A weighted empirical Bayes risk prediction model using multiple traits.
STATISTICAL APPLICATIONS IN GENETICS AND MOLECULAR BIOLOGY(2020)
摘要
With rapid advances in high-throughput sequencing technology, millions of single-nucleotide variants (SNVs) can be simultaneously genotyped in a sequencing study. These SNVs residing in functional genomic regions such as exons may play a crucial role in biological process of the body. In particular, non-synonymous SNVs are closely related to the protein sequence and its function, which are important in understanding the biological mechanism of sequence evolution. Although statistically challenging, models incorporating such SNV annotation information can improve the estimation of genetic effects, and multiple responses may further strengthen the signals of these variants on the assessment of disease risk. In this work, we develop a new weighted empirical Bayes method to integrate SNV annotation information in a multi-trait design. The performance of this proposed model is evaluated in simulation as well as a real sequencing data; thus, the proposed method shows improved prediction accuracy compared to other approaches.
更多查看译文
关键词
empirical Bayes,next-generation sequencing,rare variants,risk prediction
AI 理解论文
溯源树
样例
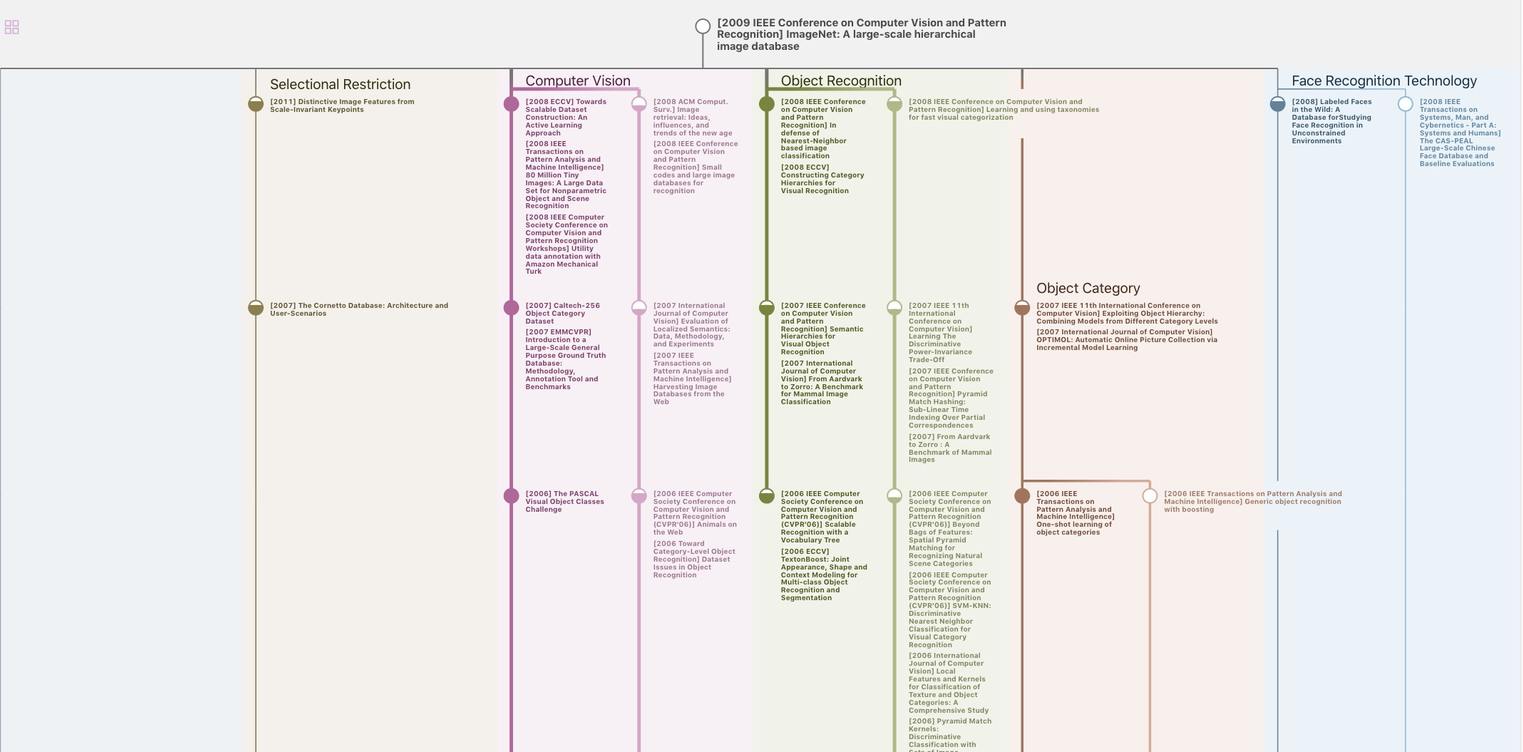
生成溯源树,研究论文发展脉络
Chat Paper
正在生成论文摘要