DAICS: A Deep Learning Solution for Anomaly Detection in Industrial Control Systems
IEEE Transactions on Emerging Topics in Computing(2022)
摘要
Deep Learning is emerging as an effective technique to detect sophisticated cyber-attacks targeting Industrial Control Systems (ICSs). The conventional detection approach is to learn the “normal” behaviour of the system, to be then able to label noteworthy deviations from it as anomalies. However, the normal behaviour of ICSs continuously evolves over time for multiple reasons, such as update/replacement of devices, workflow modifications or others. As a consequence, the accuracy of the anomaly detection process may be dramatically affected with a considerable amount of false alarms being generated. This article presents
DAICS
, a novel deep learning framework with a modular design to fit in large ICSs. The key component of the framework is a 2-branch neural network that learns the changes in the ICS behaviour with a small number of data samples and a few gradient updates. This is supported by an automatic tuning mechanism of the detection threshold that takes into account the changes in the prediction error under normal operating conditions. In this regard, no specialised human intervention is needed to update the other parameters of the system.
DAICS
has been evaluated using publicly available datasets and shows an increased detection rate and accuracy compared to state-of-the-art approaches, as well as higher robustness to additive noise.
更多查看译文
关键词
Anomaly detection,domain shift,deep learning,industrial control networks
AI 理解论文
溯源树
样例
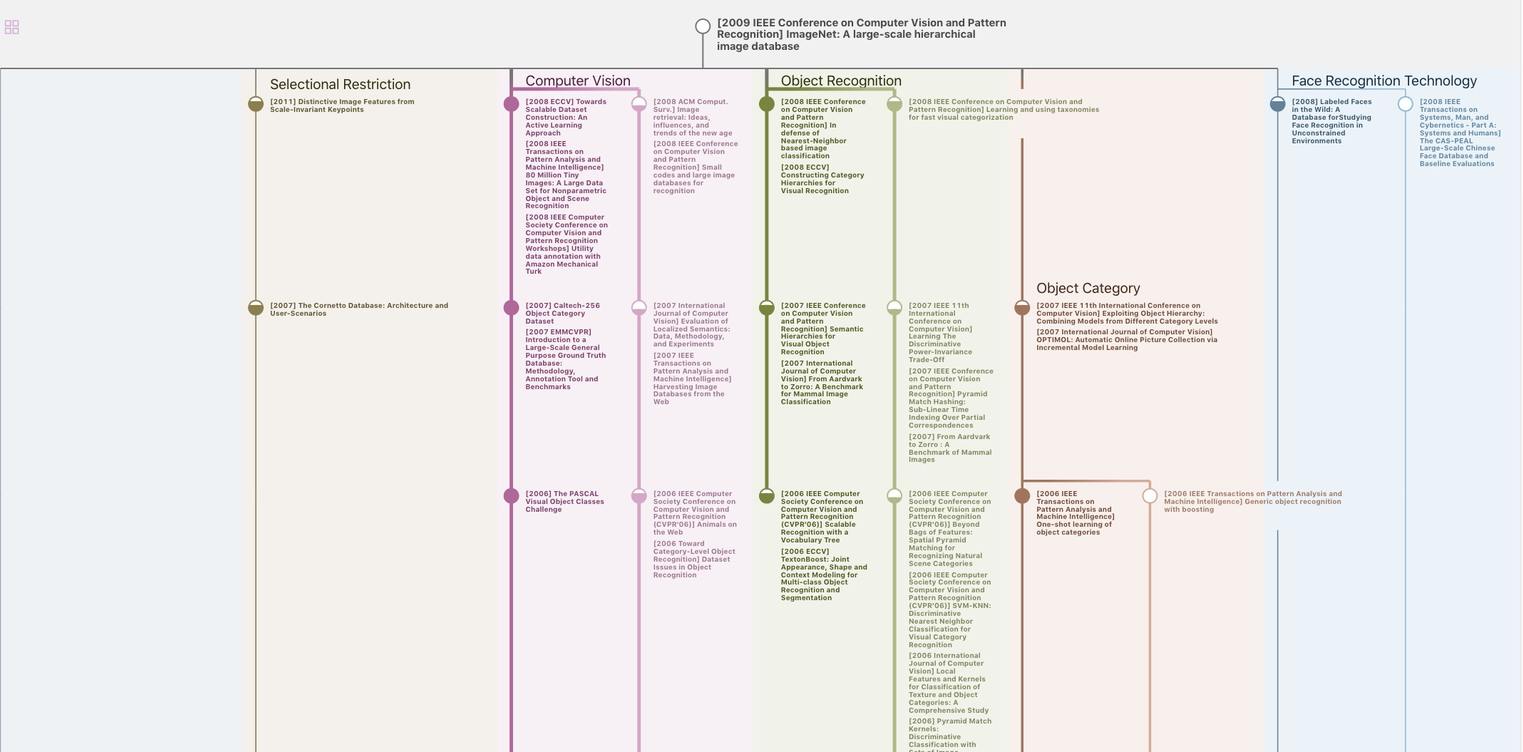
生成溯源树,研究论文发展脉络
Chat Paper
正在生成论文摘要