Optical Aberration Correction via Phase Diversity and Deep Learning
biorxiv(2020)
摘要
In modern microscopy imaging systems, optical components are carefully designed to obtain diffraction-limited resolution. However, live imaging of large biological samples rarely attains this limit because of sample induced refractive index inhomogeneities that create unknown temporally variant optical aberrations. Importantly, these aberrations are also spatially variant, thus making it challenging to correct over wide fields of view. Here, we present a framework for deep-learning based wide-field optical aberration sensing and correction. Our model consists of two modules which take in a set of three phase-diverse images and (i) estimate the wavefront phase in terms of its constituent Zernike polynomial coefficients and (ii) perform blind-deconvolution to yield an aberration-free image. First, we demonstrate our framework on simulations that incorporate optical aberrations, spatial variance, and realistic modelling of sensor noise. We find that our blind deconvolution achieves a 2-fold improvement in frequency support compared to input images, and our phase-estimation achieves a coefficient of determination () of at least 80% when estimating astigmatism, spherical aberration and coma. Second, we show that our results mostly hold for strongly varying spatially-variant aberrations with a 30% resolution improvement. Third, we demonstrate practical usability for light-sheet microscopy: we show a 46% increase in frequency support even in imaging regions affected by detection and illumination scattering.
更多查看译文
关键词
phase diversity,aberration,deep learning
AI 理解论文
溯源树
样例
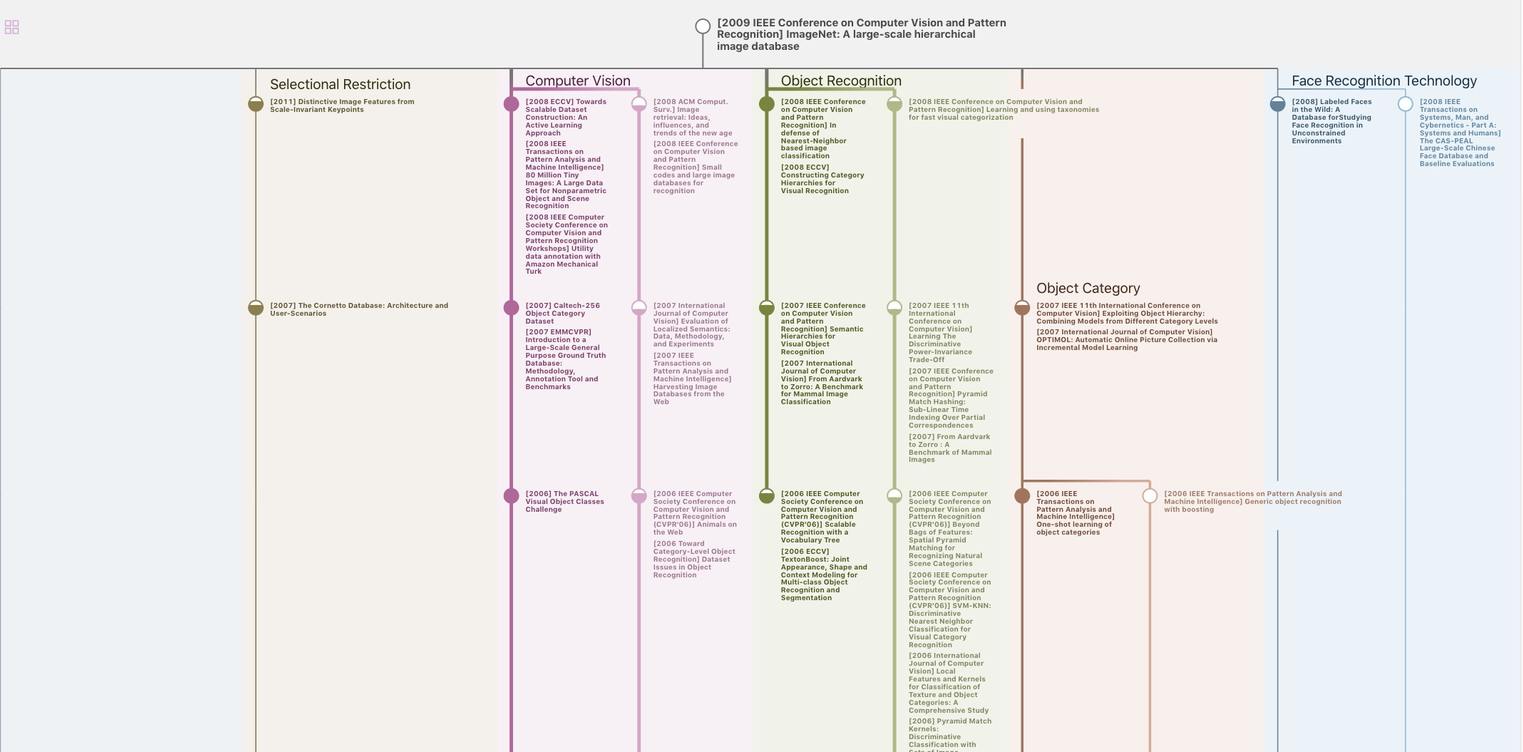
生成溯源树,研究论文发展脉络
Chat Paper
正在生成论文摘要