Player Identification in Hockey Broadcast Videos
Expert Systems with Applications(2021)
摘要
We present a deep recurrent convolutional neural network (CNN) approach to solve the problem of hockey player identification in NHL broadcast videos. Player identification is a difficult computer vision problem mainly because of the players’ similar appearance, occlusion, and blurry facial and physical features. However, we can observe players’ jersey numbers over time by processing variable length image sequences of players (aka ‘tracklets’). We propose an end-to-end trainable ResNet+LSTM network, with a residual network (ResNet) base and a long short-term memory (LSTM) layer, to discover spatio-temporal features of jersey numbers over time and learn long-term dependencies. Additionally, we employ a secondary 1-dimensional convolutional neural network classifier as a late score-level fusion method to classify the output of the ResNet+LSTM network. For this work, we created a new hockey player tracklet dataset that contains sequences of hockey player bounding boxes. This achieves an overall player identification accuracy score over 87% on the test split of our new dataset.
更多查看译文
关键词
Computer vision,Recurrent models,Convolutional neural networks,Sports player identification,Jersey numbers,Broadcast hockey videos
AI 理解论文
溯源树
样例
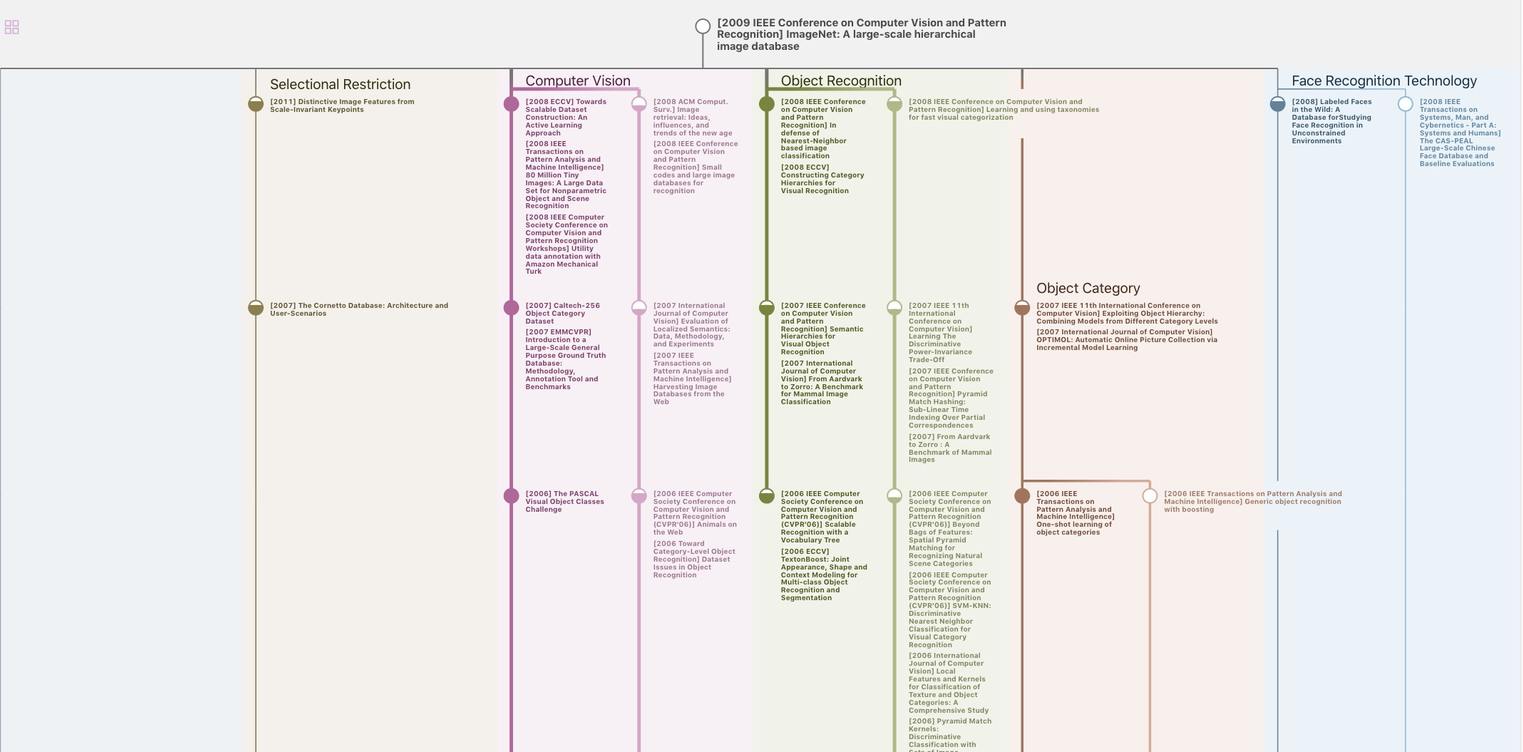
生成溯源树,研究论文发展脉络
Chat Paper
正在生成论文摘要