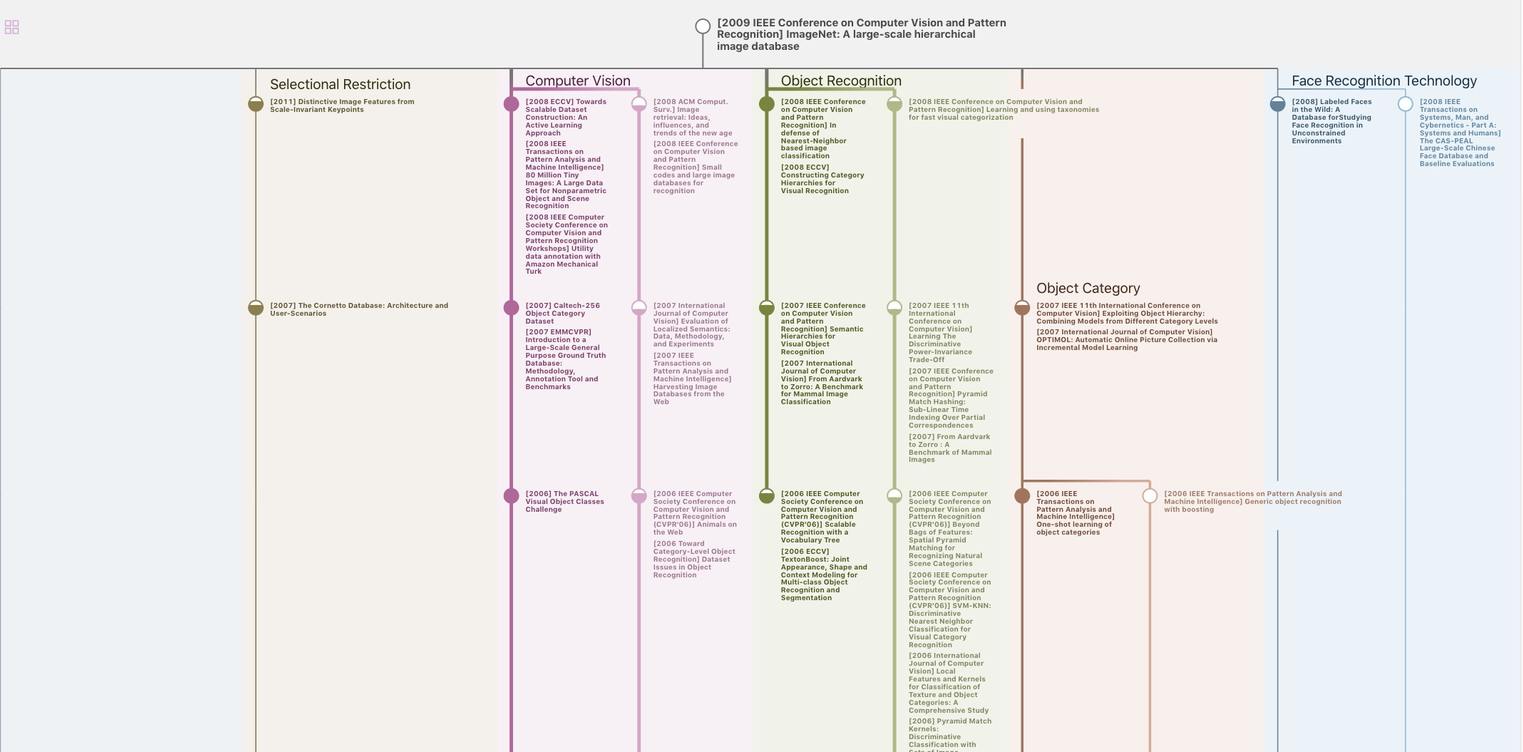
生成溯源树,研究论文发展脉络
Chat Paper
正在生成论文摘要