High-Throughput Functional Annotation of Natural Products by Integrated Activity Profiling
biorxiv(2019)
摘要
Determining mechanism of action (MOA) is one of the biggest challenges in natural products discovery. Here, we report a comprehensive platform that uses Similarity Network Fusion (SNF) to improve MOA predictions by integrating data from the cytological profiling high-content imaging platform and the gene expression platform FUSION. The predictive value of the integrative approach was assessed using a library of target-annotated small molecules as benchmarks. Using KS-tests to compare in-class to out-of-class similarity, we found that SNF resulted in improved power to correctly assign MOA over either dataset alone. Furthermore, we integrated untargeted metabolomics of complex natural product fractions to map biological signatures to specific metabolites. Three examples are presented where SNF coupled with metabolomics was used to directly functionally characterize natural products and accelerate identification of bioactive metabolites. Our results support SNF integration of multiple phenotypic screening approaches along with untargeted metabolomics as powerful approach for advancing natural products drug discovery.
更多查看译文
关键词
metabolomics,natural products,pharmacology
AI 理解论文
溯源树
样例
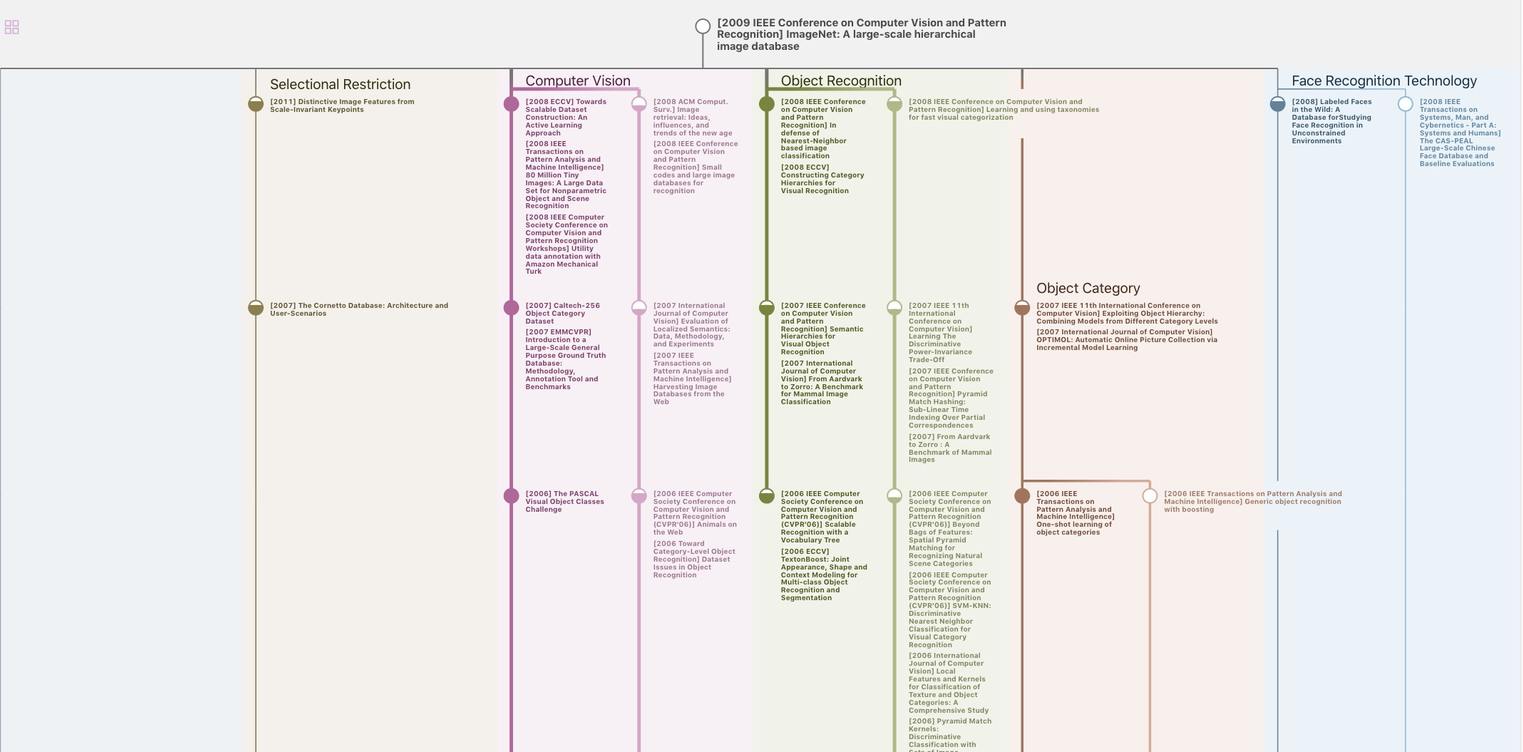
生成溯源树,研究论文发展脉络
Chat Paper
正在生成论文摘要