Reward Maximization Through Discrete Active Inference.
arxiv(2023)
摘要
Active inference is a probabilistic framework for modeling the behavior of biological and artificial agents, which derives from the principle of minimizing free energy. In recent years, this framework has been applied successfully to a variety of situations where the goal was to maximize reward, often offering comparable and sometimes superior performance to alternative approaches. In this article, we clarify the connection between reward maximization and active inference by demonstrating how and when active inference agents execute actions that are optimal for maximizing reward. Precisely, we show the conditions under which active inference produces the optimal solution to the Bellman equation, a formulation that underlies several approaches to model-based reinforcement learning and control. On partially observed Markov decision processes, the standard active inference scheme can produce Bellman optimal actions for planning horizons of 1 but not beyond. In contrast, a recently developed recursive active inference scheme (sophisticated inference) can produce Bellman optimal actions on any finite temporal horizon. We append the analysis with a discussion of the broader relationship between active inference and reinforcement learning.
更多查看译文
关键词
reward,maximization,active
AI 理解论文
溯源树
样例
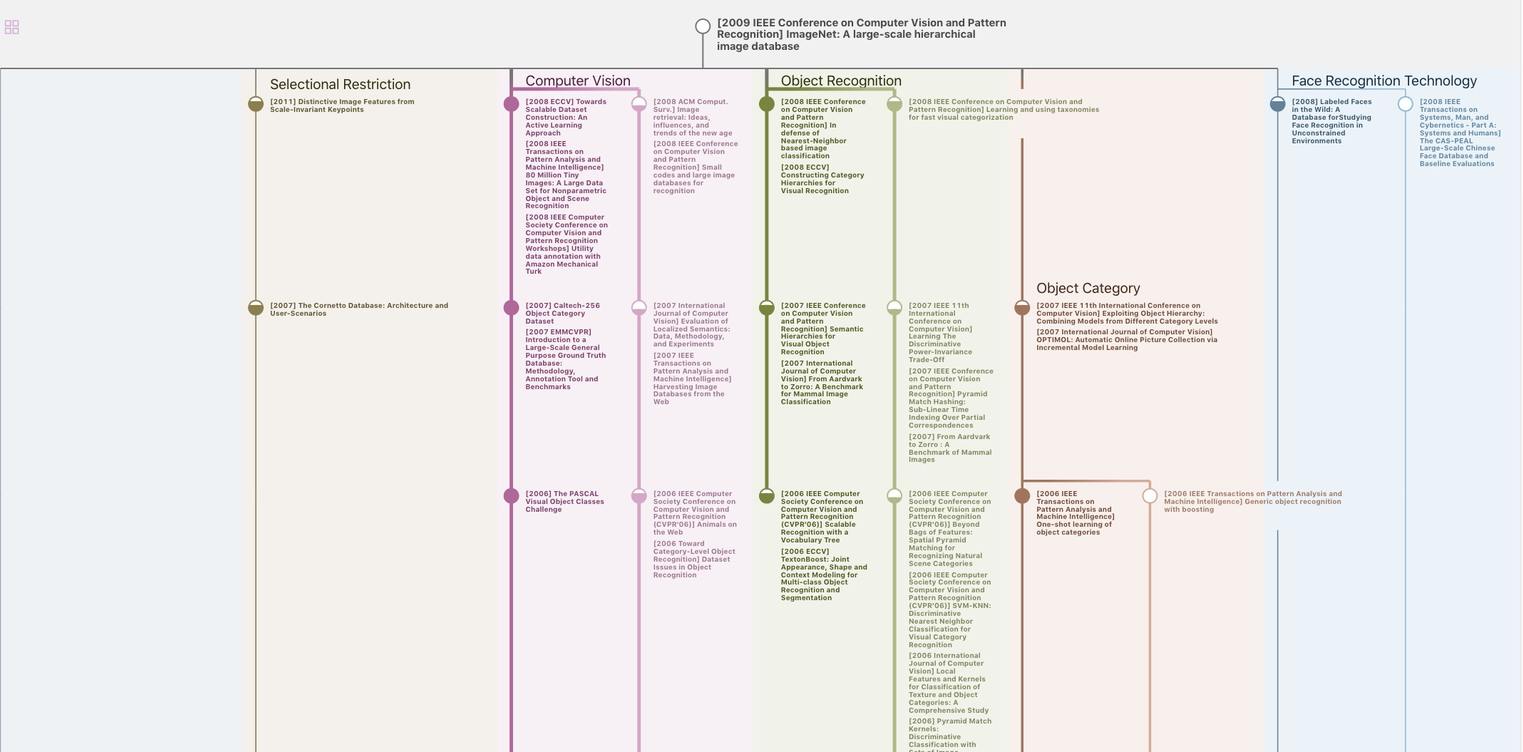
生成溯源树,研究论文发展脉络
Chat Paper
正在生成论文摘要