Data-Driven Reduction Of Dendritic Morphologies With Preserved Dendro-Somatic Responses
ELIFE(2021)
摘要
Dendrites shape information flow in neurons. Yet, there is little consensus on the level of spatial complexity at which they operate. Through carefully chosen parameter fits, solvable in the least-squares sense, we obtain accurate reduced compartmental models at any level of complexity. We show that (back-propagating) action potentials, Ca2+ spikes, and N-methyl-D-aspartate spikes can all be reproduced with few compartments. We also investigate whether afferent spatial connectivity motifs admit simplification by ablating targeted branches and grouping affected synapses onto the next proximal dendrite. We find that voltage in the remaining branches is reproduced if temporal conductance fluctuations stay below a limit that depends on the average difference in input resistance between the ablated branches and the next proximal dendrite. Furthermore, our methodology fits reduced models directly from experimental data, without requiring morphological reconstructions. We provide software that automatizes the simplification, eliminating a common hurdle toward including dendritic computations in network models.
更多查看译文
关键词
dendritic computation,model reduction,neuron models,neuroscience,none,software toolbox
AI 理解论文
溯源树
样例
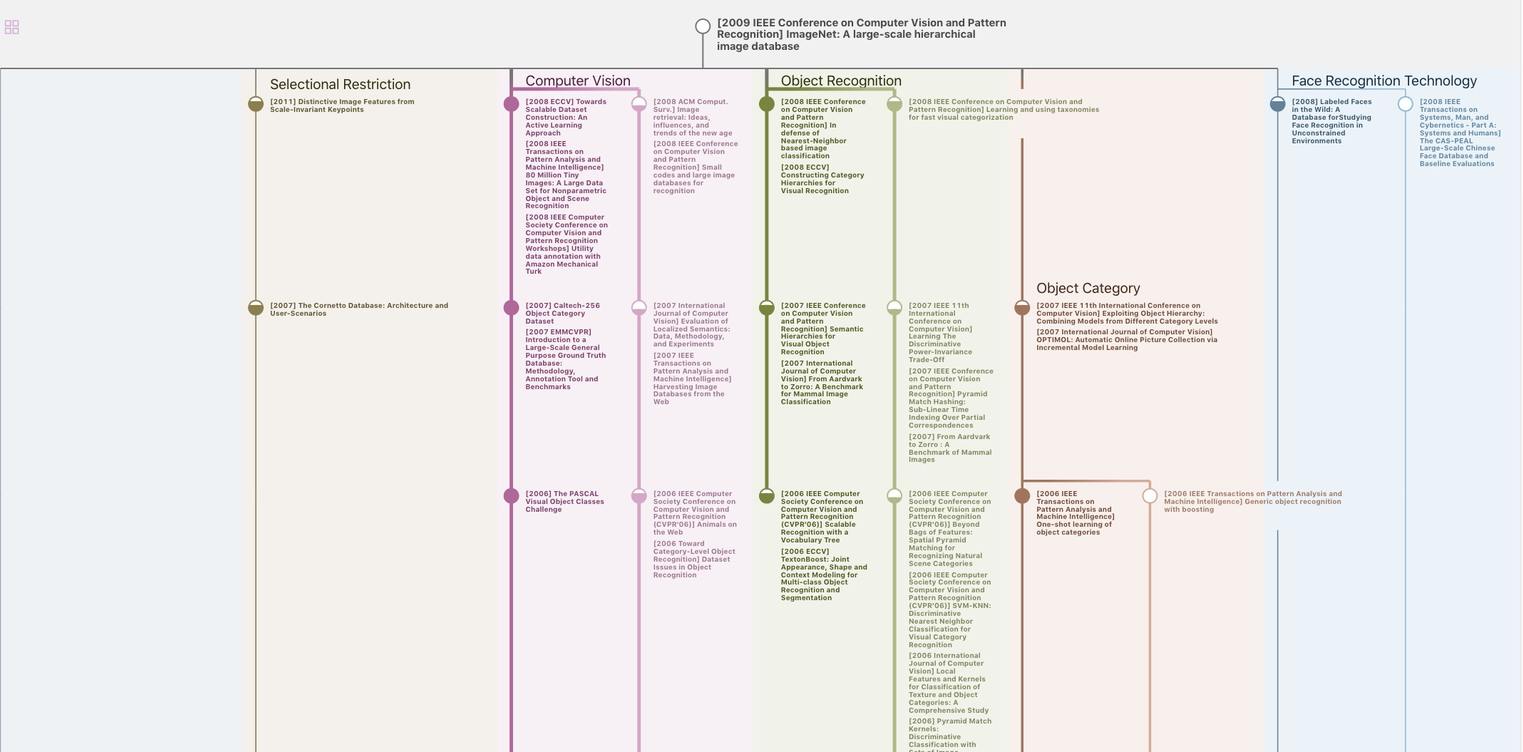
生成溯源树,研究论文发展脉络
Chat Paper
正在生成论文摘要