Gait: Gradient Adjusted Unsupervised Image-To-Image Translation
2020 IEEE INTERNATIONAL CONFERENCE ON IMAGE PROCESSING (ICIP)(2020)
摘要
Image-to-image translation (IIT) has made much progress recently with the development of adversarial learning. In most of the recent work, an adversarial loss is utilized to match the distributions of the translated and target image sets. However, this may create artifacts if two domains have different marginal distributions, for example, in uniform areas. In this work, we propose an unsupervised IIT method that preserves the uniform regions after the translation. The gradient adjustment loss, which is the L2 norm between the Sobel response of the target image and the adjusted Sobel response of the source images, is utilized. The proposed method is validated on the jellyfish-to-Haeckel dataset, which is prepared to demonstrate the mentioned problem, which contains images with different background distributions. We demonstrate that our method obtained a performance gain compared to the baseline method qualitatively and quantitatively, showing the effectiveness of the proposed method.
更多查看译文
关键词
Generative adversarial networks, image-to-image translation, domain adaptation, image processing
AI 理解论文
溯源树
样例
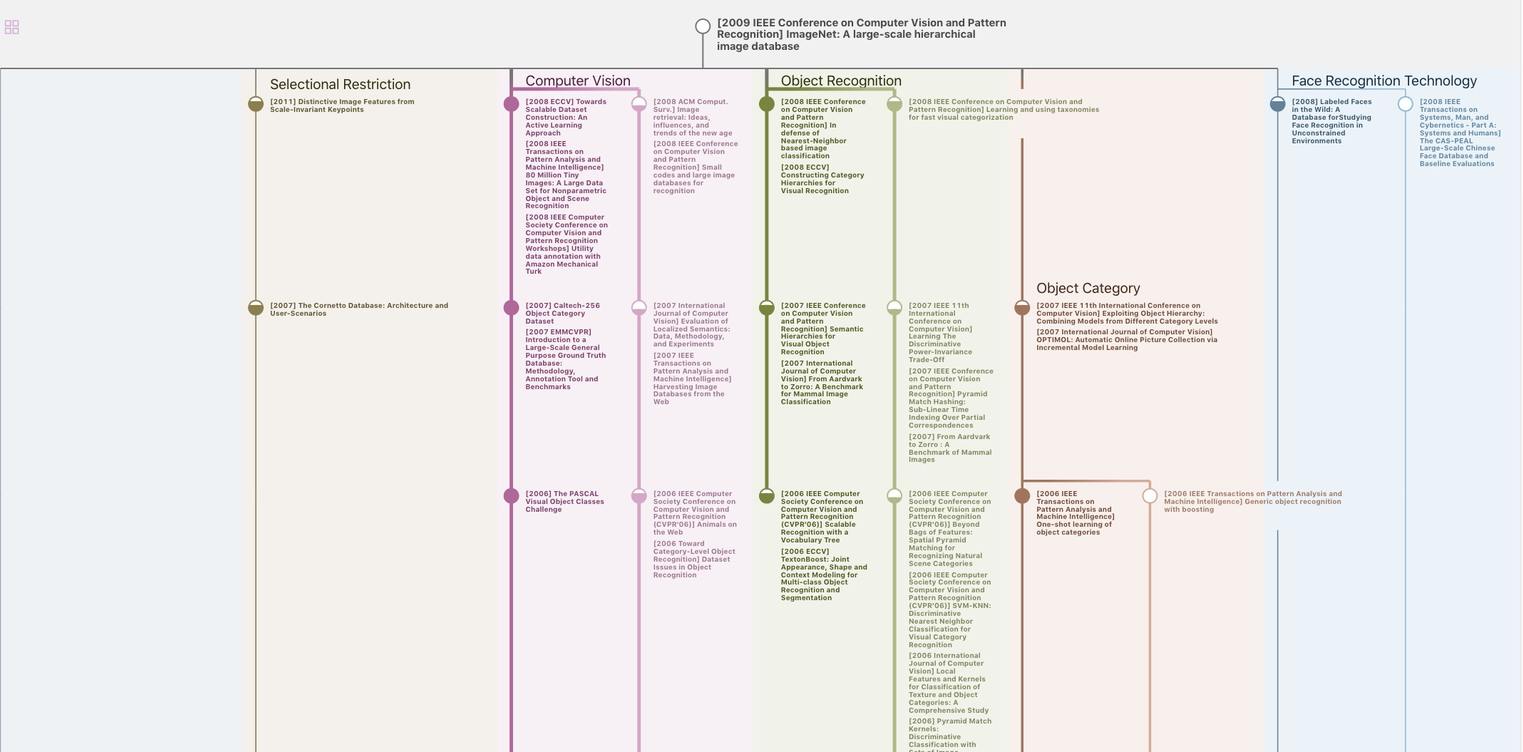
生成溯源树,研究论文发展脉络
Chat Paper
正在生成论文摘要