Active Surveillance via Group Sparse Bayesian Learning
IEEE Transactions on Pattern Analysis and Machine Intelligence(2022)
摘要
The key to the effective control of a diffusion system lies in how accurately we could predict its unfolding dynamics based on the observation of its current state. However, in the real-world applications, it is often infeasible to conduct a timely and yet comprehensive observation due to resource constraints. In view of such a practical challenge, the goal of this work is to develop a novel computational method for performing active observations, termed active surveillance, with limited resources. Specifically, we aim to predict the dynamics of a large spatio-temporal diffusion system based on the observations of some of its components. Towards this end, we introduce a novel measure, the
$\boldsymbol{\gamma }$
value, that enables us to identify the key components by means of modeling a sentinel network with a row sparsity structure. Having obtained a theoretical understanding of the
$\boldsymbol{\gamma }$
value, we design a backward-selection sentinel network mining algorithm (SNMA) for deriving the sentinel network via
group sparse Bayesian learning
. In order to be practically useful, we further address the issue of scalability in the computation of SNMA, and moreover, extend SNMA to the case of a non-linear dynamical system that could involve complex diffusion mechanisms. We show the effectiveness of SNMA by validating it using both synthetic datasets and five real-world datasets. The experimental results are appealing, which demonstrate that SNMA readily outperforms the state-of-the-art methods.
更多查看译文
关键词
Epidemic dynamics,diffusion,sensor deployment,dynamical systems,automatic relevance determination
AI 理解论文
溯源树
样例
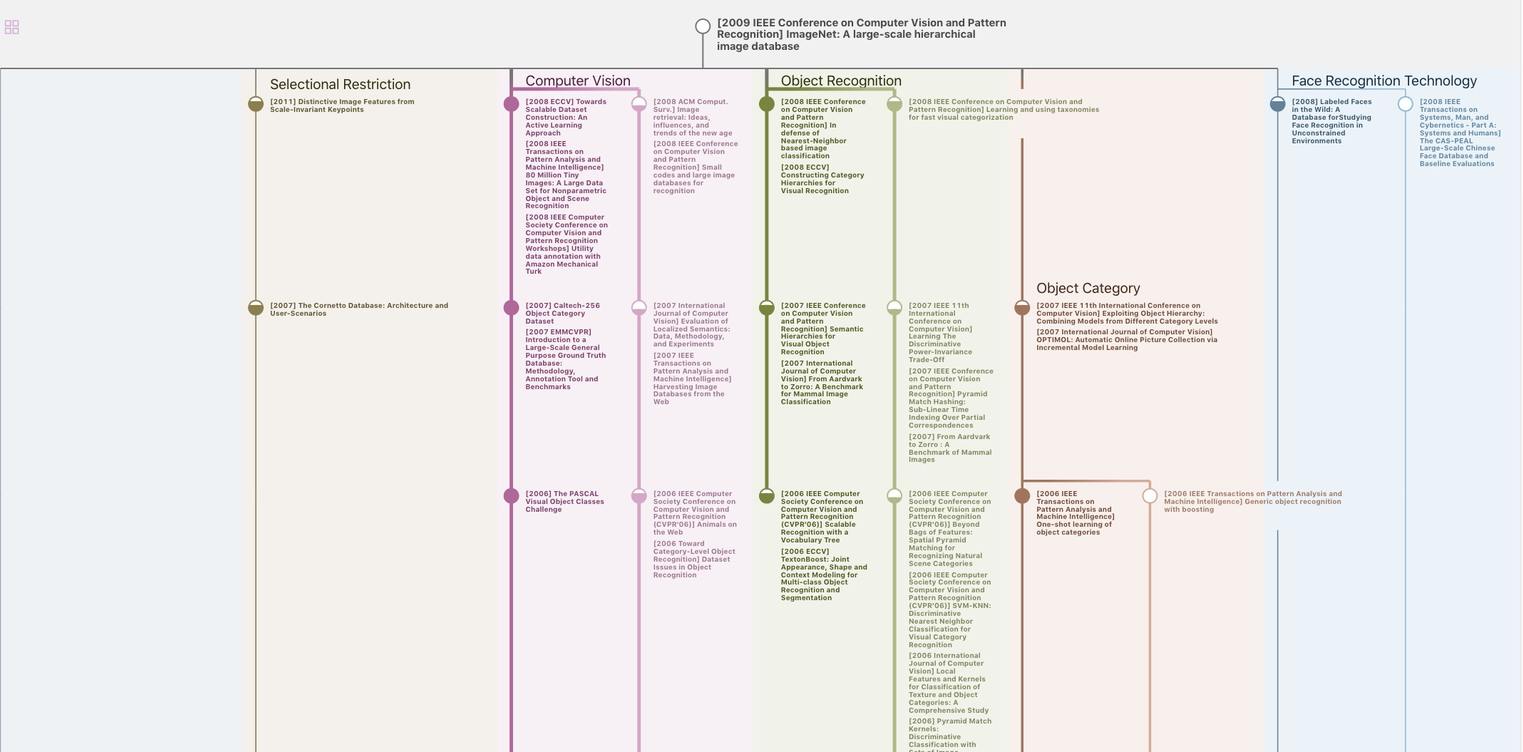
生成溯源树,研究论文发展脉络
Chat Paper
正在生成论文摘要