Morphology-based vs Unsupervised Word Clustering for Training Language Models for Serbian
ACTA POLYTECHNICA HUNGARICA(2019)
摘要
When training language models (especially for highly inflective languages), some applications require word clustering in order to mitigate the problem of insufficient training data or storage space. The goal of word clustering is to group words that can be well represented by a single class in the sense of probabilities of appearances in different contexts. This paper presents comparative results obtained by using different approaches to word clustering when training class N-gram models for Serbian, as well as models based on recurrent neural networks. One approach is unsupervised word clustering based on optimized Brown's algorithm, which relies on bigram statistics. The other approach is based on morphology, and it requires expert knowledge and language resources. Four different types of textual corpora were used in experiments, describing different functional styles. The language models were evaluated by both perplexity and word error rate. The results show notable advantage of introducing expert knowledge into word clustering process.
更多查看译文
关键词
N-gram,language model,word clustering,morphology,inflective languages
AI 理解论文
溯源树
样例
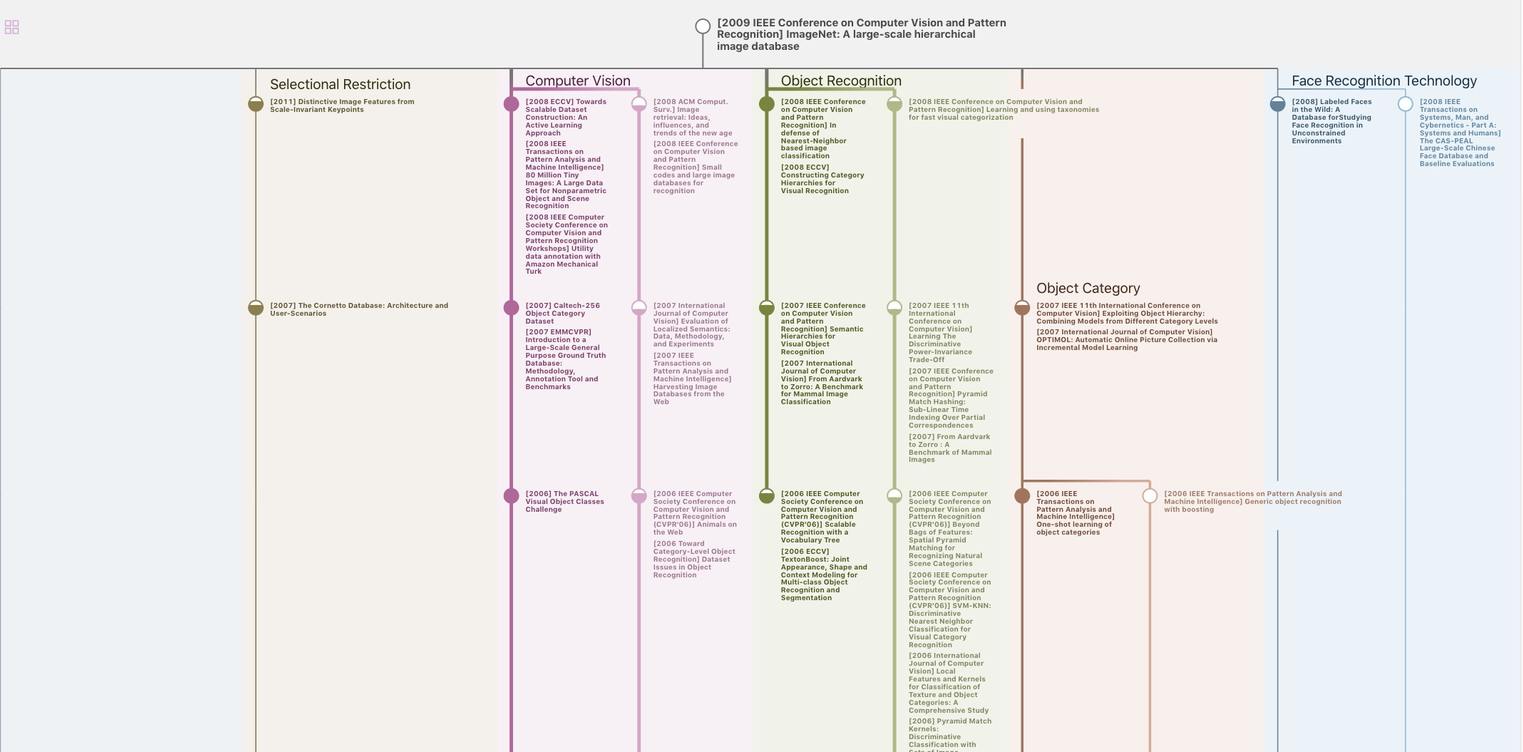
生成溯源树,研究论文发展脉络
Chat Paper
正在生成论文摘要