Physics-Informed Neural Networks for Nonhomogeneous Material Identification in Elasticity Imaging
arxiv(2020)
摘要
We apply Physics-Informed Neural Networks (PINNs) for solving identification problems of nonhomogeneous materials. We focus on the problem with a background in elasticity imaging, where one seeks to identify the nonhomogeneous mechanical properties of soft tissue based on the full-field displacement measurements under quasi-static loading. In our model, we apply two independent neural networks, one for approximating the solution of the corresponding forward problem, and the other for approximating the unknown material parameter field. As a proof of concept, we validate our model on a prototypical plane strain problem for incompressible hyperelastic tissue. The results show that the PINNs are effective in accurately recovering the unknown distribution of mechanical properties. By employing two neural networks in our model, we extend the capability of material identification of PINNs to include nonhomogeneous material parameter fields, which enables more flexibility of PINNs in representing complex material properties.
更多查看译文
关键词
nonhomogeneous material
AI 理解论文
溯源树
样例
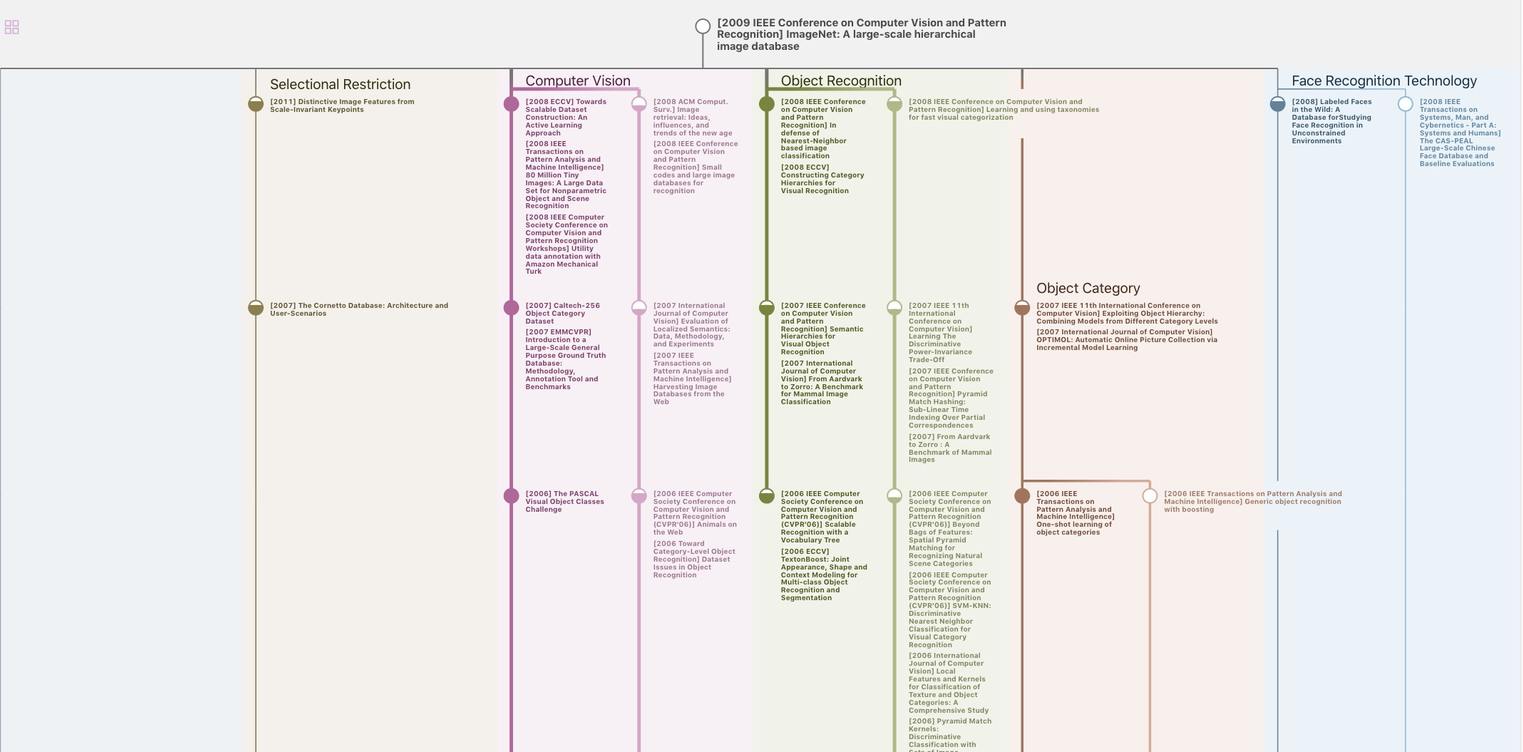
生成溯源树,研究论文发展脉络
Chat Paper
正在生成论文摘要