A Contraction Approach To Model-Based Reinforcement Learning
24TH INTERNATIONAL CONFERENCE ON ARTIFICIAL INTELLIGENCE AND STATISTICS (AISTATS)(2021)
摘要
Despite its experimental success, Model-based Reinforcement Learning still lacks a complete theoretical understanding. To this end, we analyze the error in the cumulative reward using a contraction approach. We consider both stochastic and deterministic state transitions for continuous (non-discrete) state and action spaces. This approach doesn't require strong assumptions and can recover the typical quadratic error to the horizon. We prove that branched rollouts can reduce this error and are essential for deterministic transitions to have a Bellman contraction. Our analysis of policy mismatch error also applies to Imitation Learning. In this case, we show that GAN-type learning has an advantage over Behavioral Cloning when its discriminator is well-trained.
更多查看译文
关键词
reinforcement learning,contraction approach,model-based
AI 理解论文
溯源树
样例
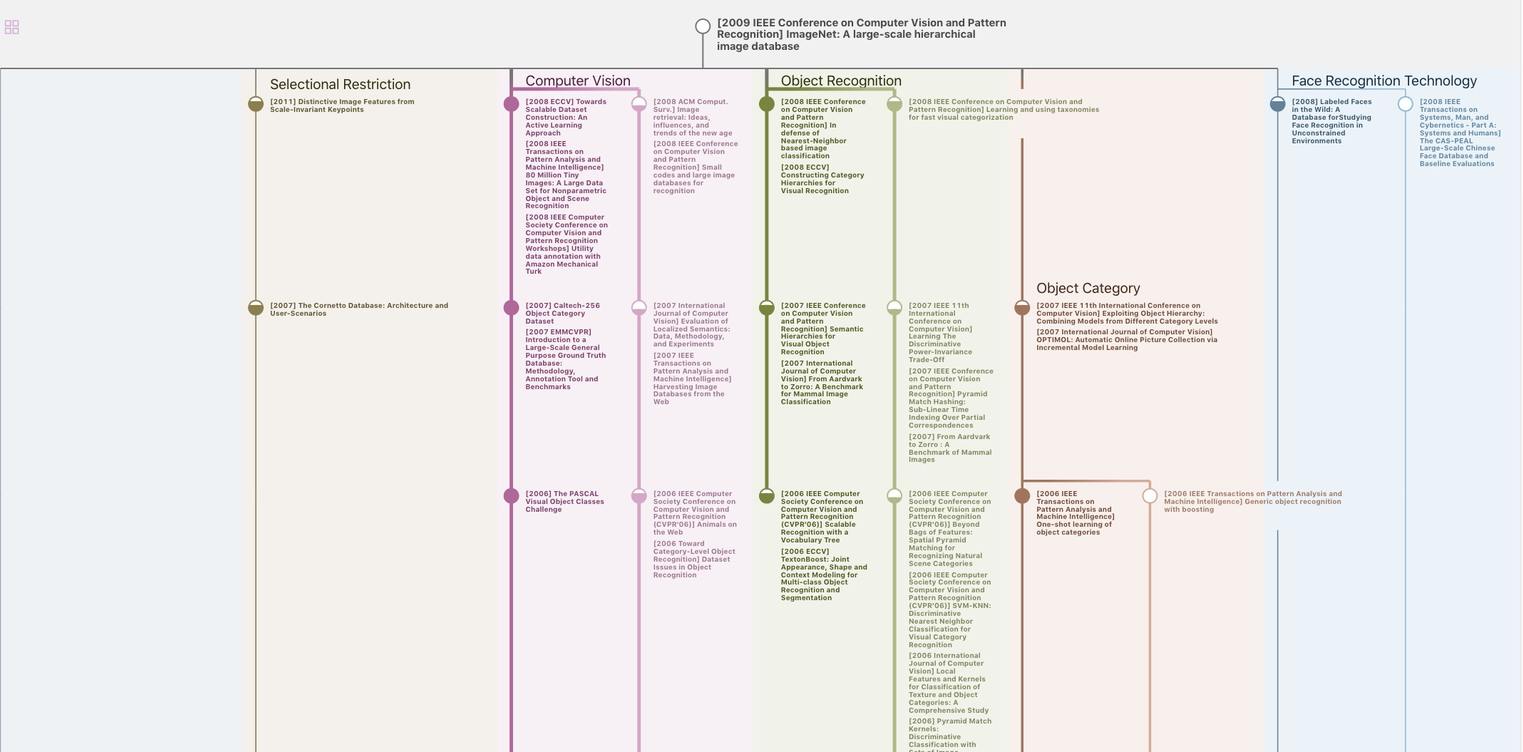
生成溯源树,研究论文发展脉络
Chat Paper
正在生成论文摘要