Leveraging Clickstream Trajectories to Reveal Low-Quality Workers in Crowdsourced Forecasting Platforms
arxiv(2020)
摘要
Crowdwork often entails tackling cognitively-demanding and time-consuming tasks. Crowdsourcing can be used for complex annotation tasks, from medical imaging to geospatial data, and such data powers sensitive applications, such as health diagnostics or autonomous driving. However, the existence and prevalence of underperforming crowdworkers is well-recognized, and can pose a threat to the validity of crowdsourcing. In this study, we propose the use of a computational framework to identify clusters of underperforming workers using clickstream trajectories. We focus on crowdsourced geopolitical forecasting. The framework can reveal different types of underperformers, such as workers with forecasts whose accuracy is far from the consensus of the crowd, those who provide low-quality explanations for their forecasts, and those who simply copy-paste their forecasts from other users. Our study suggests that clickstream clustering and analysis are fundamental tools to diagnose the performance of crowdworkers in platforms leveraging the wisdom of crowds.
更多查看译文
关键词
crowdsourced forecasting platforms,clickstream trajectories,workers,low-quality
AI 理解论文
溯源树
样例
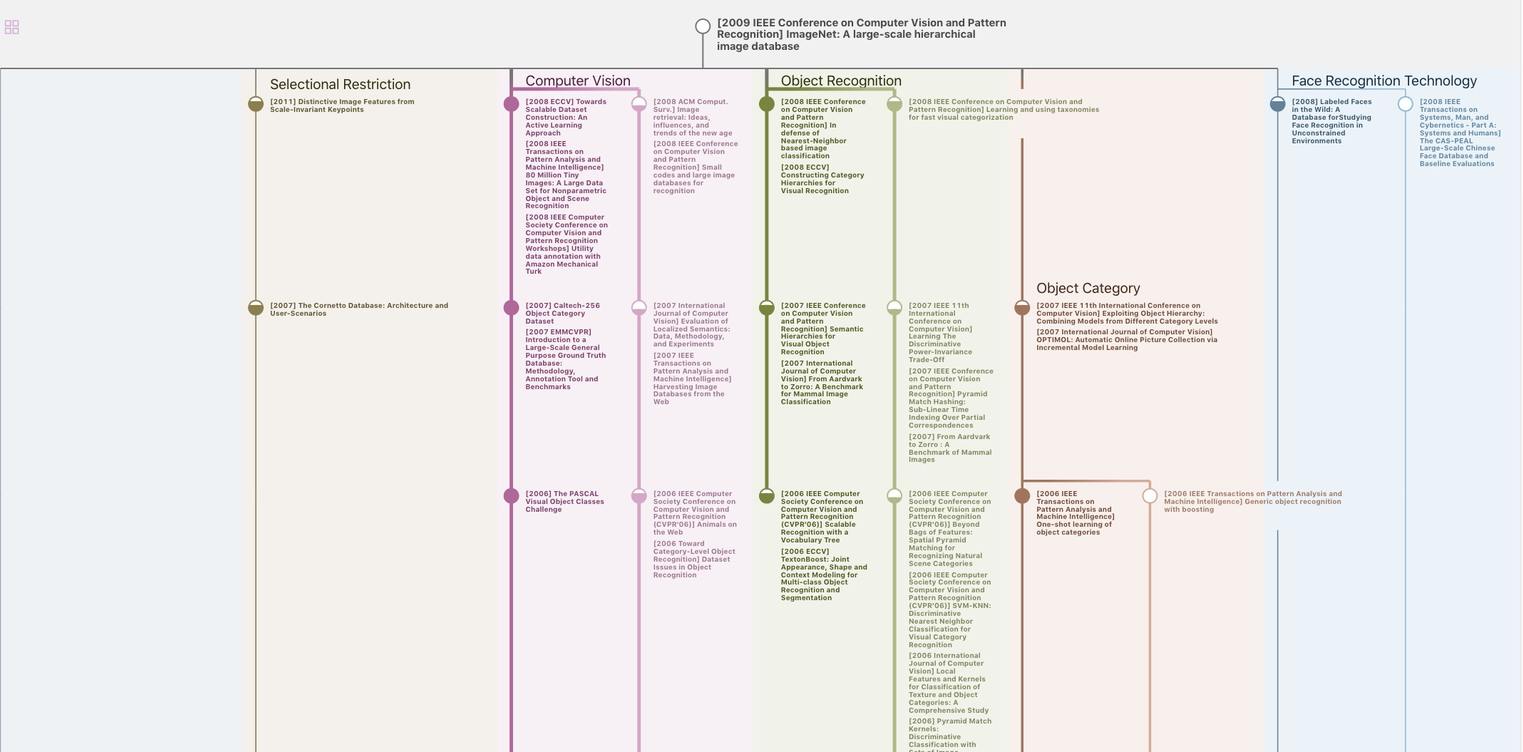
生成溯源树,研究论文发展脉络
Chat Paper
正在生成论文摘要