Left Ventricular Contraction Duration Is The Most Powerful Predictor Of Cardiac Events In Lqts: A Systematic Review And Meta-Analysis
JOURNAL OF CLINICAL MEDICINE(2020)
摘要
Background: Long-QT syndrome (LQTS) is primarily an electrical disorder characterized by a prolonged myocardial action potential. The delay in cardiac repolarization leads to electromechanical (EM) abnormalities, which adds a diagnostic value for LQTS. Prolonged left ventricular (LV) contraction was identified as a potential risk for arrhythmia. The aim of this meta-analysis was to assess the best predictor of all EM parameters for cardiac events (CEs) in LQTS patients. Methods: We systematically searched all electronic databases up to March 2020, to select studies that assessed the relationship between echocardiographic indices-contraction duration (CD), mechanical dispersion (MD), QRS onset to peak systolic strain (QAoC), and the EM window (EMW); and electrical indices- corrected QT interval (QT(C)), QT(C)dispersion, RR interval in relation to CEs in LQTS. This meta-analysis included a total of 1041 patients and 373 controls recruited from 12 studies. Results: The meta-analysis showed that LQTS patients had electrical and mechanical abnormalities as compared to controls-QT(C), WMD 72.8; QT(C)dispersion, WMD 31.7; RR interval, WMD 91.5; CD, WMD 49.2; MD, WMD 15.9; QAoC, WMD 27.8; and EMW, WMD -62.4. These mechanical abnormalities were more profound in symptomatic compared to asymptomatic patients in whom disturbances were already manifest, compared to controls. A CD >= 430 ms had a summary sensitivity (SS) of 71%, specificity of 84%, and diagnostic odds ratio (DOR) >19.5 in predicting CEs. EMW and QT(C)had a lower accuracy. Conclusions: LQTS is associated with pronounced EM abnormalities, particularly prolonged LV myocardial CD, which is profound in symptomatic patients. These findings highlight the significant role of EM indices like CD in managing LQTS patients.
更多查看译文
关键词
contraction duration, long-QT syndrome, electromechanical window, asymptomatic, symptomatic
AI 理解论文
溯源树
样例
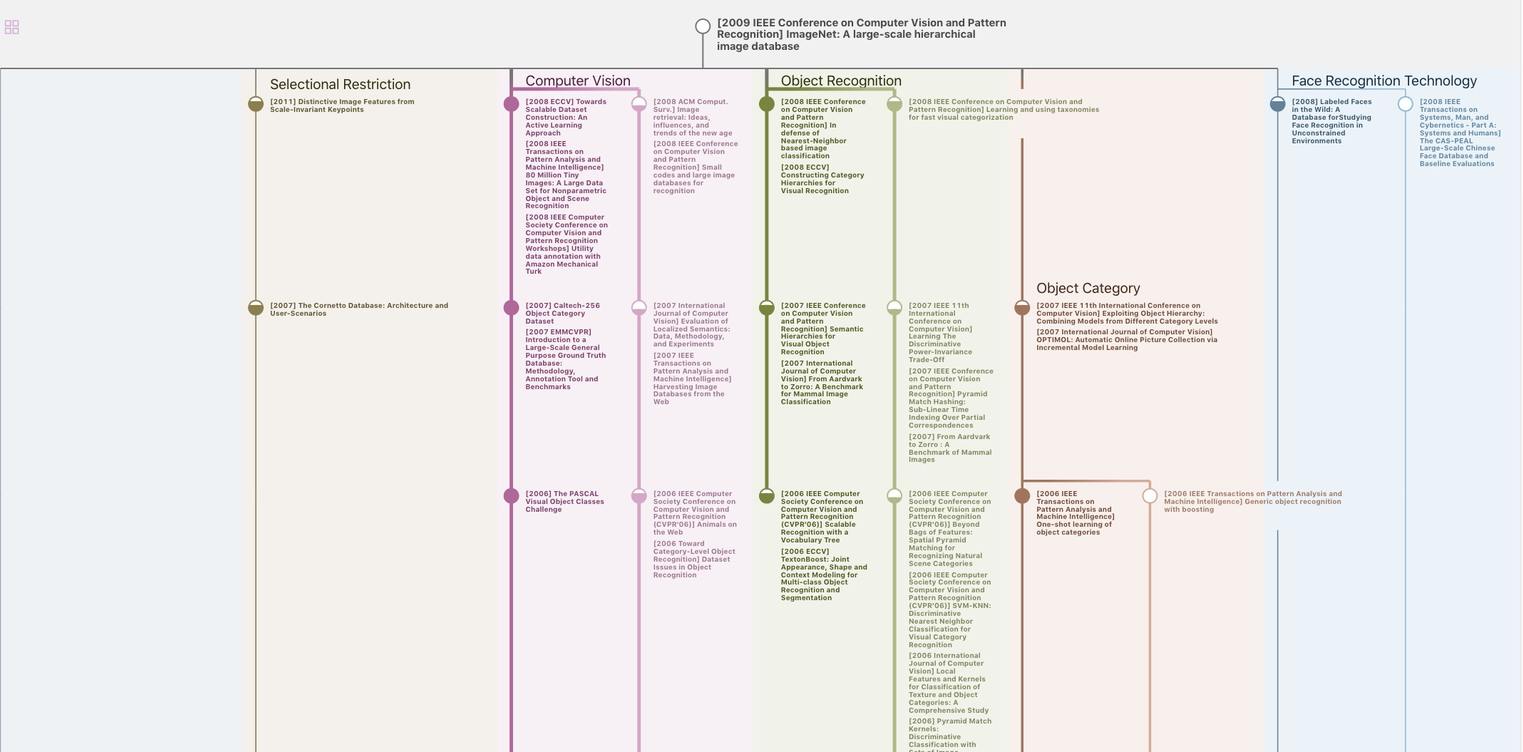
生成溯源树,研究论文发展脉络
Chat Paper
正在生成论文摘要