A Deep Learning Pipeline for Nucleus Segmentation
CYTOMETRY PART A(2020)
摘要
Deep learning is rapidly becoming the technique of choice for automated segmentation of nuclei in biological image analysis workflows. In order to evaluate the feasibility of training nuclear segmentation models on small, custom annotated image datasets that have been augmented, we have designed a computational pipeline to systematically compare different nuclear segmentation model architectures and model training strategies. Using this approach, we demonstrate that transfer learning and tuning of training parameters, such as the composition, size, and preprocessing of the training image dataset, can lead to robust nuclear segmentation models, which match, and often exceed, the performance of existing, off-the-shelf deep learning models pretrained on large image datasets. We envision a practical scenario where deep learning nuclear segmentation models trained in this way can be shared across a laboratory, facility, or institution, and continuously improved by training them on progressively larger and varied image datasets. Our work provides computational tools and a practical framework for deep learning-based biological image segmentation using small annotated image datasets. Published [2020]. This article is a U.S. Government work and is in the public domain in the USA
更多查看译文
关键词
deep learning,machine learning,nucleus segmentation,fluorescence microscopy,high‐,content imaging
AI 理解论文
溯源树
样例
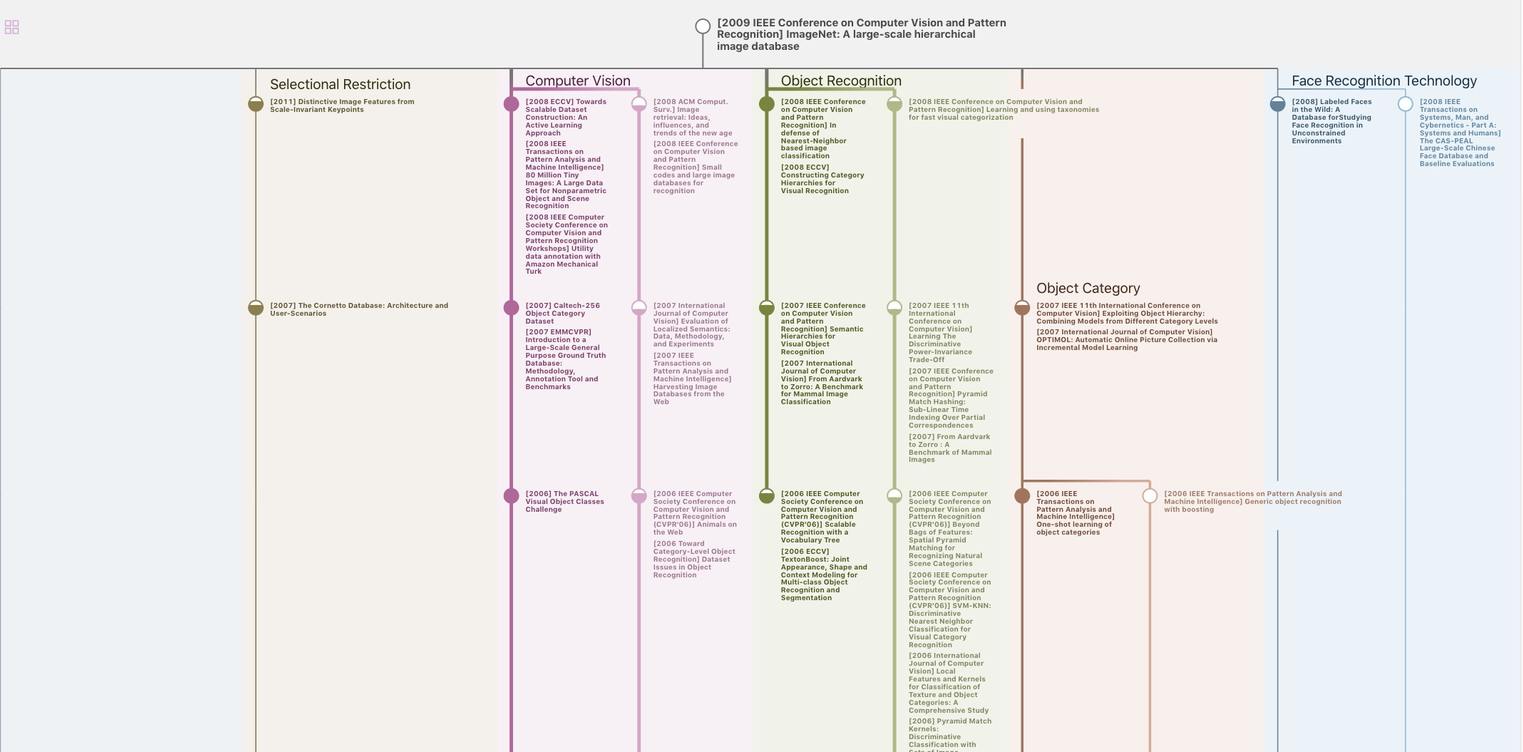
生成溯源树,研究论文发展脉络
Chat Paper
正在生成论文摘要