On the estimation of spatial density from mobile network operator data
arxiv(2020)
摘要
Mobile Network Operator (MNO) data are increasingly used to infer mobility and presence patterns of human population. Whether based on Cell Detail Records (CDR) or signalling events, MNO data can be leveraged to estimate the spatial distribution of mobile devices at a given time, and from there extrapolate the distribution of humans. The process of transforming MNO data to a density map involves a chain of multiple processing blocks. A key block relates to \emph{geo-location} of individual radio cells or groups thereof, i.e., to methods for determining the spatial footprint of radio cells. Traditionally, researchers have resorted to geo-location methods based on Voronoi tessellations or variants thereof: with this approach, cell locations are mutually disjoint and the density estimation task reduces to a simple area-proportional computation. More recently, some pioneering work have started to consider more elaborated geo-location methods with partially overlapping (non-disjont) cell footprints. Estimating the spatial density from a set of overlapping cell locations is currently an open research problem, and it is the focus of this work. In this contribution we start by reviewing three different estimation methods proposed in the literature, for which we provide novel analytic results, based on formal proofs, unveiling some key aspects of their mutual relationships. Furthermore, we develop a novel estimator for which a closed-form solution can be given. Numerical results based on synthetic data are presented to assess the relative accuracy of each method.
更多查看译文
关键词
Mobile network data,call detail records,spatial density estimation,present population
AI 理解论文
溯源树
样例
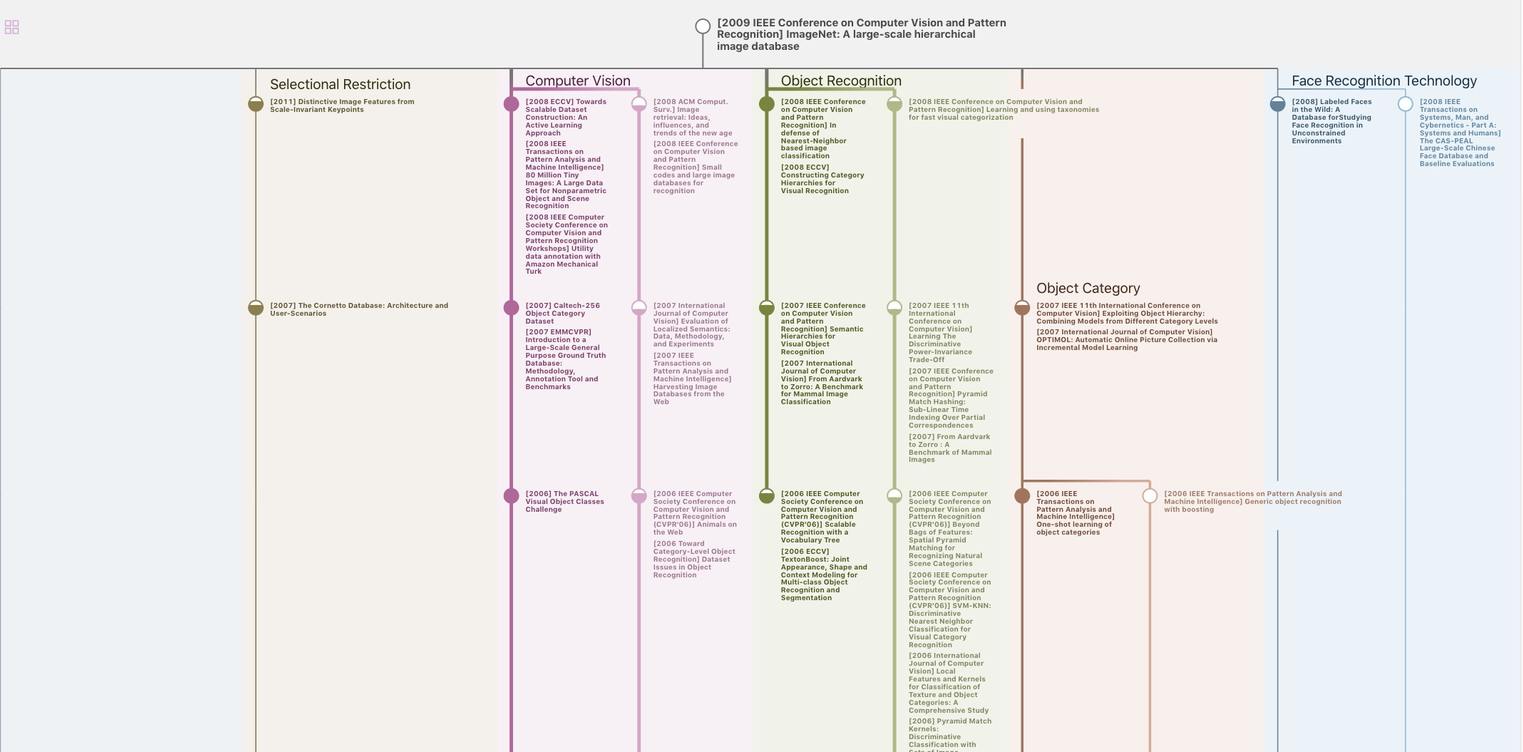
生成溯源树,研究论文发展脉络
Chat Paper
正在生成论文摘要