Comparison of Mortality Predictive Models of Sepsis Patients Based on Machine Learning.
Chinese medical sciences journal = Chung-kuo i hsueh k'o hsueh tsa chih(2022)
摘要
Objective To compare the performance of five machine learning models and SAPS II score in predicting the 30-day mortality amongst patients with sepsis. Methods The sepsis patient-related data were extracted from the MIMIC-IV database. Clinical features were generated and selected by mutual information and grid search. Logistic regression, Random forest, LightGBM, XGBoost, and other machine learning models were constructed to predict the mortality probability. Five measurements including accuracy, precision, recall, F1 score, and area under curve (AUC) were acquired for model evaluation. An external validation was implemented to avoid conclusion bias. Results LightGBM outperformed other methods, achieving the highest AUC (0.900), accuracy (0.808), and precision (0.559). All machine learning models performed better than SAPS II score (AUC=0.748). LightGBM achieved 0.883 in AUC in the external data validation. Conclusions The machine learning models are more effective in predicting the 30-day mortality of patients with sepsis than the traditional SAPS II score.
更多查看译文
关键词
MIMIC-IV,machine learning,risk prediction,sepsis
AI 理解论文
溯源树
样例
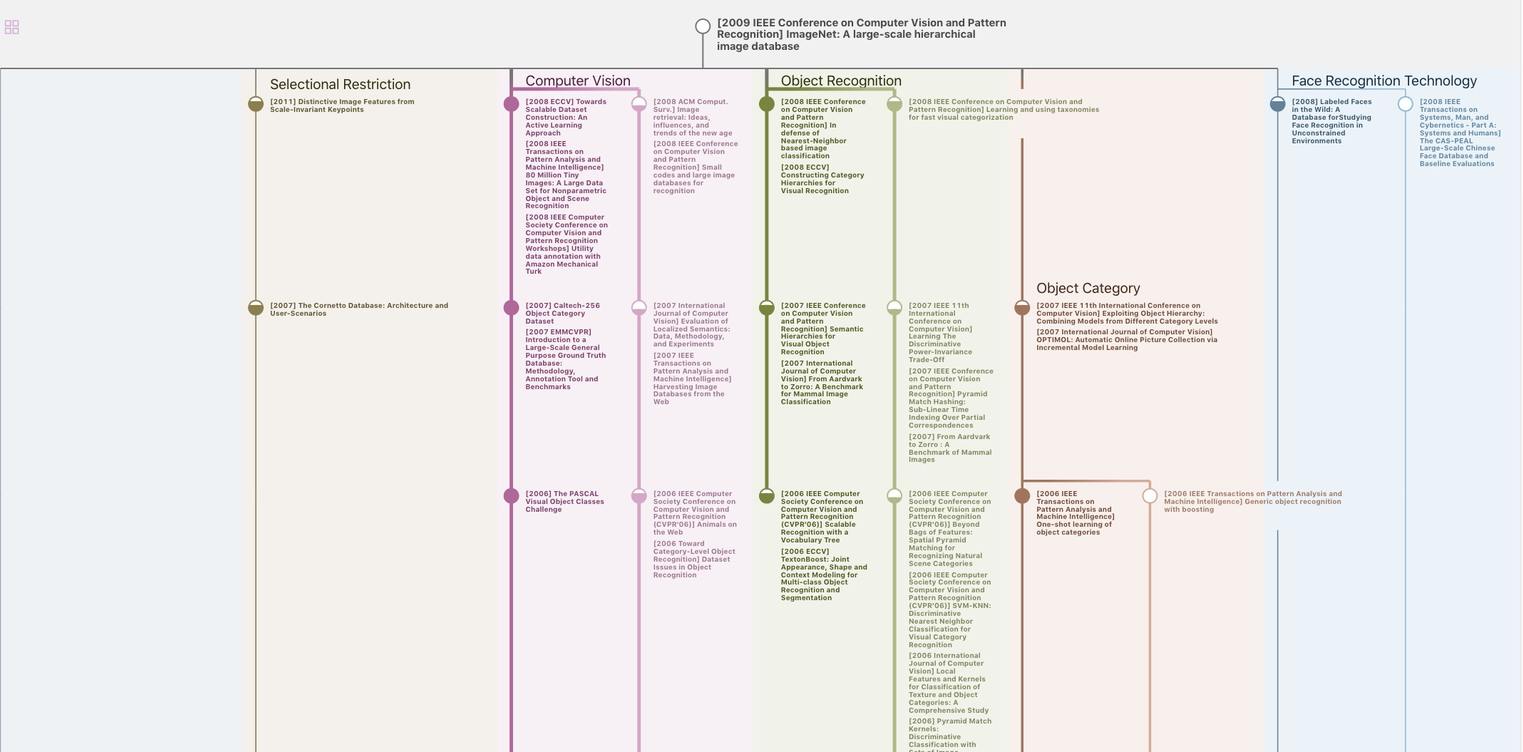
生成溯源树,研究论文发展脉络
Chat Paper
正在生成论文摘要