Robust Aggregation for Adaptive Privacy Preserving Federated Learning in Healthcare
arxiv(2020)
摘要
Federated learning (FL) has enabled training models collaboratively from multiple data owning parties without sharing their data. Given the privacy regulations of patient's healthcare data, learning-based systems in healthcare can greatly benefit from privacy-preserving FL approaches. However, typical model aggregation methods in FL are sensitive to local model updates, which may lead to failure in learning a robust and accurate global model. In this work, we implement and evaluate different robust aggregation methods in FL applied to healthcare data. Furthermore, we show that such methods can detect and discard faulty or malicious local clients during training. We run two sets of experiments using two real-world healthcare datasets for training medical diagnosis classification tasks. Each dataset is used to simulate the performance of three different robust FL aggregation strategies when facing different poisoning attacks. The results show that privacy preserving methods can be successfully applied alongside Byzantine-robust aggregation techniques. We observed in particular how using differential privacy (DP) did not significantly impact the final learning convergence of the different aggregation strategies.
更多查看译文
关键词
federated learning,privacy,aggregation,healthcare
AI 理解论文
溯源树
样例
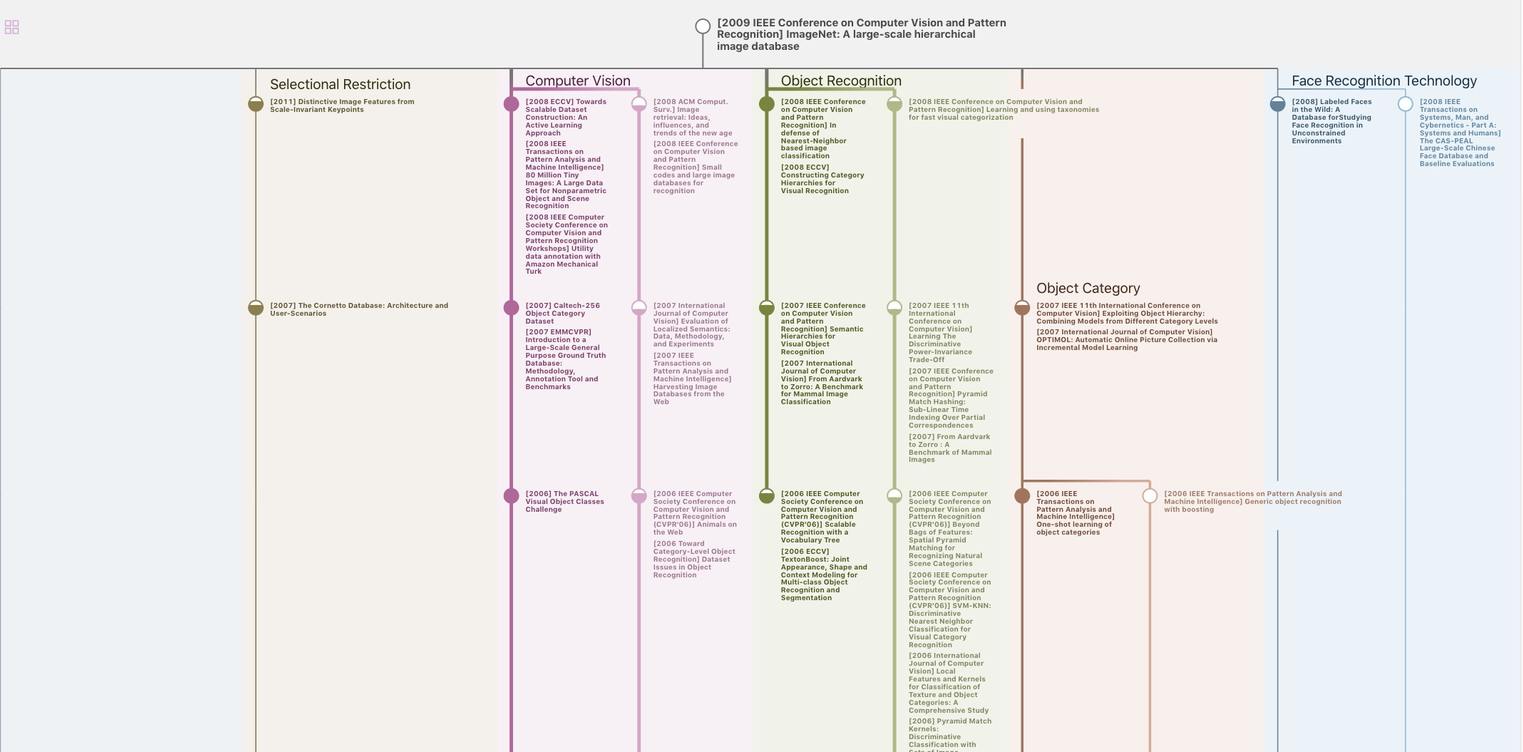
生成溯源树,研究论文发展脉络
Chat Paper
正在生成论文摘要