Spoiled for Choice? Personalized Recommendation for Healthcare Decisions: A Multiarmed Bandit Approach
arxiv(2023)
摘要
Online healthcare platforms provide users with various intervention programs to promote personal wellness. Given the many options available, it's often difficult for individuals to decide in which intervention to participate, especially when they lack the experience or knowledge to evaluate the interventions. This may discourage individuals' continuous engagement in online health management. In this study, we are motivated to develop a personalized healthcare recommendation framework to help individuals better discover the interventions that fit their needs. Considering the challenges in intervention adaptation and diversification in a highly dynamic online healthcare environment, we propose an innovative online learning framework that synthesizes deep representation learning and a theoryguided diversity promotion scheme. We evaluate our approach through a real-world data set on users' intervention participation in an online weight-loss community. Our results provide strong evidence for the effectiveness of our proposed recommendation framework and each of its design components. Our study contributes to the emerging information systems research on prescriptive analytics and the application of business intelligence. The proposed modeling framework and evaluation results offer important implications for multiple stakeholders, including online healthcare platforms, policymakers, and users.
更多查看译文
关键词
personal health management,online healthcare interventions,recommendation systems,health behavior dynamics,diversity,deep representation learning,multiarmed bandit,prescriptive analytics
AI 理解论文
溯源树
样例
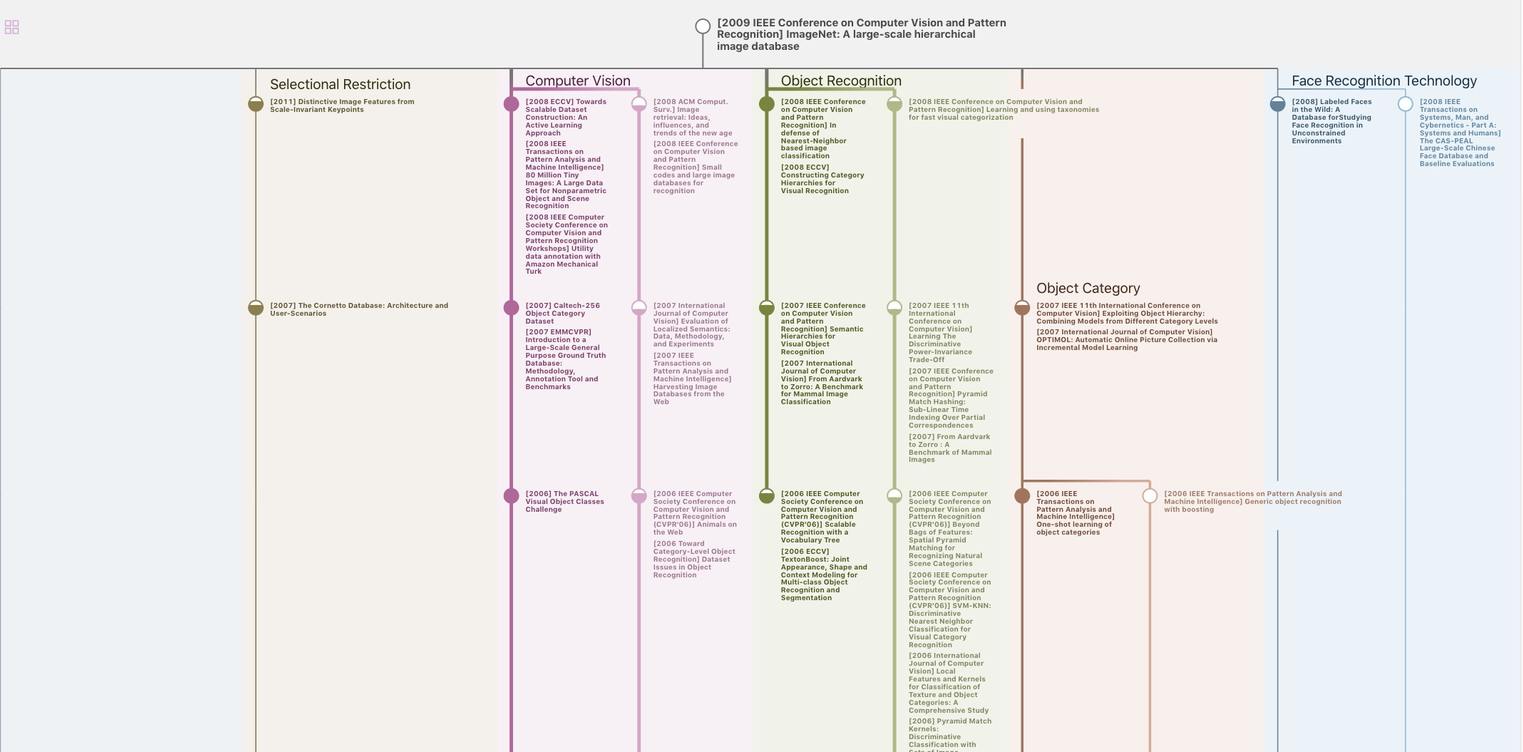
生成溯源树,研究论文发展脉络
Chat Paper
正在生成论文摘要