Map-Adaptive Goal-Based Trajectory Prediction.
CoRL(2020)
摘要
We present a new method for multi-modal, long-term vehicle trajectory prediction. Our approach relies on using lane centerlines captured in rich maps of the environment to generate a set of proposed goal paths for each vehicle. Using these paths -- which are generated at run time and therefore dynamically adapt to the scene -- as spatial anchors, we predict a set of goal-based trajectories along with a categorical distribution over the goals. This approach allows us to directly model the goal-directed behavior of traffic actors, which unlocks the potential for more accurate long-term prediction. Our experimental results on both a large-scale internal driving dataset and on the public nuScenes dataset show that our model outperforms state-of-the-art approaches for vehicle trajectory prediction over a 6-second horizon. We also empirically demonstrate that our model is better able to generalize to road scenes from a completely new city than existing methods.
更多查看译文
关键词
trajectory prediction,map-adaptive,goal-based
AI 理解论文
溯源树
样例
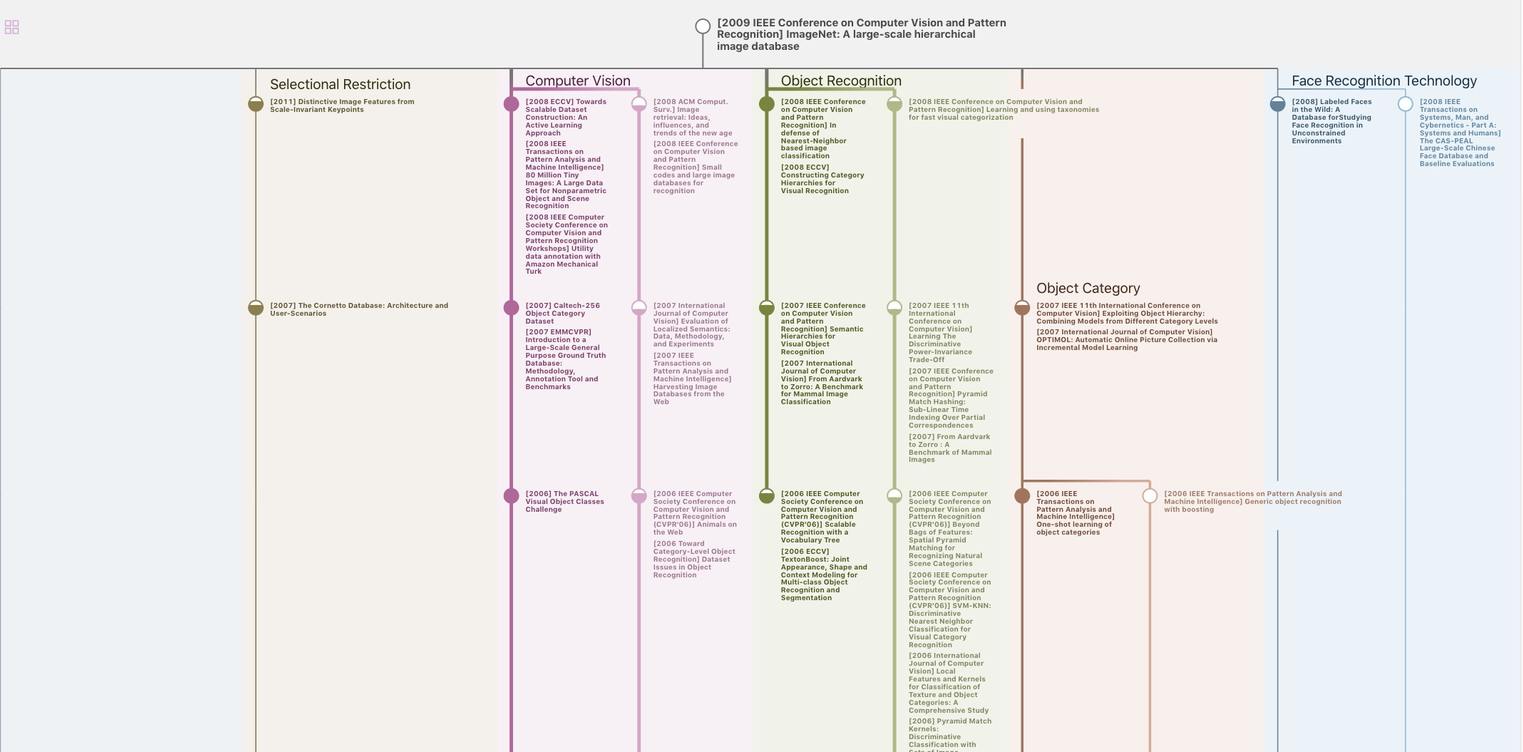
生成溯源树,研究论文发展脉络
Chat Paper
正在生成论文摘要