Cost-sensitive sample shifting in feature space
Pattern Analysis and Applications(2020)
摘要
The asymmetry of different misclassification costs is a common problem in many realistic applications. As one of the most familiar preprocessing methods, cost-sensitive resampling has drawn great attention due to its easy-implemented and universal properties. However, current methods mainly concentrate on changing the amount of the training set, which will alter the original distribution shapes and lead to the classifiers be over-fitted or unstable. For this case, a new method named cost-sensitive kernel shifting is proposed. The training data are remapped from the input space to the feature space by a particular kernel function, in which a distance metric is defined. Then the outliers are eliminated and the informative samples, including border and edge samples are selected due to the neighbor and geometrical information in the mapped space. Thirdly the positions of all the selected samples in the feature space are shifted. A moving step length is defined in proportion to both the ratio and different of the misclassification costs. In all steps only the kernel matrix is needed to be reshaped due to the kernel trick. Experiments on both synthetic and public datasets verify the effectiveness of the proposed methods.
更多查看译文
关键词
Cost-sensitive learning,Resampling,Feature space,SVM
AI 理解论文
溯源树
样例
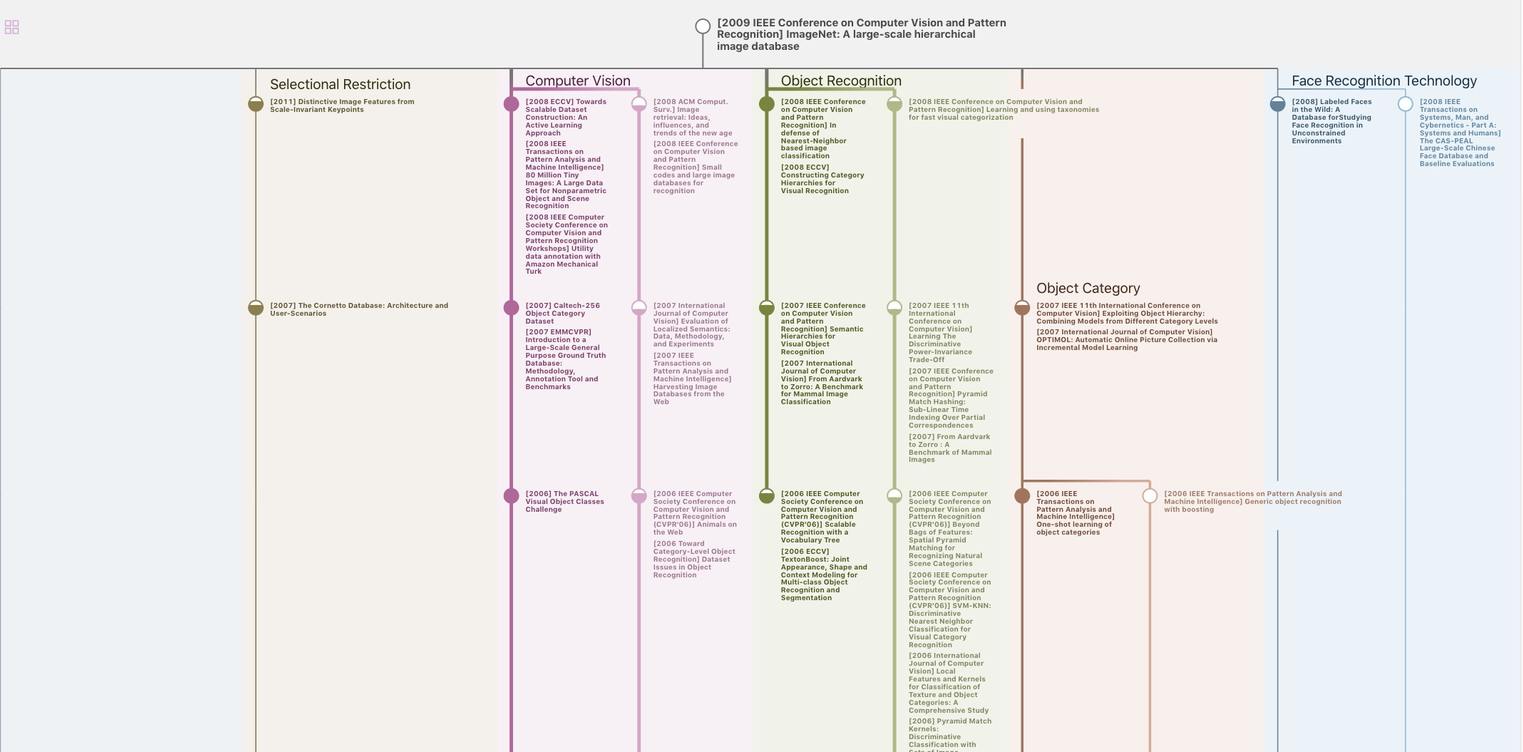
生成溯源树,研究论文发展脉络
Chat Paper
正在生成论文摘要