Automatic Subtyping Of Individuals With Primary Progressive Aphasia
JOURNAL OF ALZHEIMERS DISEASE(2021)
摘要
Background: The classification of patients with primary progressive aphasia (PPA) into variants is time-consuming, costly, and requires combined expertise by clinical neurologists, neuropsychologists, speech pathologists, and radiologists.Objective: The aim of the present study is to determine whether acoustic and linguistic variables provide accurate classification of PPA patients into one of three variants: nonfluent PPA, semantic PPA, and logopenic PPA.Methods: In this paper, we present a machine learning model based on deep neural networks (DNN) for the subtyping of patients with PPA into three main variants, using combined acoustic and linguistic information elicited automatically via acoustic and linguistic analysis. The performance of the DNN was compared to the classification accuracy of Random Forests, Support Vector Machines, and Decision Trees, as well as to expert clinicians' classifications.Results: The DNN model outperformed the other machine learning models as well as expert clinicians' classifications with 80% classification accuracy. Importantly, 90% of patients with nfvPPA and 95% of patients with 1vPPA was identified correctly, providing reliable subtyping of these patients into their corresponding PPA variants.Conclusion: We show that the combined speech and language markers from connected speech productions can inform variant subtyping in patients with PPA. The end-to-end automated machine learning approach we present can enable clinicians and researchers to provide an easy, quick, and inexpensive classification of patients with PPA.
更多查看译文
关键词
Classification, machine learning, natural language processing, primary progressive aphasia
AI 理解论文
溯源树
样例
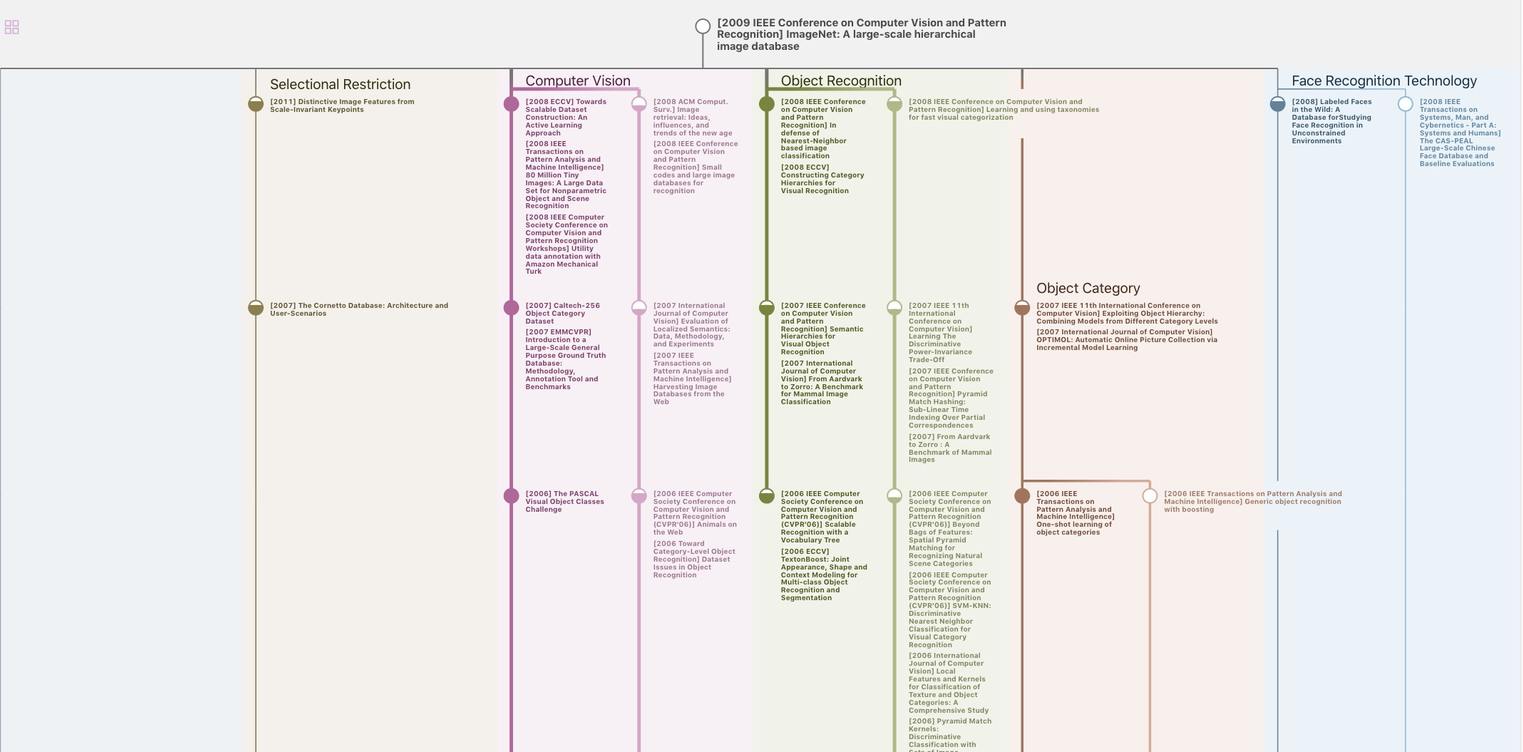
生成溯源树,研究论文发展脉络
Chat Paper
正在生成论文摘要