Data-Assisted Combustion Simulations With Dynamic Submodel Assignment Using Random Forests
COMBUSTION AND FLAME(2021)
摘要
This investigation outlines a data-assisted approach that employs random forest classifiers for local and dynamic submodel assignment in turbulent-combustion simulations. This method is demonstrated in simulations of a single-element GOX/GCH4 rocket combustor; a priori as well as a posteriori assessments are conducted to (i) evaluate the accuracy and adjustability of the classifier for targeting different quantities of interest (QoIs), and (ii) assess improvements, resulting from the data-assisted combustion model assignment, in predicting target QoIs during simulation runtime. Results from the a priori study show that random forests, trained with local flow properties as input variables and combustion model errors as training labels, assign three different combustion models - finite-rate chemistry (FRC), flamelet progress variable (FPV) model, and inert mixing (IM) - with reasonable classification performance even when targeting multiple QoIs. Applications in a posteriori studies demonstrate improved predictions from data-assisted simulations, in temperature and CO mass fraction, when compared with monolithic FPV calculations. An additional a posteriori data-assisted simulation of a modified configuration demonstrates that the present approach can be successfully applied to different configurations, as long as thermophysical behavior can be represented by the training data. These results demonstrate that this data-driven framework holds promise for dynamic combustion submodel assignments in reacting flow simulations.(c) 2021 The Combustion Institute. Published by Elsevier Inc. All rights reserved.
更多查看译文
关键词
Combustion modeling, Model assignment, Random forests, Machine learning, Classification, Turbulent combustion
AI 理解论文
溯源树
样例
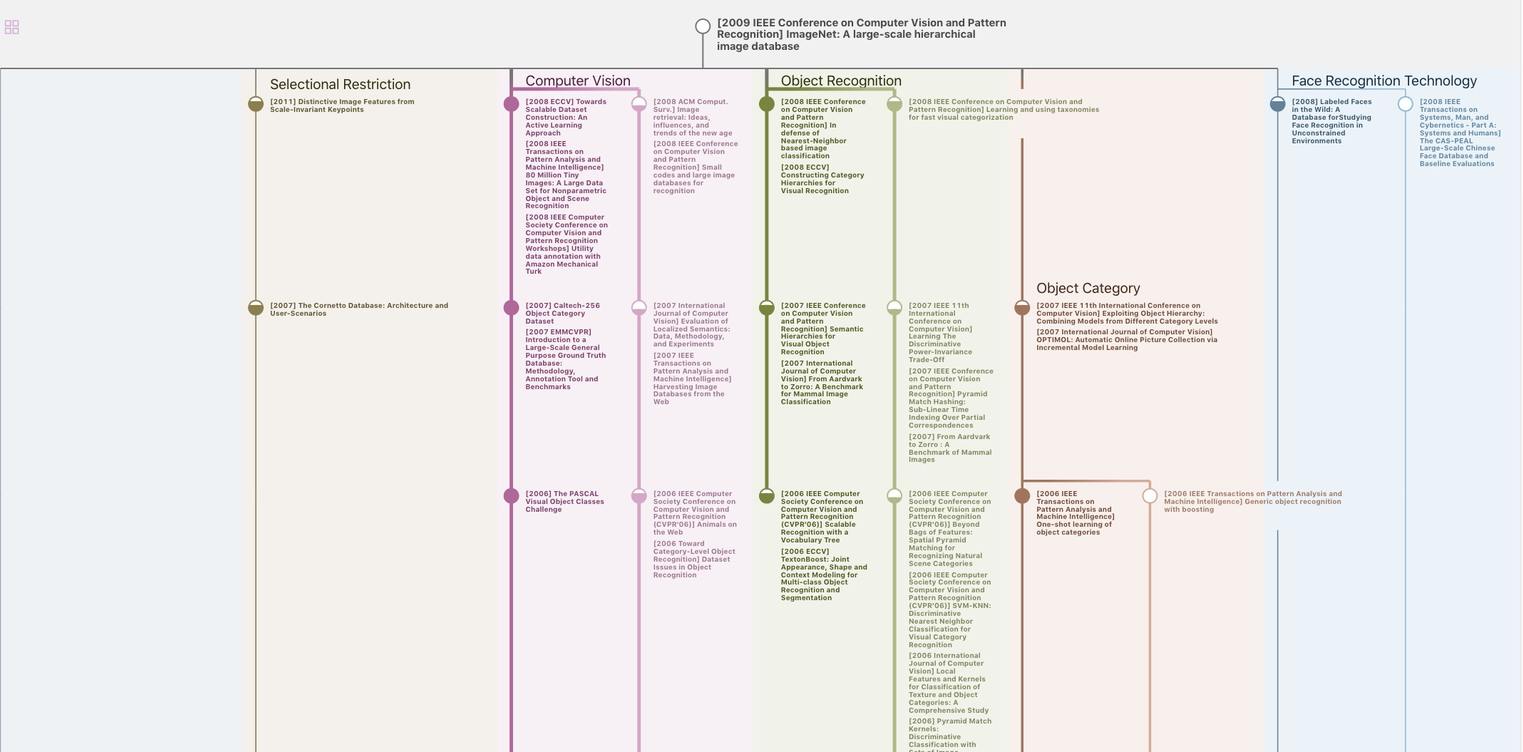
生成溯源树,研究论文发展脉络
Chat Paper
正在生成论文摘要