Mechanisms for a No-Regret Agent: Beyond the Common Prior
2020 IEEE 61st Annual Symposium on Foundations of Computer Science (FOCS)(2020)
摘要
A rich class of mechanism design problems can be understood as incomplete-information games between a principal who commits to a policy and an agent who responds, with payoffs determined by an unknown state of the world. Traditionally, these models require strong and often-impractical assumptions about beliefs (a common prior over the state). In this paper, we dispense with the common prior. Instead, we consider a repeated interaction where both the principal and the agent may learn over time from the state history. We reformulate mechanism design as a reinforcement learning problem and develop mechanisms that attain natural benchmarks without any assumptions on the state-generating process. Our results make use of novel behavioral assumptions for the agent - based on counterfactual internal regret - that capture the spirit of rationality without relying on beliefs.
11
For the full version of this paper, see https://arxiv.org/abs/2009.05518.
更多查看译文
关键词
n/a
AI 理解论文
溯源树
样例
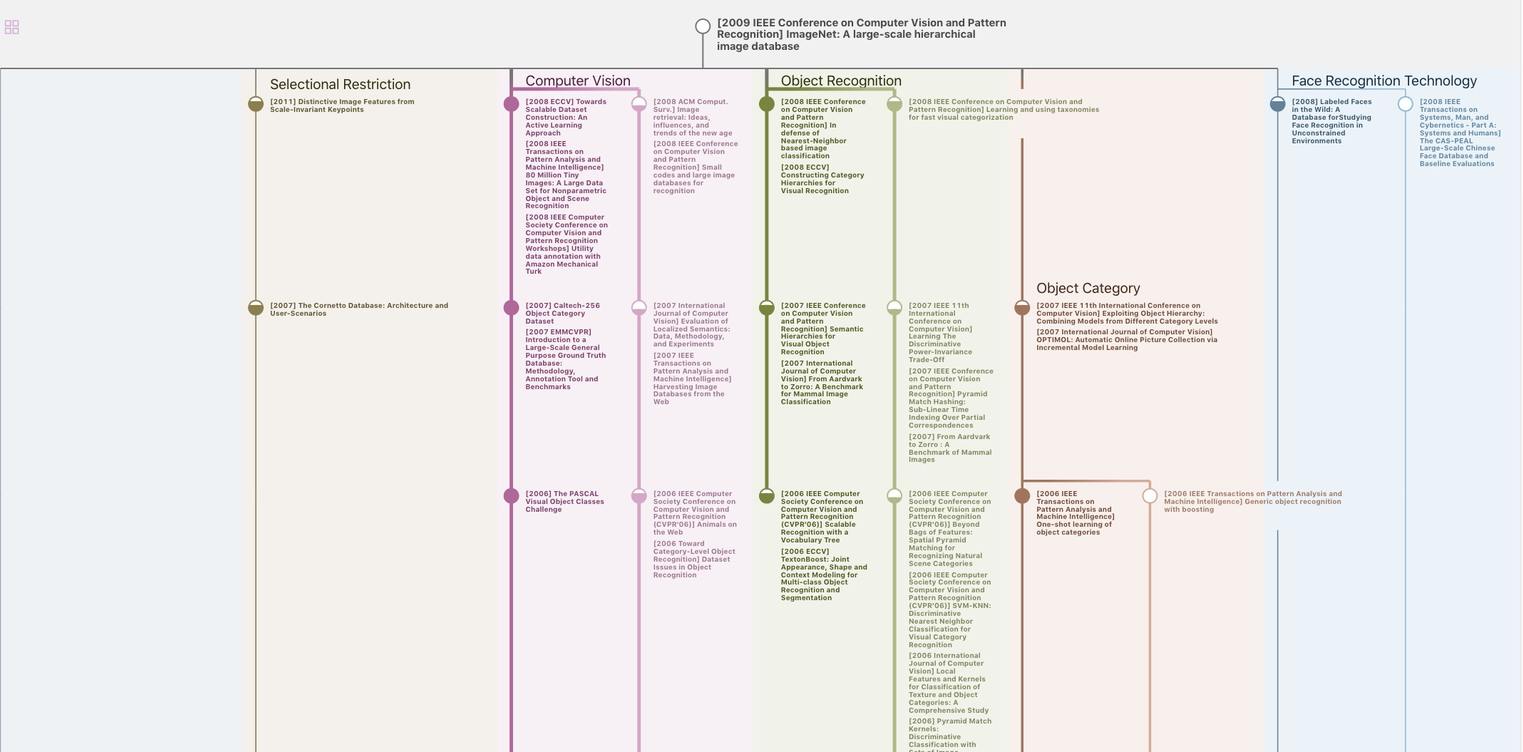
生成溯源树,研究论文发展脉络
Chat Paper
正在生成论文摘要