DE-GAN: A Conditional Generative Adversarial Network for Document Enhancement
IEEE Transactions on Pattern Analysis and Machine Intelligence(2022)
摘要
Documents often exhibit various forms of degradation, which make it hard to be read and substantially deteriorate the performance of an OCR system. In this paper, we propose an effective end-to-end framework named document enhancement generative adversarial networks (DE-GAN) that uses the conditional GANs (cGANs) to restore severely degraded document images. To the best of our knowledge, this practice has not been studied within the context of generative adversarial deep networks. We demonstrate that, in different tasks (document clean up, binarization, deblurring and watermark removal), DE-GAN can produce an enhanced version of the degraded document with a high quality. In addition, our approach provides consistent improvements compared to state-of-the-art methods over the widely used DIBCO 2013, DIBCO 2017, and H-DIBCO 2018 datasets, proving its ability to restore a degraded document image to its ideal condition. The obtained results on a wide variety of degradation reveal the flexibility of the proposed model to be exploited in other document enhancement problems.
更多查看译文
关键词
Document analysis,document enhancement,degraded document binarization,watermark removal,deep learning,generative adversarial networks
AI 理解论文
溯源树
样例
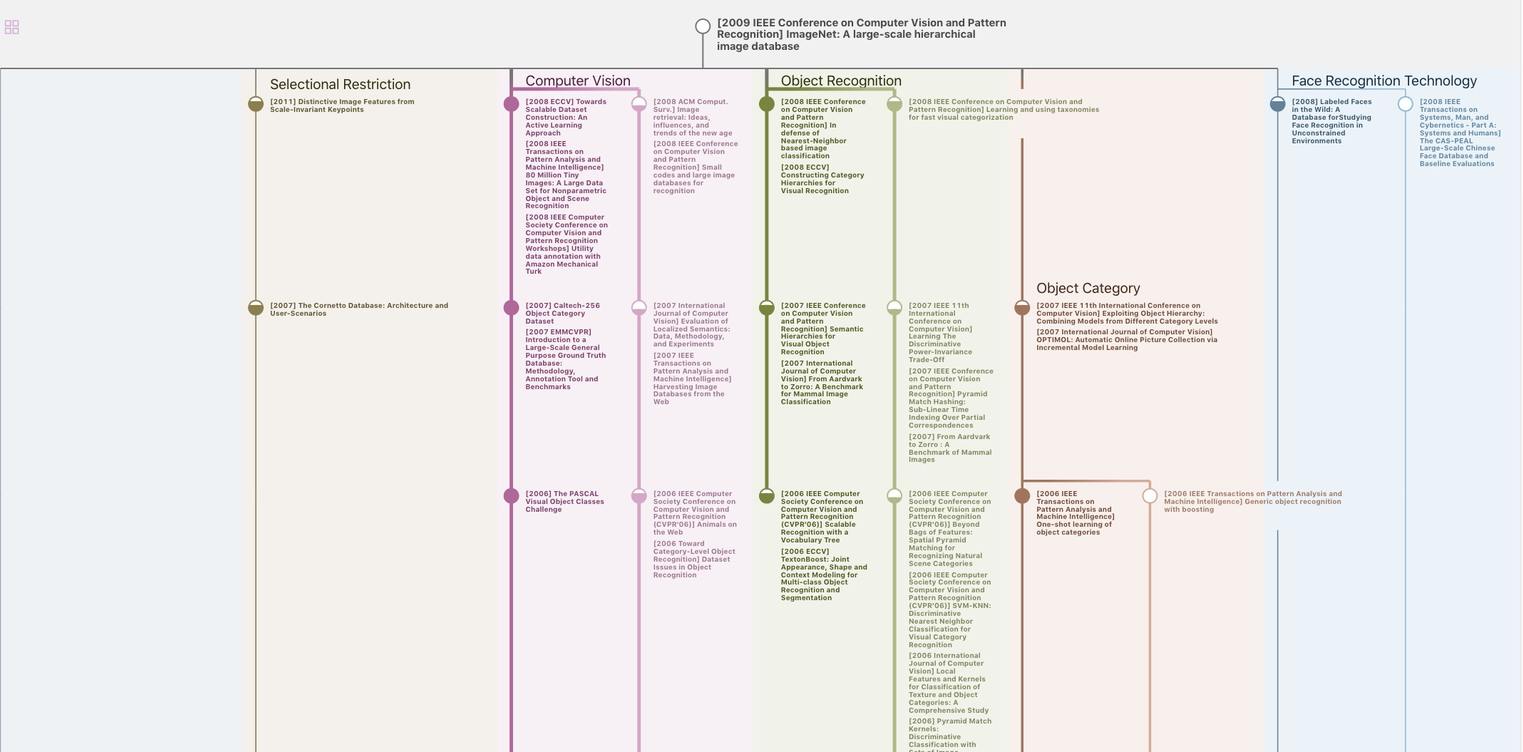
生成溯源树,研究论文发展脉络
Chat Paper
正在生成论文摘要