Sub-linear Regret Bounds for Bayesian Optimisation in Unknown Search Spaces.
NeurIPS(2020)
摘要
Bayesian optimisation is a popular method for efficient optimisation of expensive black-box functions. Traditionally, BO assumes that the search space is known. However, in many problems, this assumption does not hold. To this end, we propose a novel BO algorithm which expands (and shifts) the search space over iterations based on controlling the expansion rate thought a hyperharmonic series. Further, we propose another variant of our algorithm that scales to high dimensions. We show theoretically that for both our algorithms, the cumulative regret grows at sub-linear rates. Our experiments with synthetic and real-world optimisation tasks demonstrate the superiority of our algorithms over the current state-of-the-art methods for Bayesian optimisation in unknown search space.
更多查看译文
关键词
bayesian optimisation,unknown search spaces,sub-linear
AI 理解论文
溯源树
样例
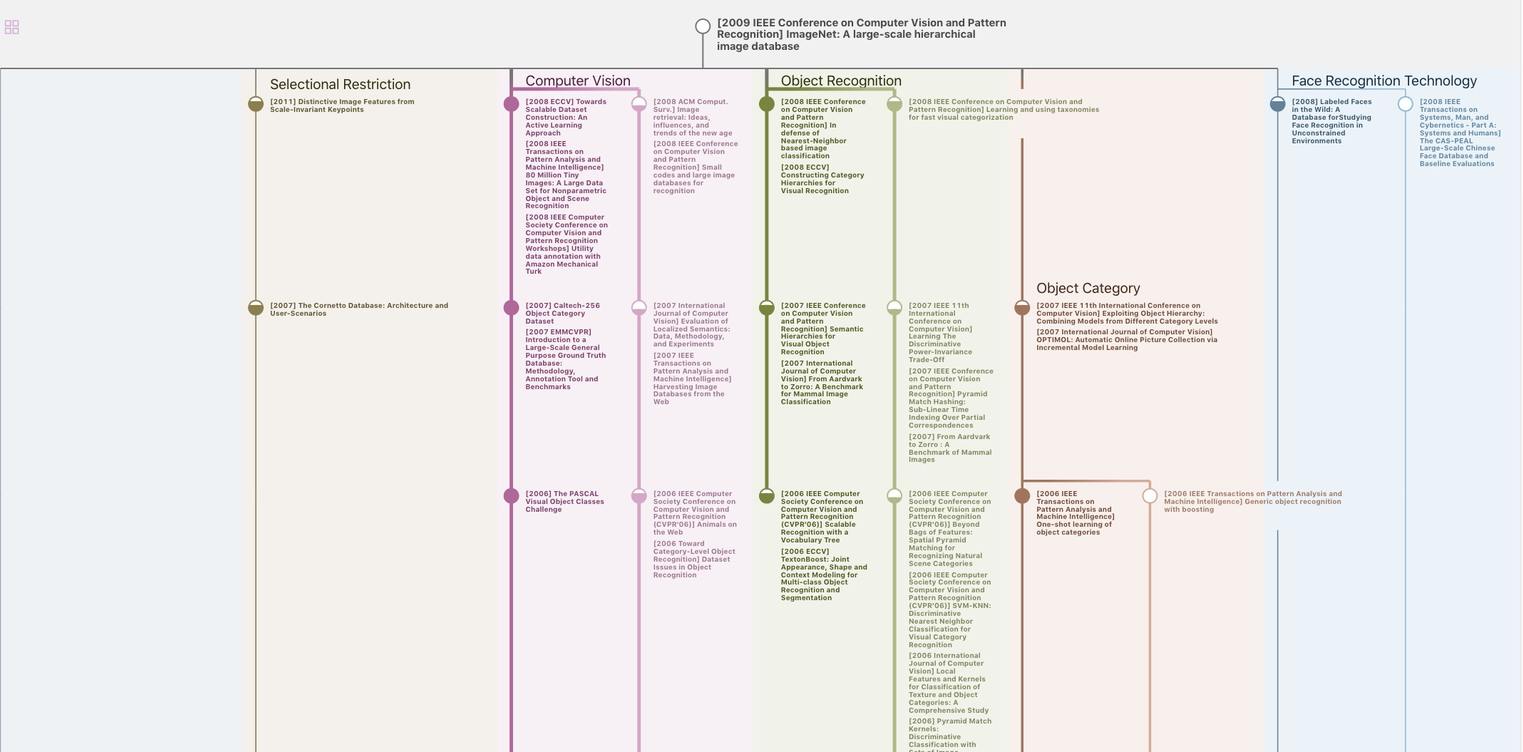
生成溯源树,研究论文发展脉络
Chat Paper
正在生成论文摘要