Computational target fishing by mining transcriptional data using a novel Siamese spectral-based graph convolutional network
biorxiv(2020)
摘要
Computational target fishing aims to investigate the mechanism of action or the side effects of bioactive small molecules. Unfortunately, conventional ligand-based computational methods only explore a confined chemical space, and structure-based methods are limited by the availability of crystal structures. Moreover, these methods cannot describe cellular context-dependent effects and are thus not useful for exploring the targets of drugs in specific cells. To address these challenges, we propose a novel Siamese spectral-based graph convolutional network (SSGCN) model for inferring the protein targets of chemical compounds from gene transcriptional profiles. Although the gene signature of a compound perturbation only provides indirect clues of the interacting targets, the SSGCN model was successfully trained to learn from known compound-target pairs by uncovering the hidden correlations between compound perturbation profiles and gene knockdown profiles. Using a benchmark set, the model achieved impressive target inference results compared with previous methods such as Connectivity Map and ProTINA. More importantly, the powerful generalization ability of the model observed with the external LINCS phase II dataset suggests that the model is an efficient target fishing or repositioning tool for bioactive compounds.
更多查看译文
关键词
transcriptional data,computational target,graph,spectral-based
AI 理解论文
溯源树
样例
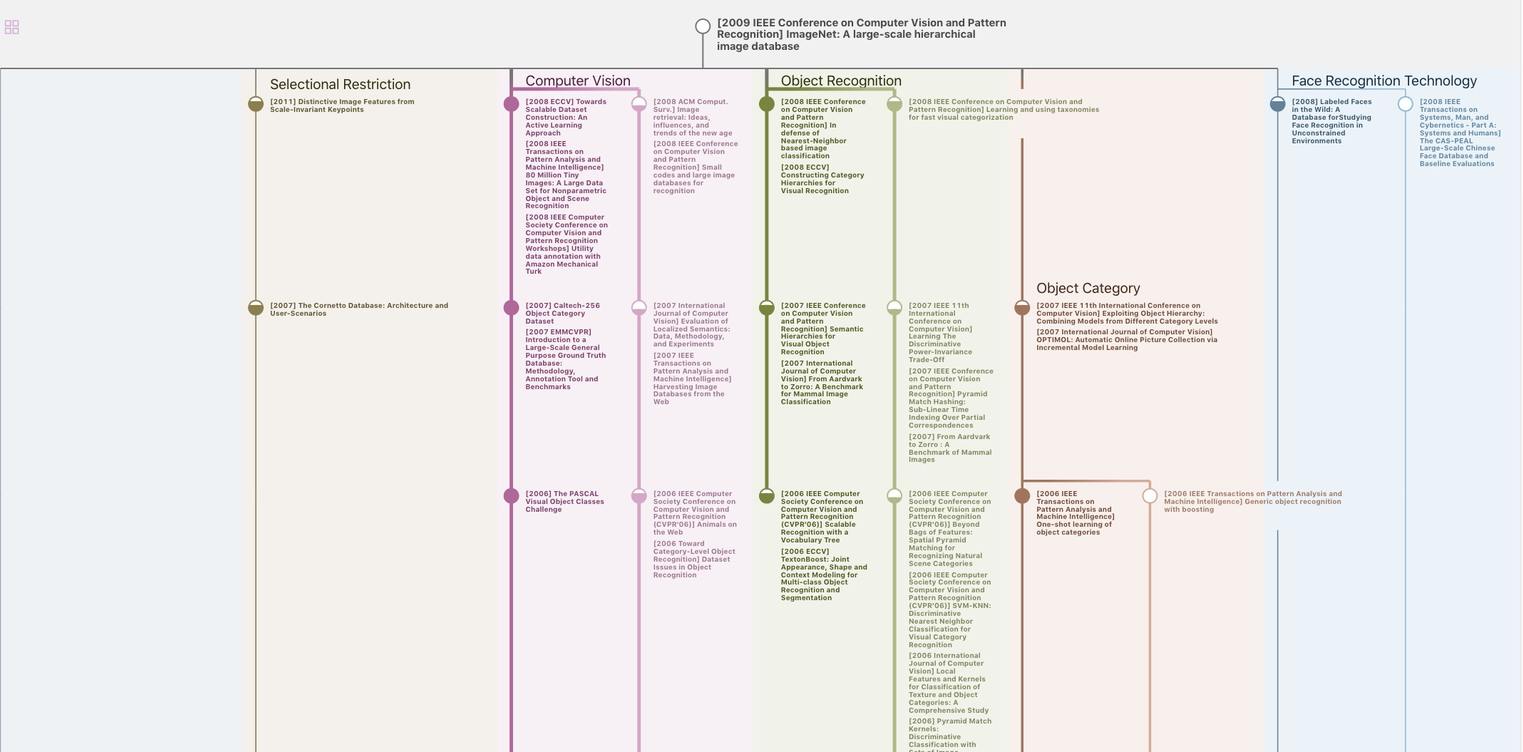
生成溯源树,研究论文发展脉络
Chat Paper
正在生成论文摘要