SHIFT: speedy histological-to-immunofluorescent translation of whole slide images enabled by deep learning
biorxiv(2019)
摘要
Spatially-resolved molecular profiling by immunostaining tissue sections is a key feature in cancer diagnosis, subtyping, and treatment, where it complements routine histopathological evaluation by clarifying tumor phenotypes. In this work, we present a deep learning-based method called speedy histological-to-immunofluorescent translation (SHIFT) which takes histologic images of hematoxylin and eosin-stained tissue as input, then in near-real time returns inferred virtual immunofluorescence (IF) images that accurately depict the underlying distribution of phenotypes without requiring immunostaining of the tissue being tested. We show that deep learning-extracted feature representations of histological images can guide representative sample selection, which improves SHIFT generalizability. SHIFT could serve as an efficient preliminary, auxiliary, or substitute for IF by delivering multiplexed virtual IF images for a fraction of the cost and in a fraction of the time required by nascent multiplexed imaging technologies.
更多查看译文
关键词
Digital pathology,deep learning,generative adversarial networks,hematoxylin and eosin,immunofluorescence
AI 理解论文
溯源树
样例
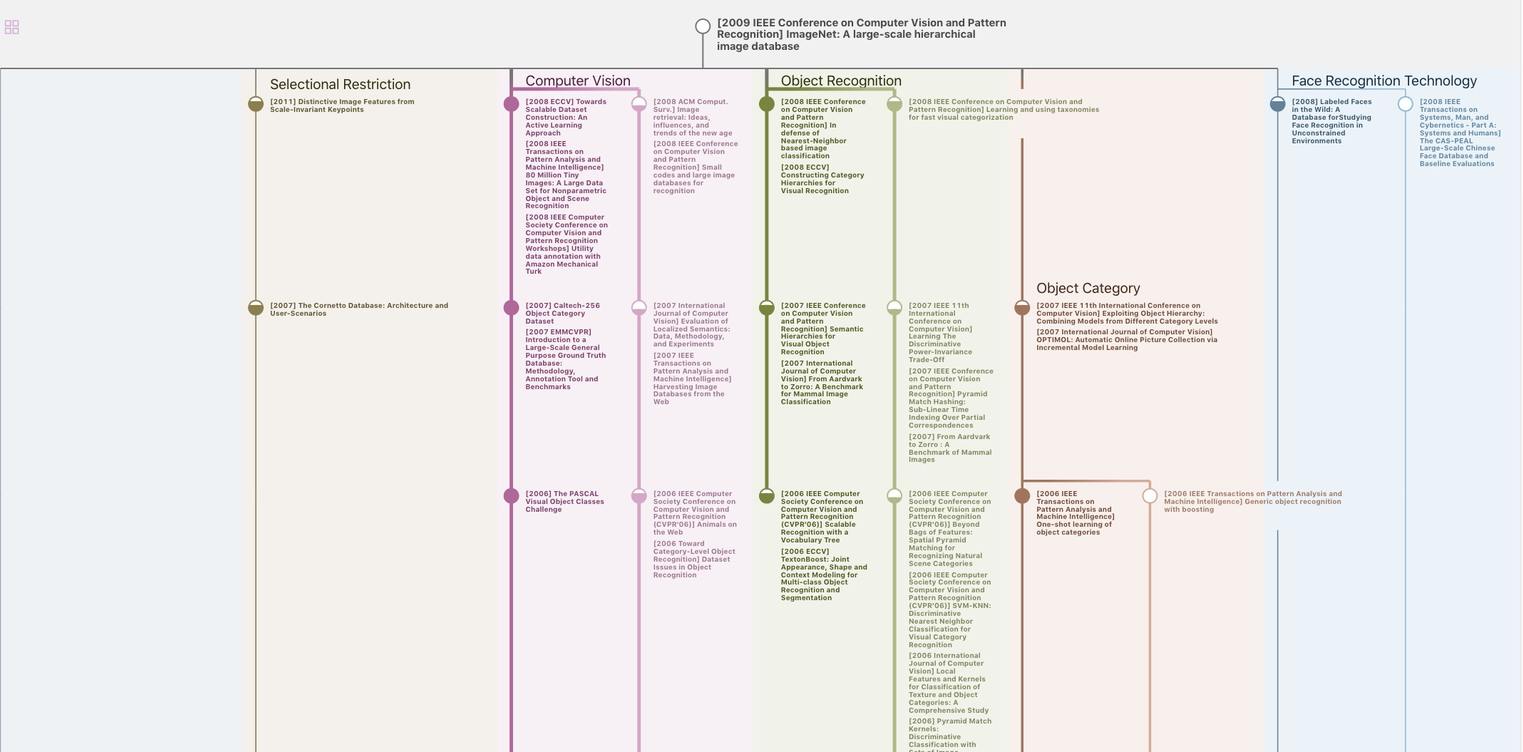
生成溯源树,研究论文发展脉络
Chat Paper
正在生成论文摘要