Reservoir Memory Machines as Neural Computers
IEEE Transactions on Neural Networks and Learning Systems(2022)
摘要
Differentiable neural computers (DNCs) extend artificial neural networks with an explicit memory without interference, thus enabling the model to perform classic computation tasks, such as graph traversal. However, such models are difficult to train, requiring long training times and large datasets. In this work, we achieve some of the computational capabilities of DNCs with a model that can be trained very efficiently, namely, an echo state network with an explicit memory without interference. This extension enables echo state networks to recognize all regular languages, including those that contractive echo state networks provably cannot recognize. Furthermore, we demonstrate experimentally that our model performs comparably to its fully trained deep version on several typical benchmark tasks for DNCs.
更多查看译文
关键词
Computers,Language,Memory,Neural Networks, Computer
AI 理解论文
溯源树
样例
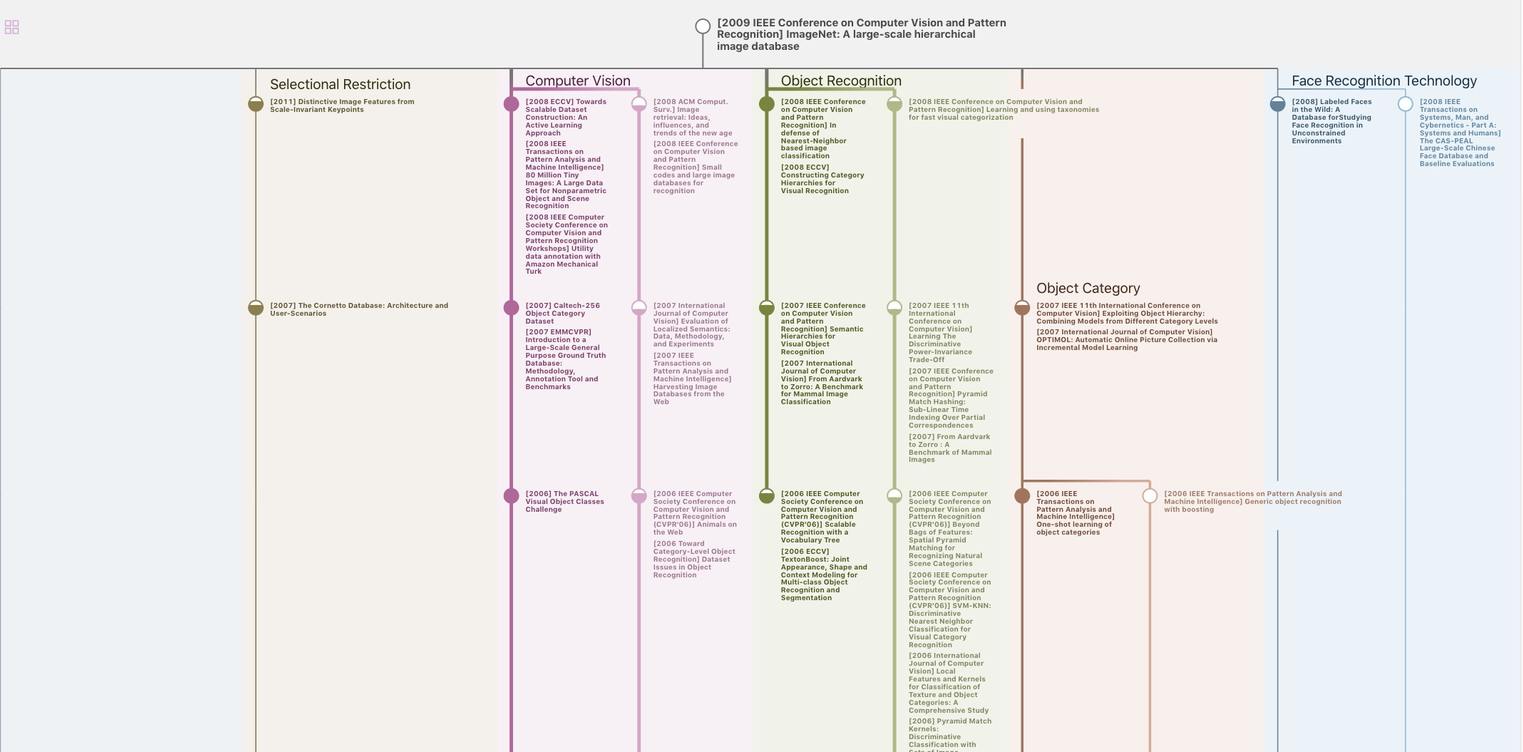
生成溯源树,研究论文发展脉络
Chat Paper
正在生成论文摘要