Proposal-Free Volumetric Instance Segmentation from Latent Single-Instance Masks
GCPR(2020)
摘要
This work introduces a new proposal-free instance segmentation method that builds on single-instance segmentation masks predicted across the entire image in a sliding window style. In contrast to related approaches, our method concurrently predicts all masks, one for each pixel, and thus resolves any conflict jointly across the entire image. Specifically, predictions from overlapping masks are combined into edge weights of a signed graph that is subsequently partitioned to obtain all final instances concurrently. The result is a parameter-free method that is strongly robust to noise and prioritizes predictions with the highest consensus across overlapping masks. All masks are decoded from a low dimensional latent representation, which results in great memory savings strictly required for applications to large volumetric images. We test our method on the challenging CREMI 2016 neuron segmentation benchmark where it achieves competitive scores.
更多查看译文
关键词
proposal-free,single-instance
AI 理解论文
溯源树
样例
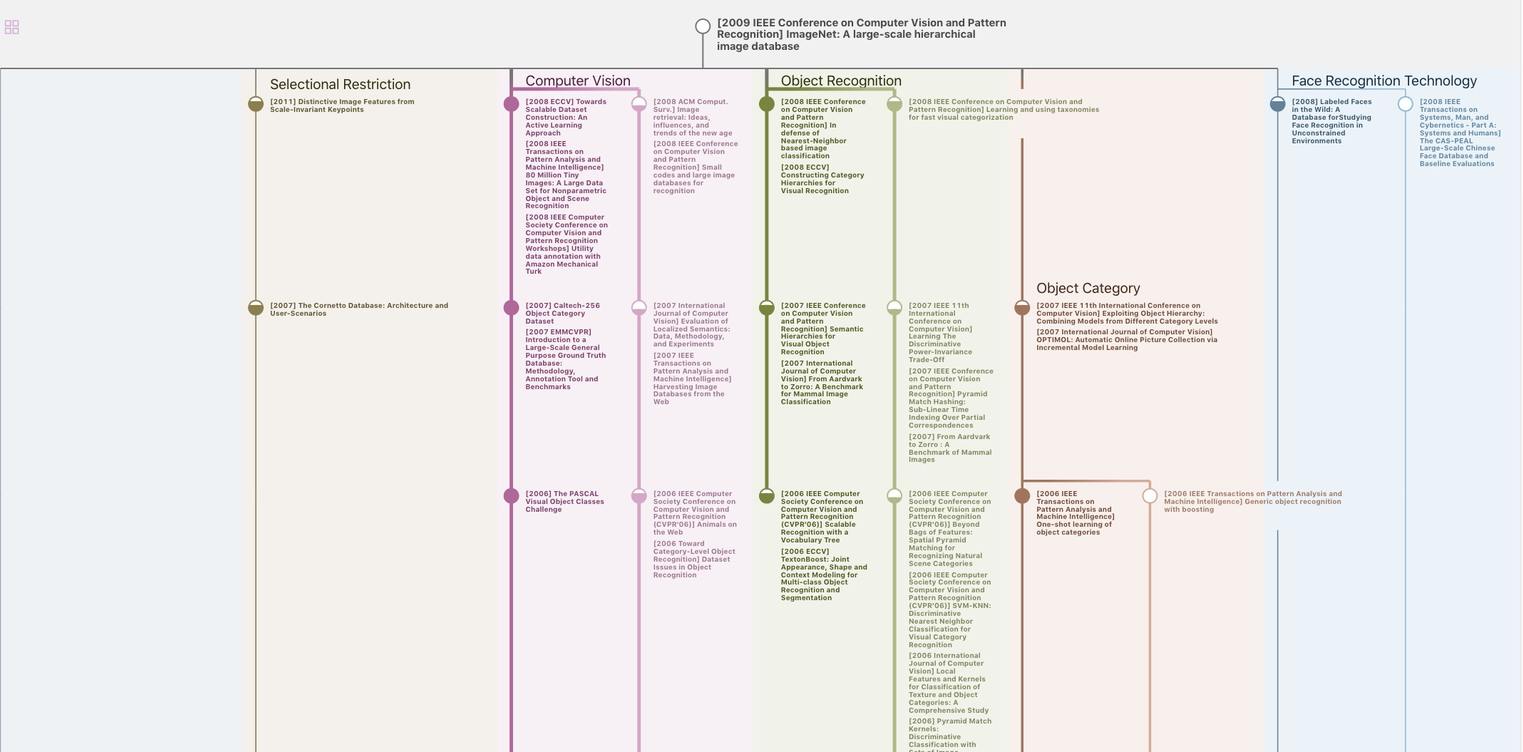
生成溯源树,研究论文发展脉络
Chat Paper
正在生成论文摘要