Population structure-learned classifier for high-dimension low-sample-size class-imbalanced problem
Engineering Applications of Artificial Intelligence(2022)
摘要
The classification on high-dimension low-sample-size data (HDLSS) is a challenging problem and it is common to have class-imbalanced data in most application fields. We term this as Imbalanced HDLSS (IHDLSS). Recent theoretical results reveal that the classification criterion and tolerance similarity are crucial to HDLSS, which emphasizes the maximization of within-class variance on the premise of class separability. Based on this idea, a novel linear binary classifier, termed Population Structure-learned Classifier (PSC), is proposed. The proposed PSC can obtain better generalization performance on IHDLSS by maximizing the sum of inter-class scatter matrix and intra-class scatter matrix on the premise of class separability and assigning different intercept values to majority and minority classes. The salient features of the proposed approach are: (1) It works well on IHDLSS; (2) The inverse of high dimensional matrix can be solved in low dimensional space; (3) It is self-adaptive in determining the intercept term for each class; (4) Its computational complexity is analyzed. A series of evaluations are conducted on one simulated data set and ten real-world benchmark data sets on IHDLSS on gene analysis. Experimental results demonstrate that the PSC is superior to the state-of-art methods in IHDLSS.
更多查看译文
关键词
High-dimension low-sample-size,Binary linear classifier,Data piling,Class-imbalanced data,Population structure-learned
AI 理解论文
溯源树
样例
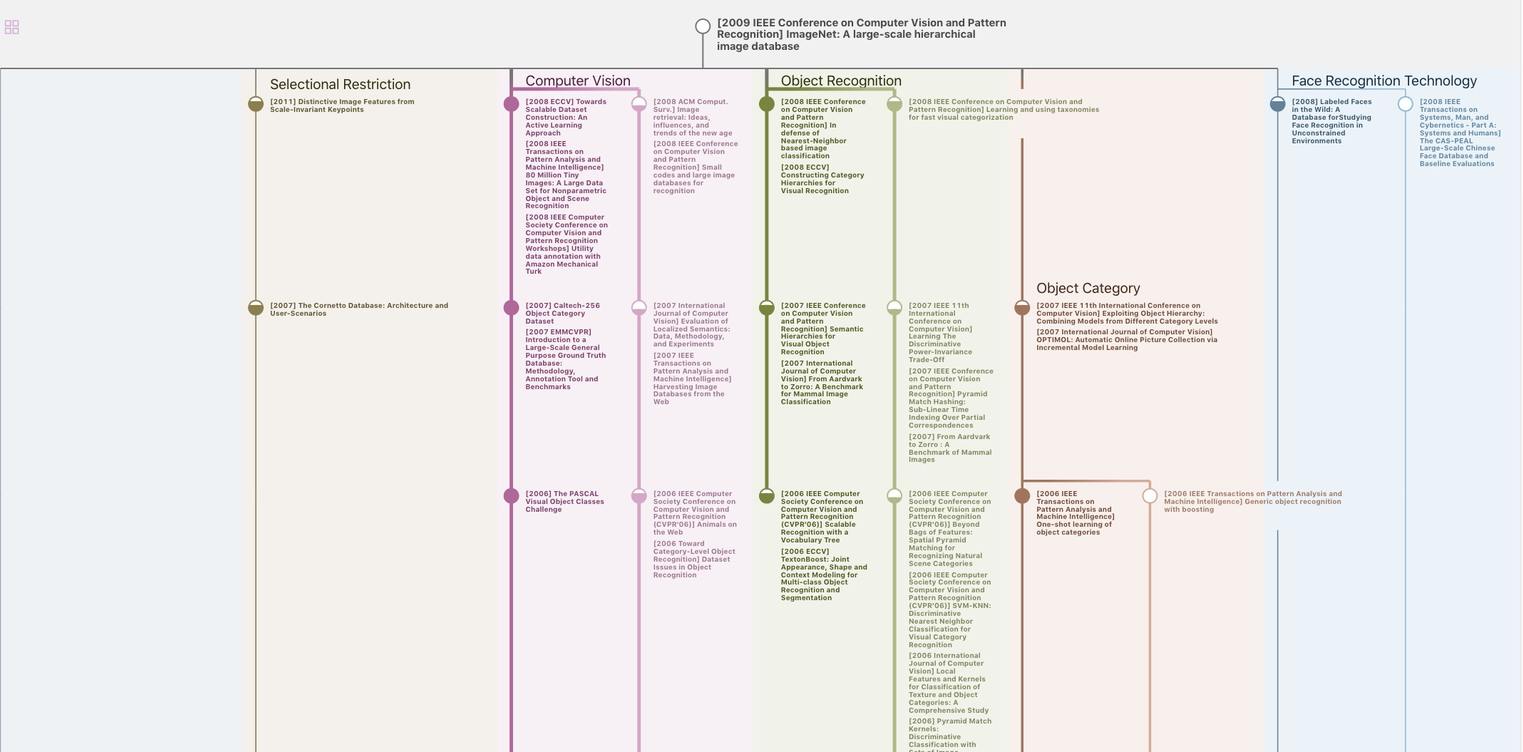
生成溯源树,研究论文发展脉络
Chat Paper
正在生成论文摘要