Artificial intelligence-based computational framework for drug-target prioritization and inference of novel repositionable drugs for Alzheimer’s disease
biorxiv(2021)
摘要
Background Identifying novel therapeutic targets is crucial for the successful development of drugs. However, the cost to experimentally identify therapeutic targets is huge and only approximately 400 genes are targets for FDA-approved drugs. As a result, it is inevitable to develop powerful computational tools that can identify potential novel therapeutic targets. Fortunately, the human protein-protein interaction network (PIN) could be a useful resource to achieve this objective. Methods In this study, we developed a deep learning-based computational framework that extracts low-dimensional representations of high-dimensional PIN data. Our computational framework uses latent features and state-of-the-art machine learning techniques to infer potential drug target genes. Results We applied our computational framework to prioritize novel putative target genes for Alzheimer’s disease and successfully identified key genes that may serve as novel therapeutic targets (e.g., DLG4, EGFR, RAC1, SYK, PTK2B, SOCS1). Furthermore, based on these putative targets, we could infer repositionable candidate-compounds for the disease (e.g., tamoxifen, bosutinib, and dasatinib). Conclusions Our deep learning-based computational framework could be a powerful tool to efficiently prioritize new therapeutic targets and enhance the drug repositioning strategy.
更多查看译文
关键词
network bedding,deep learning,machine learning,systems biology,drug discovery,protein interaction network
AI 理解论文
溯源树
样例
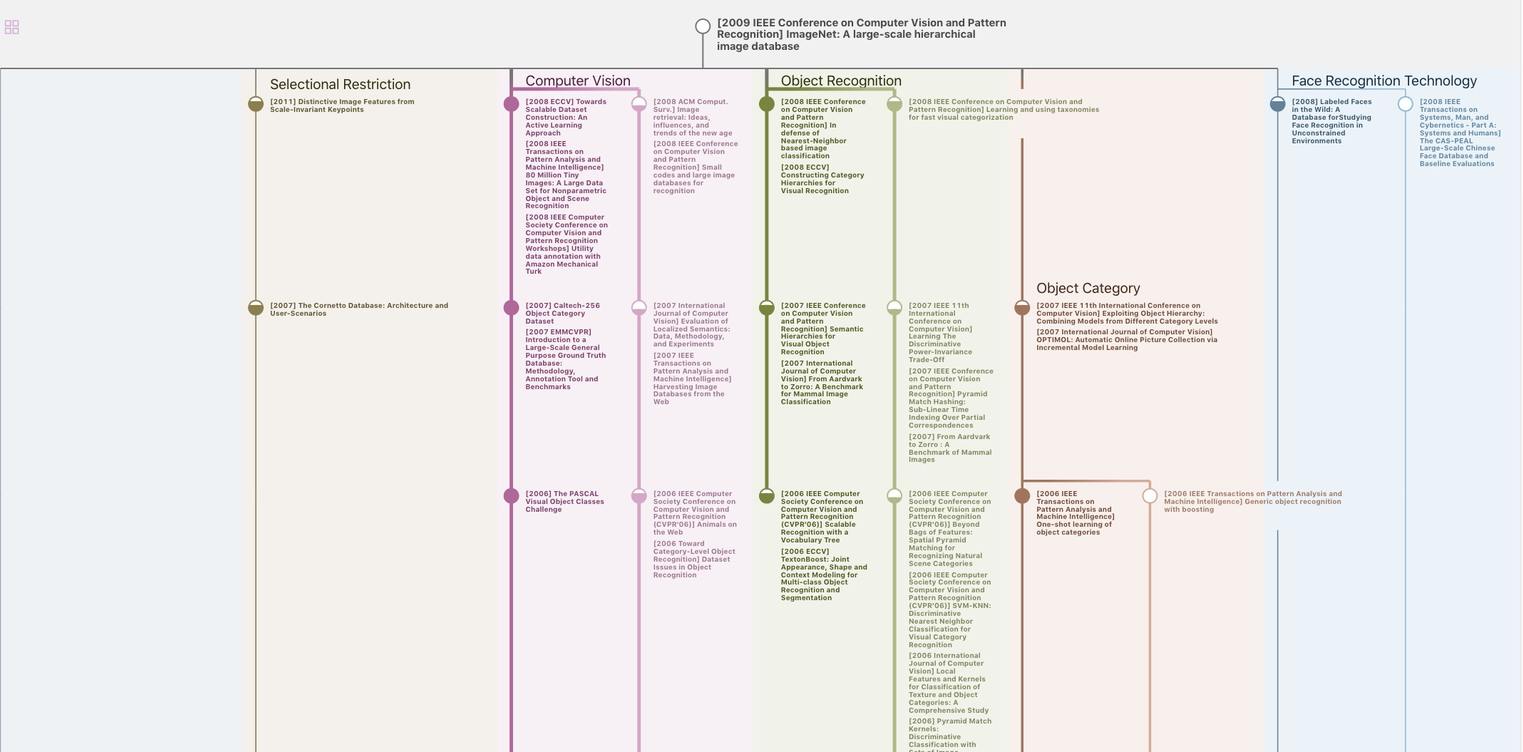
生成溯源树,研究论文发展脉络
Chat Paper
正在生成论文摘要