RNN-K: A Reinforced Newton Method for Consensus-Based Distributed Optimization and Control Over Multiagent Systems
IEEE Transactions on Cybernetics(2022)
摘要
With the rise of the processing power of networked agents in the last decade, second-order methods for machine learning have received increasing attention. To solve the distributed optimization problems over multiagent systems, Newton’s method has the benefits of fast convergence and high estimation accuracy. In this article, we propose a reinforced network Newton method with
$K$
-order control flexibility (RNN-K) in a distributed manner by integrating the consensus strategy and the latest knowledge across the network into local descent direction. The key component of our method is to make the best of intermediate results from the local neighborhood to learn global knowledge, not just for the consensus effect like most existing works, including the gradient descent and Newton methods as well as their refinements. Such a reinforcement enables revitalizing the traditional iterative consensus strategy to accelerate the descent of the Newton direction. The biggest difficulty to design the approximated Newton descent in distributed settings is addressed by using a special Taylor expansion that follows the matrix splitting technique. Based on the truncation on the Taylor series, our method also presents a tradeoff effect between estimation accuracy and computation/communication cost, which provides the control flexibility as a practical consideration. We derive theoretically the sufficient conditions for the convergence of the proposed RNN-K method of at least a linear rate. The simulation results illustrate the performance effectiveness by being applied to three types of distributed optimization problems that arise frequently in machine-learning scenarios.
更多查看译文
关键词
Consensus,distributed optimization,gradient descent,machine learning,Newton method
AI 理解论文
溯源树
样例
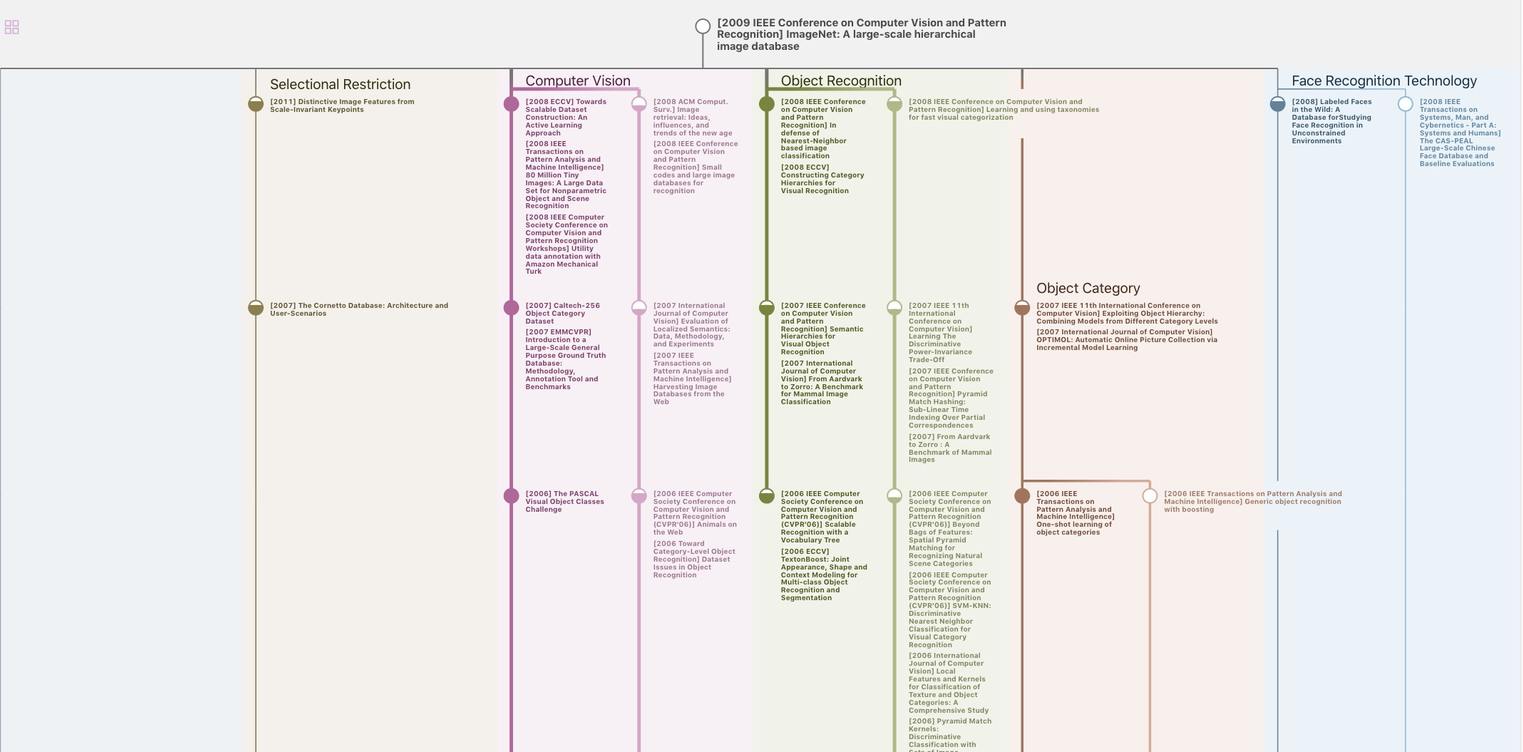
生成溯源树,研究论文发展脉络
Chat Paper
正在生成论文摘要