GINet: Graph Interaction Network for Scene Parsing
European Conference on Computer Vision(2020)
摘要
Recently, context reasoning using image regions beyond local convolution has shown great potential for scene parsing. In this work, we explore how to incorperate the linguistic knowledge to promote context reasoning over image regions by proposing a Graph Interaction unit (GI unit) and a Semantic Context Loss (SC-loss). The GI unit is capable of enhancing feature representations of convolution networks over high-level semantics and learning the semantic coherency adaptively to each sample. Specifically, the dataset-based linguistic knowledge is first incorporated in the GI unit to promote context reasoning over the visual graph, then the evolved representations of the visual graph are mapped to each local representation to enhance the discriminated capability for scene parsing. GI unit is further improved by the SC-loss to enhance the semantic representations over the exemplar-based semantic graph. We perform full ablation studies to demonstrate the effectiveness of each component in our approach. Particularly, the proposed GINet outperforms the state-of-the-art approaches on the popular benchmarks, including Pascal-Context and COCO Stuff.
更多查看译文
关键词
Scene parsing, Context reasoning, Graph interaction
AI 理解论文
溯源树
样例
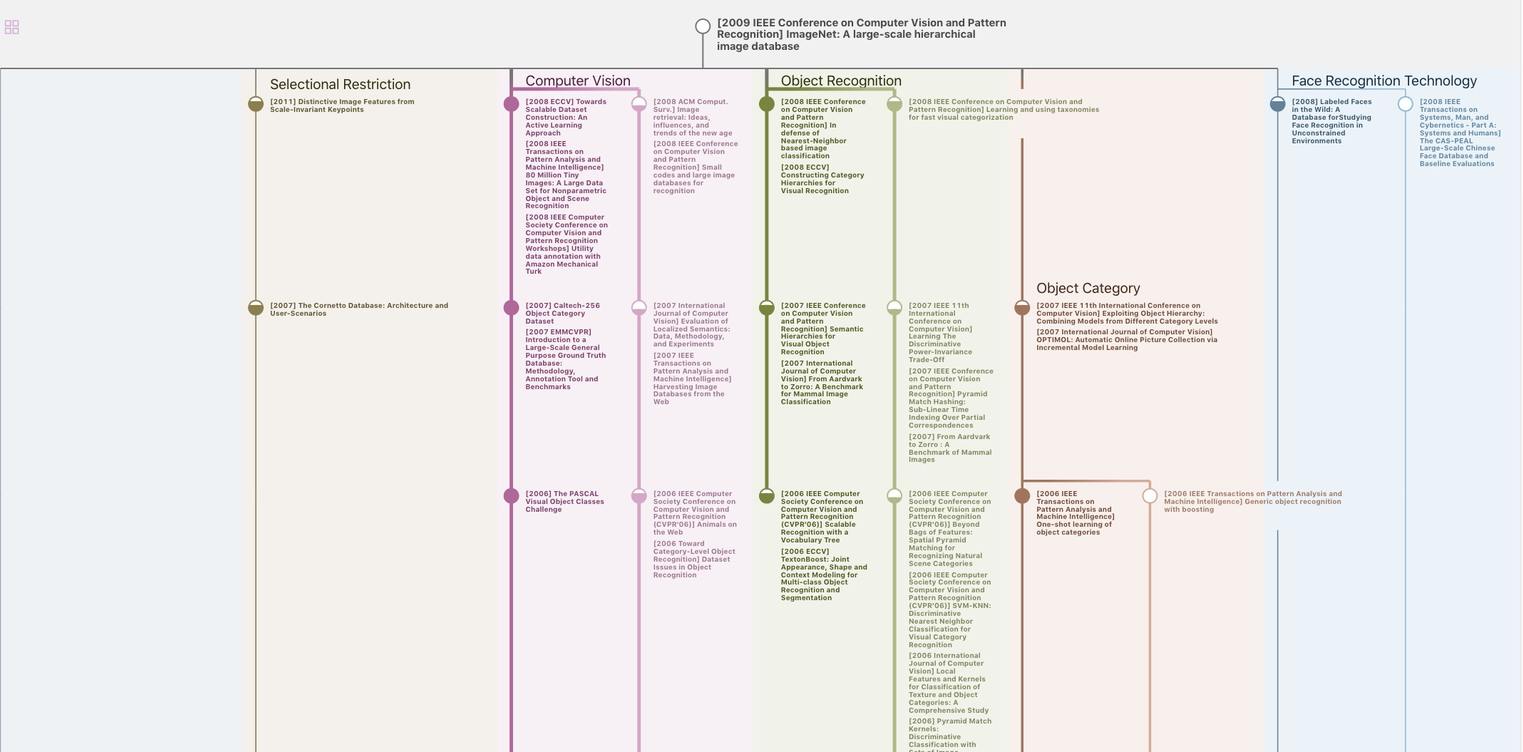
生成溯源树,研究论文发展脉络
Chat Paper
正在生成论文摘要