Deep Graph Pose: a semi-supervised deep graphical model for improved animal pose tracking.
NIPS 2020(2020)
摘要
Noninvasive behavioral tracking of animals is crucial for many scientific investigations. Recent transfer learning approaches for behavioral tracking have considerably advanced the state of the art. Typically these methods treat each video frame and each object to be tracked independently. In this work, we improve on these methods (particularly in the regime of few training labels) by leveraging the rich spatiotemporal structures pervasive in behavioral video — specifically, the spatial statistics imposed by physical constraints (e.g., paw to elbow distance), and the temporal statistics imposed by smoothness from frame to frame. We propose a probabilistic graphical model built on top of deep neural networks, Deep Graph Pose (DGP), to leverage these useful spatial and temporal constraints, and develop an efficient structured variational approach to perform inference in this model. The resulting semi-supervised model exploits both labeled and unlabeled frames to achieve significantly more accurate and robust tracking while requiring users to label fewer training frames. In turn, these tracking improvements enhance performance on downstream applications, including robust unsupervised segmentation of behavioral “syllables,” and estimation of interpretable “disentangled” low-dimensional representations of the full behavioral video.
更多查看译文
关键词
deep graph pose,tracking,improved animal,semi-supervised
AI 理解论文
溯源树
样例
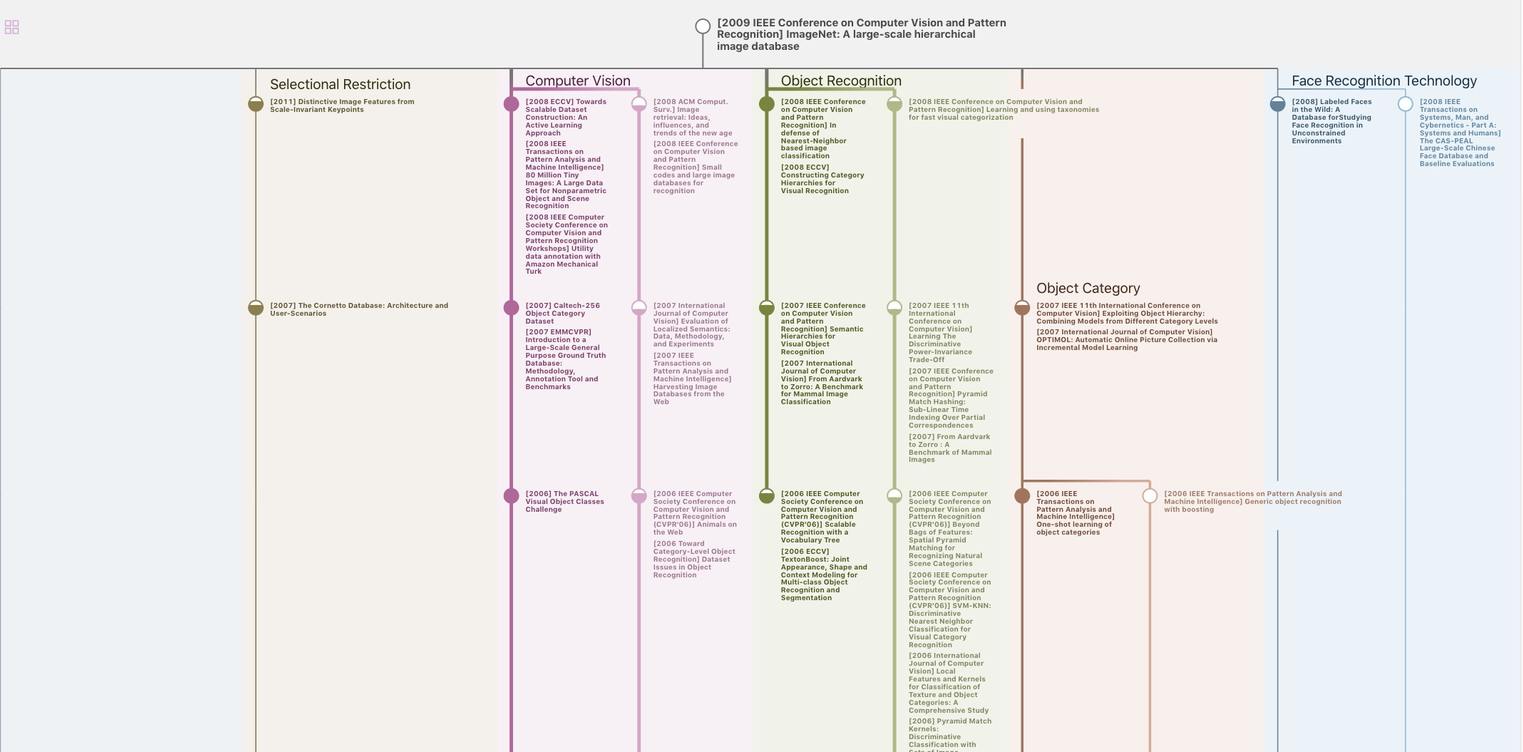
生成溯源树,研究论文发展脉络
Chat Paper
正在生成论文摘要