SAPAG: A Self-Adaptive Privacy Attack From Gradients
arxiv(2020)
摘要
Distributed learning such as federated learning or collaborative learning enables model training on decentralized data from users and only collects local gradients, where data is processed close to its sources for data privacy. The nature of not centralizing the training data addresses the privacy issue of privacy-sensitive data. Recent studies show that a third party can reconstruct the true training data in the distributed machine learning system through the publicly-shared gradients. However, existing reconstruction attack frameworks lack generalizability on different Deep Neural Network (DNN) architectures and different weight distribution initialization, and can only succeed in the early training phase. To address these limitations, in this paper, we propose a more general privacy attack from gradient, SAPAG, which uses a Gaussian kernel based of gradient difference as a distance measure. Our experiments demonstrate that SAPAG can construct the training data on different DNNs with different weight initializations and on DNNs in any training phases.
更多查看译文
关键词
privacy,self-adaptive
AI 理解论文
溯源树
样例
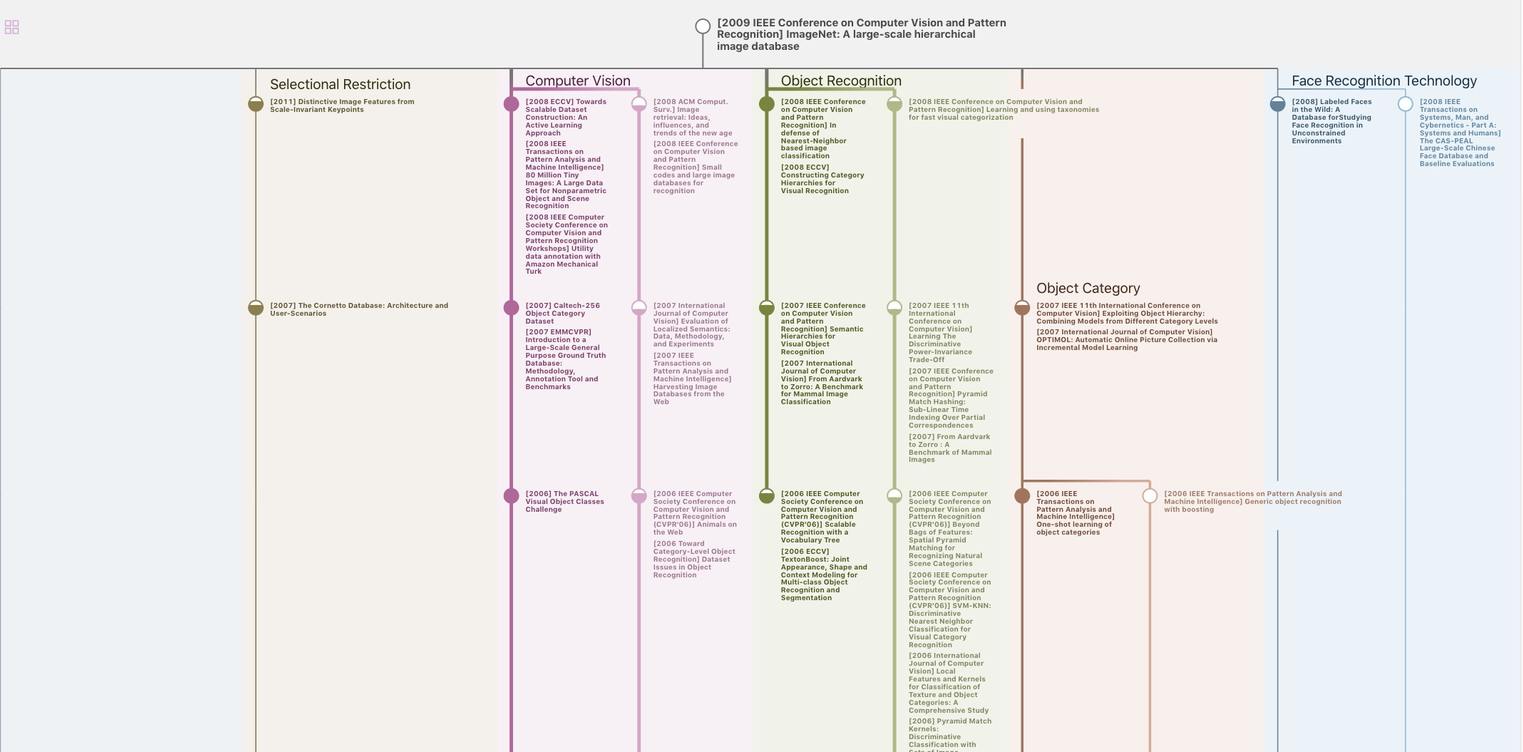
生成溯源树,研究论文发展脉络
Chat Paper
正在生成论文摘要