Stochastic optimization with adaptive restart: a framework for integrated local and global learning
JOURNAL OF GLOBAL OPTIMIZATION(2020)
摘要
A common approach to global optimization is to combine local optimization methods with random restarts. Restarts have been used as a performance boosting approach. They can be a means to avoid “slow progress” by exploiting a potentially good solution, and restarts can enable the potential discovery of multiple local solutions, thus improving the overall quality of the returned solution. A multi-start method is a way to integrate local and global approaches; where the global search itself can be used to restart a local search. Bayesian optimization methods aim to find global optima of functions that can only be point-wise evaluated by means of a possibly expensive oracle. We propose the stochastic optimization with adaptive restart (SOAR) framework, that uses the predictive capability of Gaussian process models as a means to adaptively restart local search and intelligently select restart locations with current information. This approach attempts to balance exploitation with exploration of the solution space. We study the asymptotic convergence of SOAR to a global optimum, and empirically evaluate SOAR performance through a specific implementation that uses the Trust Region method as the local search component. Numerical experiments show that the proposed algorithm outperforms existing methodologies over a suite of test problems of varying problem dimension with a finite budget of function evaluations.
更多查看译文
关键词
Stochastic search,Surrogate modeling,Local restart,Black-box optimization
AI 理解论文
溯源树
样例
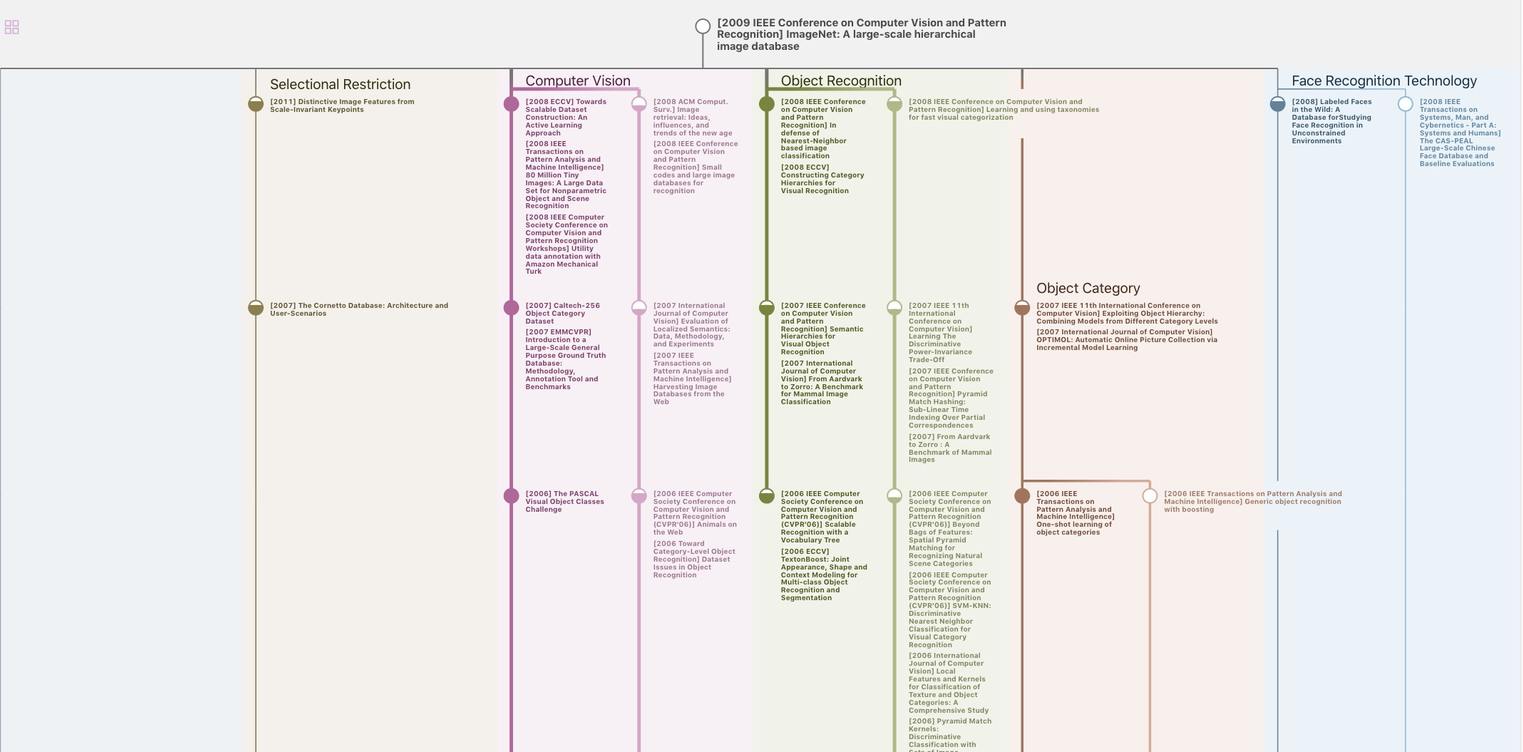
生成溯源树,研究论文发展脉络
Chat Paper
正在生成论文摘要