LaSOT: A High-quality Large-scale Single Object Tracking Benchmark
INTERNATIONAL JOURNAL OF COMPUTER VISION(2020)
摘要
Despite great recent advances in visual tracking, its further development, including both algorithm design and evaluation, is limited due to lack of dedicated large-scale benchmarks. To address this problem, we present LaSOT , a high-quality La rge-scale S ingle O bject T racking benchmark. LaSOT contains a diverse selection of 85 object classes, and offers 1550 totaling more than 3.87 million frames. Each video frame is carefully and manually annotated with a bounding box. This makes LaSOT, to our knowledge, the largest densely annotated tracking benchmark. Our goal in releasing LaSOT is to provide a dedicated high quality platform for both training and evaluation of trackers. The average video length of LaSOT is around 2500 frames, where each video contains various challenge factors that exist in real world video footage,such as the targets disappearing and re-appearing. These longer video lengths allow for the assessment of long-term trackers. To take advantage of the close connection between visual appearance and natural language, we provide language specification for each video in LaSOT. We believe such additions will allow for future research to use linguistic features to improve tracking. Two protocols, full-overlap and one-shot , are designated for flexible assessment of trackers. We extensively evaluate 48 baseline trackers on LaSOT with in-depth analysis, and results reveal that there still exists significant room for improvement. The complete benchmark, tracking results as well as analysis are available at http://vision.cs.stonybrook.edu/~lasot/ .
更多查看译文
关键词
Visual tracking, Large-scale benchmark, High-quality dense annotation, Tracking evaluation
AI 理解论文
溯源树
样例
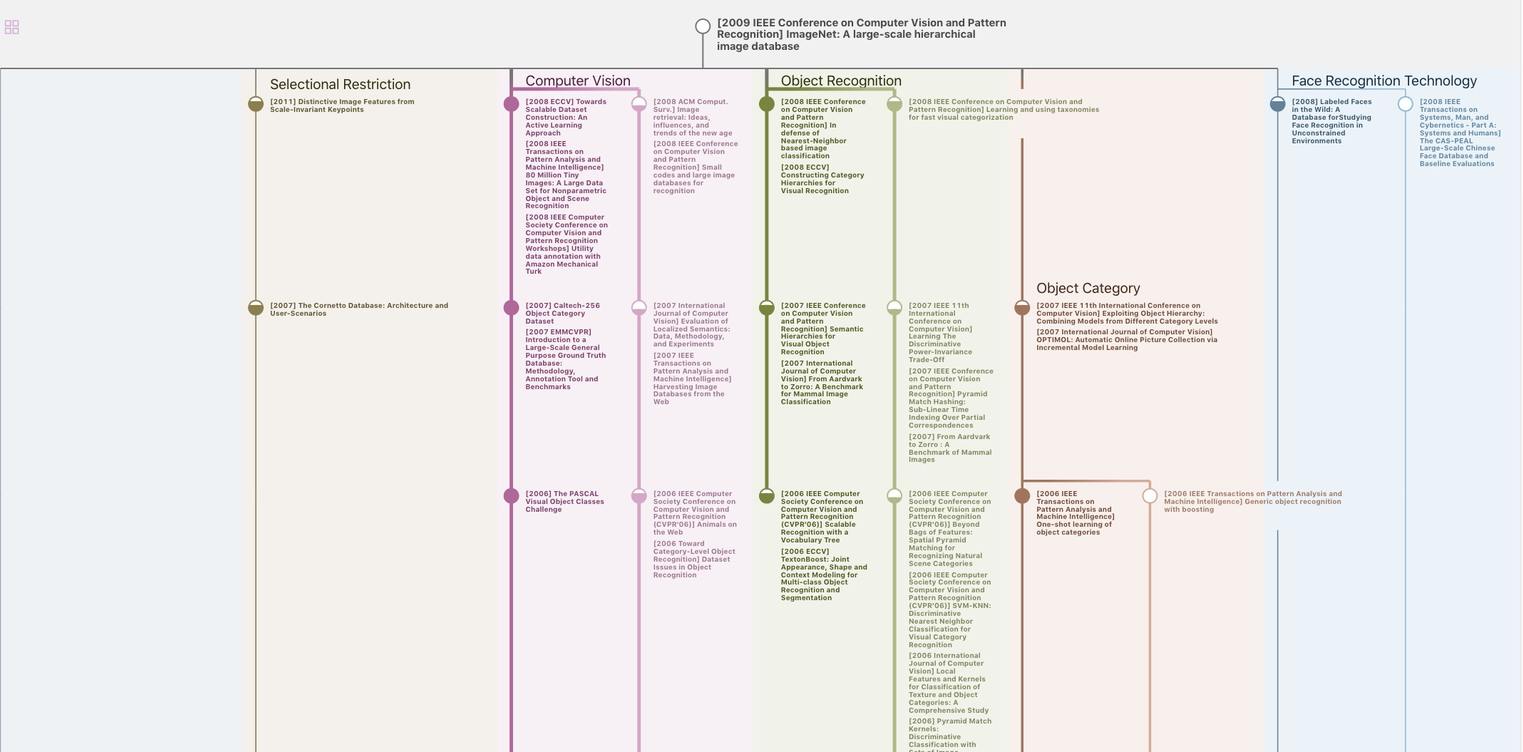
生成溯源树,研究论文发展脉络
Chat Paper
正在生成论文摘要