APPROXIMATE GENERALIZED INVERSES WITH ITERATIVE REFINEMENT FOR \bfitepsilon -ACCURATE PRECONDITIONING OF SINGULAR SYSTEMS\ast
SIAM JOURNAL ON MATRIX ANALYSIS AND APPLICATIONS(2022)
摘要
We introduce a new class of preconditioners to enable flexible GMRES to find a least-squares solution, and potentially the pseudoinverse solution, of large-scale sparse, asymmetric, singular, and potentially inconsistent systems. We develop the preconditioners based on a new observation that generalized inverses (i.e., Ag \in \{ G | AGA = A\} ) enable the preconditioned Krylov subspaces to converge in a single step. We then compute an approximate generalized inverse (AGI ) efficiently using a hybrid incomplete factorization (HIF), which combines multilevel incomplete LU with rank-revealing QR on its final Schur complement. We define the criteria of \epsilon -accuracy and stability of AGI to guarantee the convergence of preconditioned GMRES for consistent systems. For inconsistent systems, we fortify HIF with iterative refinement to obtain HIFIR, which allows accurate computations of the null-space vectors. By combining the two techniques, we then obtain a new solver, called PIPIT, for obtaining the pseudoinverse solutions for systems with low-dimensional null spaces. We demonstrate the robustness of HIF and HIFIR and show that they improve both accuracy and efficiency of the prior state of the art by orders of magnitude for systems with up to a million unknowns.
更多查看译文
关键词
rank-deficient least squares, pseudoinverse solution, generalized inverses, flexible Krylov subspaces, hybrid incomplete factorization, iterative refinement, variable preconditions
AI 理解论文
溯源树
样例
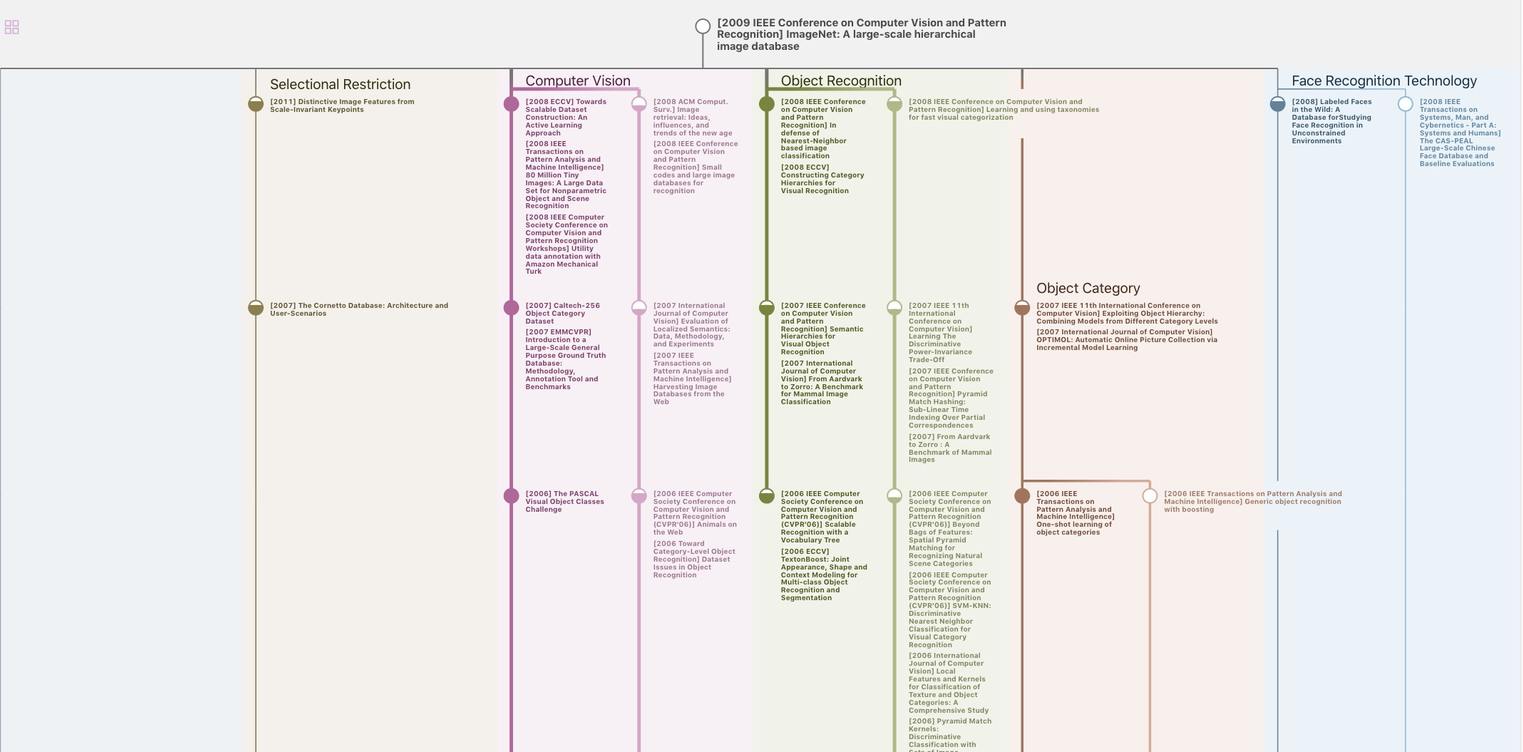
生成溯源树,研究论文发展脉络
Chat Paper
正在生成论文摘要