Data Programming by Demonstration: A Framework for Interactively Learning Labeling Functions
arxiv(2020)
摘要
Data programming is a programmatic weak supervision approach to efficiently curate large-scale labeled training data. Writing data programs (labeling functions) requires, however, both programming literacy and domain expertise. Many subject matter experts have neither programming proficiency nor time to effectively write data programs. Furthermore, regardless of one's expertise in coding or machine learning, transferring domain expertise into labeling functions by enumerating rules and thresholds is not only time consuming but also inherently difficult. Here we propose a new framework, data programming by demonstration (DPBD), to generate labeling rules using interactive demonstrations of users. DPBD aims to relieve the burden of writing labeling functions from users, enabling them to focus on higher-level semantics such as identifying relevant signals for labeling tasks. We operationalize our framework with Ruler, an interactive system that synthesizes labeling rules for document classification by using span-level annotations of users on document examples. We compare Ruler with conventional data programming through a user study conducted with 10 data scientists creating labeling functions for sentiment and spam classification tasks. We find that Ruler is easier to use and learn and offers higher overall satisfaction, while providing discriminative model performances comparable to ones achieved by conventional data programming.
更多查看译文
关键词
data programming,learning labeling functions
AI 理解论文
溯源树
样例
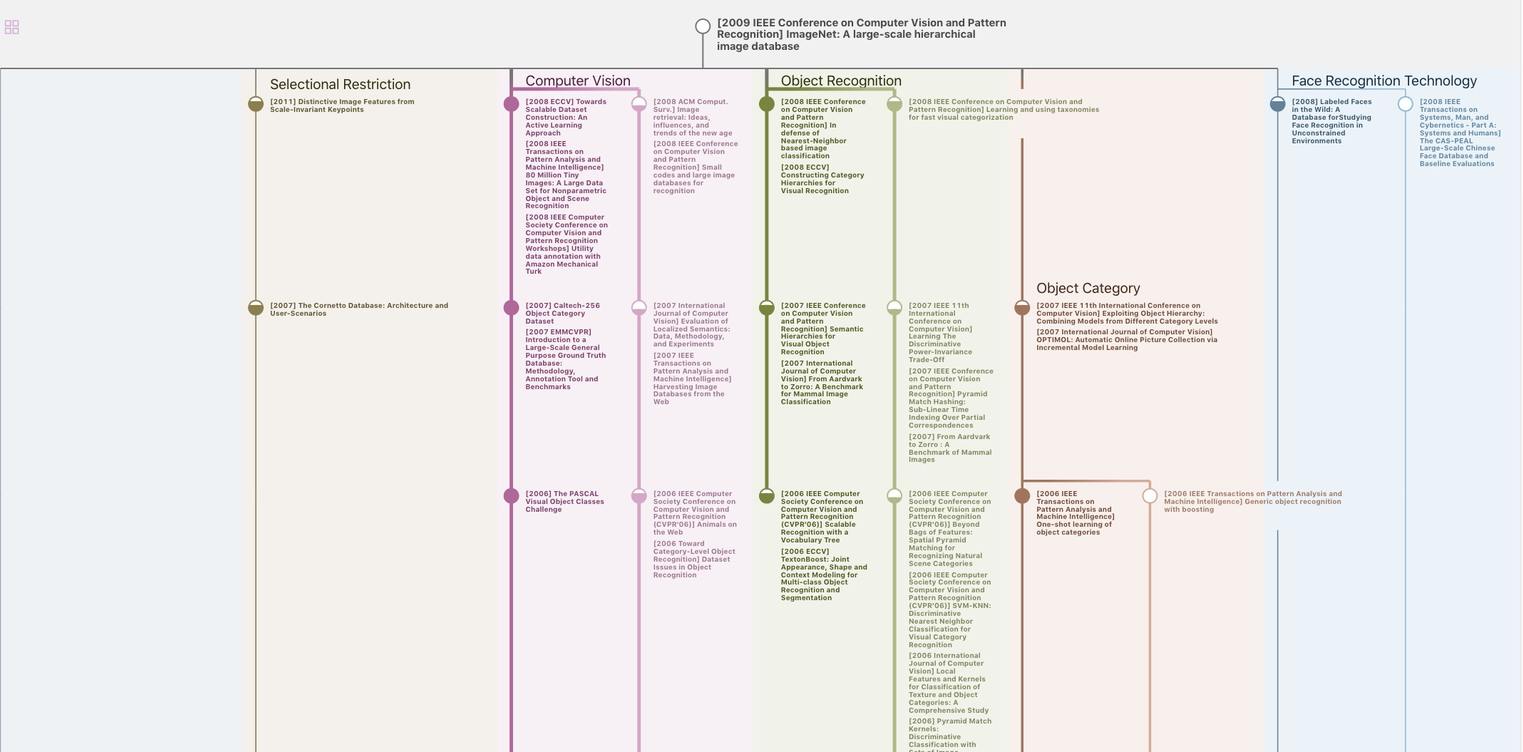
生成溯源树,研究论文发展脉络
Chat Paper
正在生成论文摘要