Efficient Sample based Neural Architecture Search with Learnable Predictor
arXiv(2019)
摘要
Neural Architecture Search (NAS) has shown great potentials in finding a better neural network design than human design. Sample-based NAS is the most fundamental method aiming at exploring the search space and evaluating the most promising architecture. However, few works have focused on improving the sampling efficiency for NAS algorithm. For balancing exploitation and exploration, we propose BONAS (Bayesian Optimized Neural Architecture Search), a sample-based NAS framework combined with Bayesian Optimization. The main components of BONAS are Sampler and Learnable Embedding Extractor. Specifically, we apply Evolution Algorithm method as our sampler and apply Graph Convolutional Network predictor as a surrogate model to adaptively discover and incorporate nodes structure to approximate the performance of the architecture. For NAS-oriented tasks, we also design a weighted …
更多查看译文
AI 理解论文
溯源树
样例
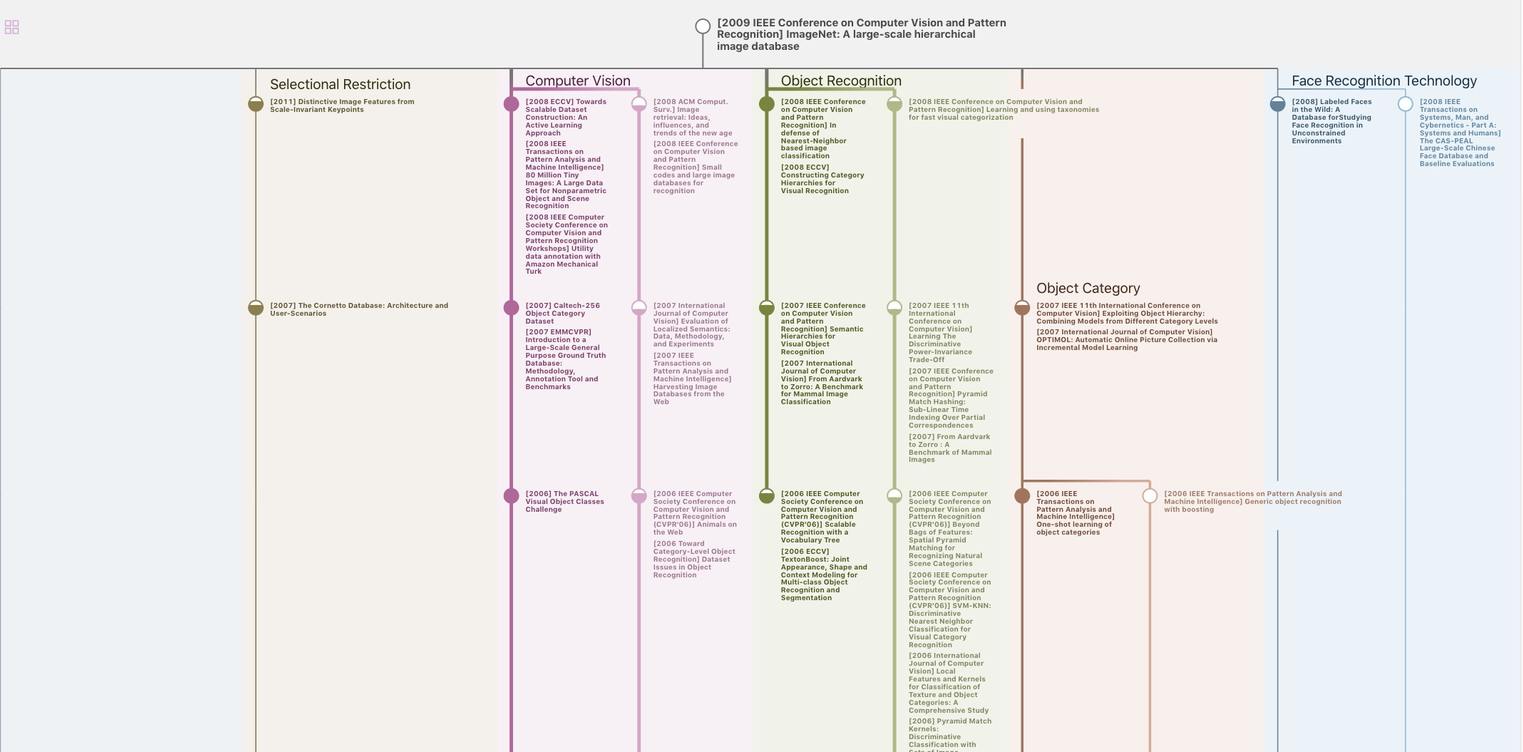
生成溯源树,研究论文发展脉络
Chat Paper
正在生成论文摘要