AutoHash: Learning Higher order Feature Interactions for Deep CTR Prediction
IEEE Transactions on Knowledge and Data Engineering(2020)
摘要
Feature combinations are essential for the success of many web applications, such as personalised recommendation and online advertising. State-of-the-art methods usually model explicit feature interactions to help neural networks reduce the number of parameters and achieve better performance. However, their explicit feature interactions are often restricted to the second-order due to computational complexity. In this work, we propose efficient ways to represent explicit high-order feature combinations as well as prune redundant features in the mean time. To begin with, we make novel use of the Count Sketch algorithm within a DNN classifier such that high-order feature combinations can be compactly represented. After that, to combat the problem of redundant features which degrade the prediction performance, we introduce an adaptive hashing algorithm, AutoHash, which can automatically select meaningful …
更多查看译文
关键词
Neural networks,Frequency modulation,Kernel,Feature extraction,Computational modeling,Training,Recommender systems
AI 理解论文
溯源树
样例
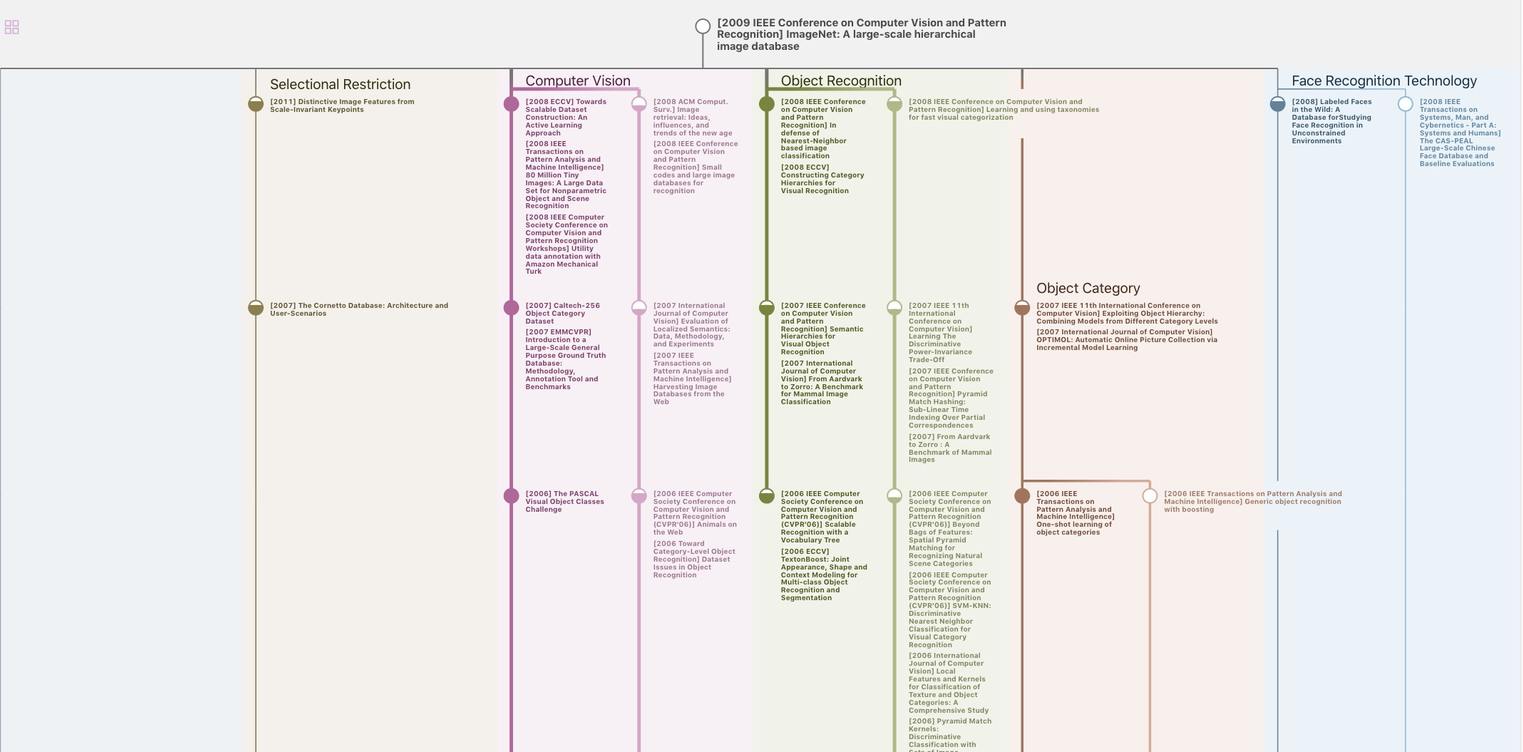
生成溯源树,研究论文发展脉络
Chat Paper
正在生成论文摘要