Towards Interpretable Multi-task Learning Using Bilevel Programming
MACHINE LEARNING AND KNOWLEDGE DISCOVERY IN DATABASES, ECML PKDD 2020, PT II(2021)
摘要
Interpretable Multi-Task Learning can be expressed as learning a sparse graph of the task relationship based on the prediction performance of the learned models. Since many natural phenomenon exhibit sparse structures, enforcing sparsity on learned models reveals the underlying task relationship. Moreover, different sparsification degrees from a fully connected graph uncover various types of structures, like cliques, trees, lines, clusters or fully disconnected graphs. In this paper, we propose a bilevel formulation of multi-task learning that induces sparse graphs, thus, revealing the underlying task relationships, and an efficient method for its computation. We show empirically how the induced sparse graph improves the interpretability of the learned models and their relationship on synthetic and real data, without sacrificing generalization performance. Code at https://bit.ly/GraphGuidedMTL.
更多查看译文
关键词
Interpretable machine learning, Multi-task learning, Structure learning, Sparse graph, Transfer learning
AI 理解论文
溯源树
样例
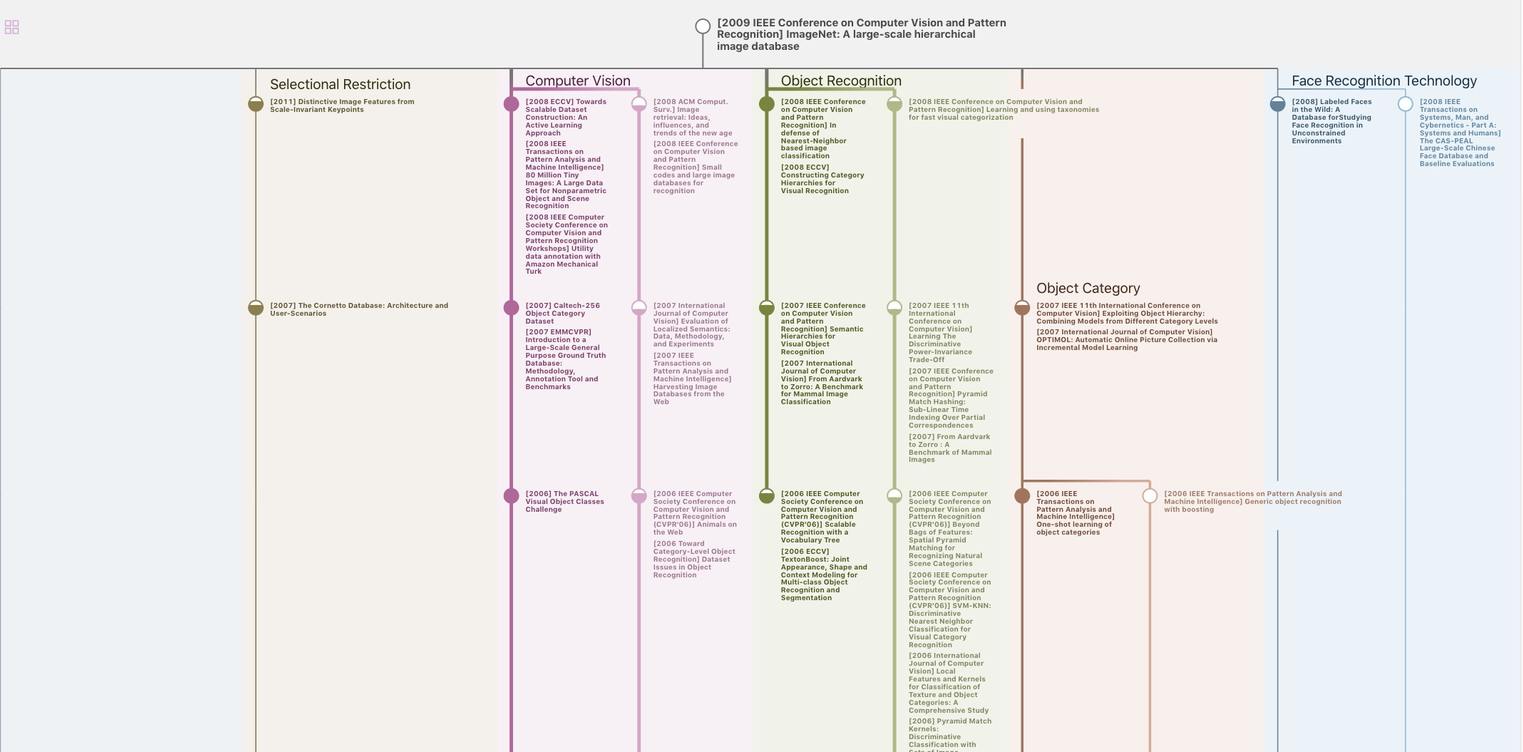
生成溯源树,研究论文发展脉络
Chat Paper
正在生成论文摘要