A Markovian Model-Driven Deep Learning Framework for Massive MIMO CSI Feedback
IEEE Transactions on Wireless Communications(2022)
摘要
Channel state information (CSI) plays a vital role in scheduling and capacity-approaching transmission optimization of massive MIMO communication systems. In frequency division duplex (FDD) MIMO systems, forward link CSI reconstruction at transmitter relies on CSI feedback from receiving nodes and must carefully weigh the tradeoff between reconstruction accuracy and feedback bandwidth. Recent application of recurrent neural networks (RNN) has demonstrated promising results of massive MIMO CSI feedback compression. However, the cost of computation and memory associated with RNN deep learning remains high. In this work, we exploit channel temporal coherence to improve learning accuracy and feedback efficiency. Leveraging a Markovian model, we develop a deep convolutional neural network (CNN)-based framework called MarkovNet to efficiently encode CSI feedback to improve accuracy and efficiency. We explore important physical insights including spherical normalization of input data and deep learning network optimizations in feedback compression. We demonstrate that MarkovNet provides a substantial performance improvement and computational complexity reduction over the RNN-based work. We demonstrate MarkovNet’s performance under different MIMO configurations and for a range of feedback intervals and rates. CSI recovery with MarkovNet outperforms RNN-based CSI estimation with only a fraction of computational cost.
更多查看译文
关键词
Massive MIMO,channel estimation,MarkovNet,compression,model-driven learning
AI 理解论文
溯源树
样例
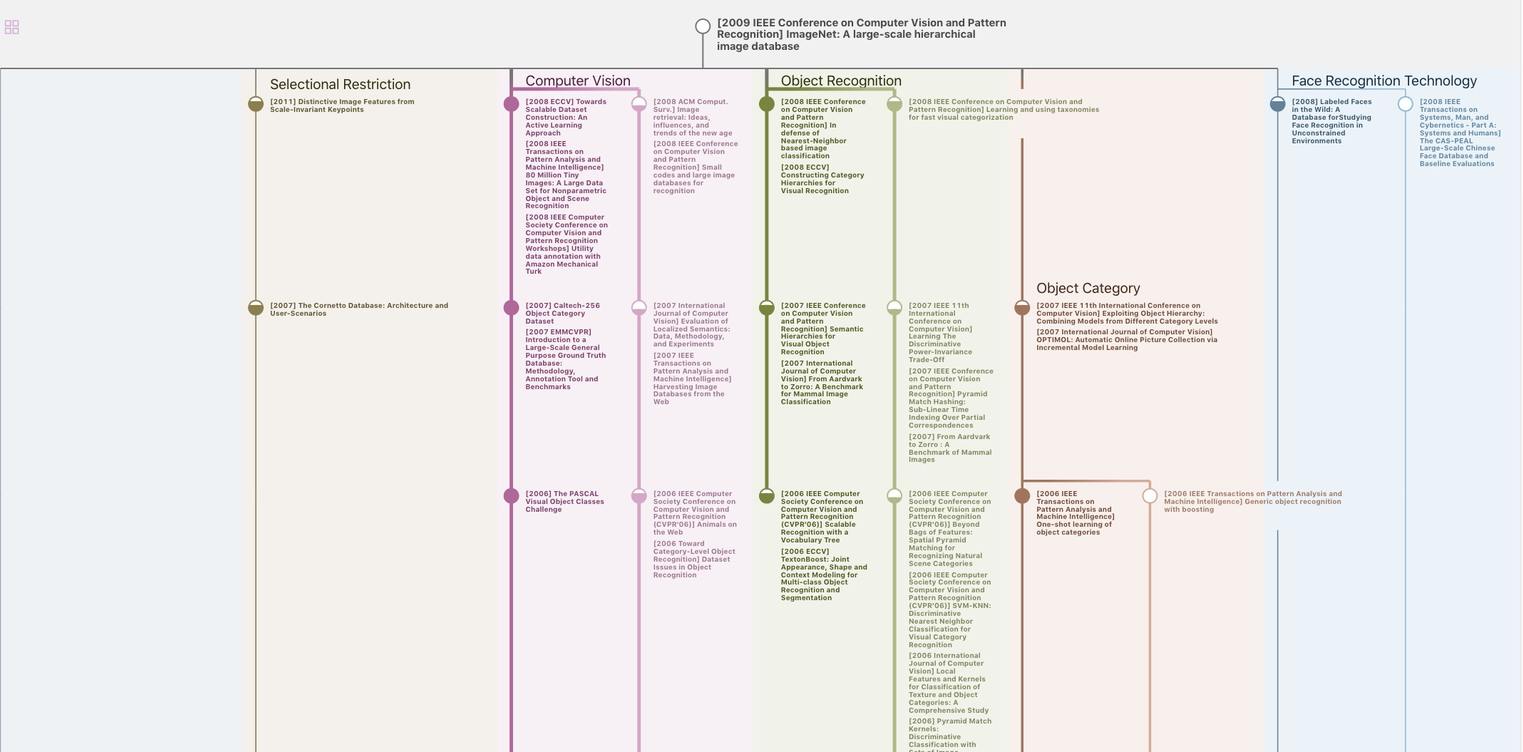
生成溯源树,研究论文发展脉络
Chat Paper
正在生成论文摘要