WaveGrad: Estimating Gradients for Waveform Generation
ICLR(2021)
摘要
This paper introduces WaveGrad, a conditional model for waveform generation through estimating gradients of the data density. This model is built on the prior work on score matching and diffusion probabilistic models. It starts from Gaussian white noise and iteratively refines the signal via a gradient-based sampler conditioned on the mel-spectrogram. WaveGrad is non-autoregressive, and requires only a constant number of generation steps during inference. It can use as few as 6 iterations to generate high fidelity audio samples. WaveGrad is simple to train, and implicitly optimizes for the weighted variational lower-bound of the log-likelihood. Empirical experiments reveal WaveGrad to generate high fidelity audio samples matching a strong likelihood-based autoregressive baseline with less sequential operations.
更多查看译文
AI 理解论文
溯源树
样例
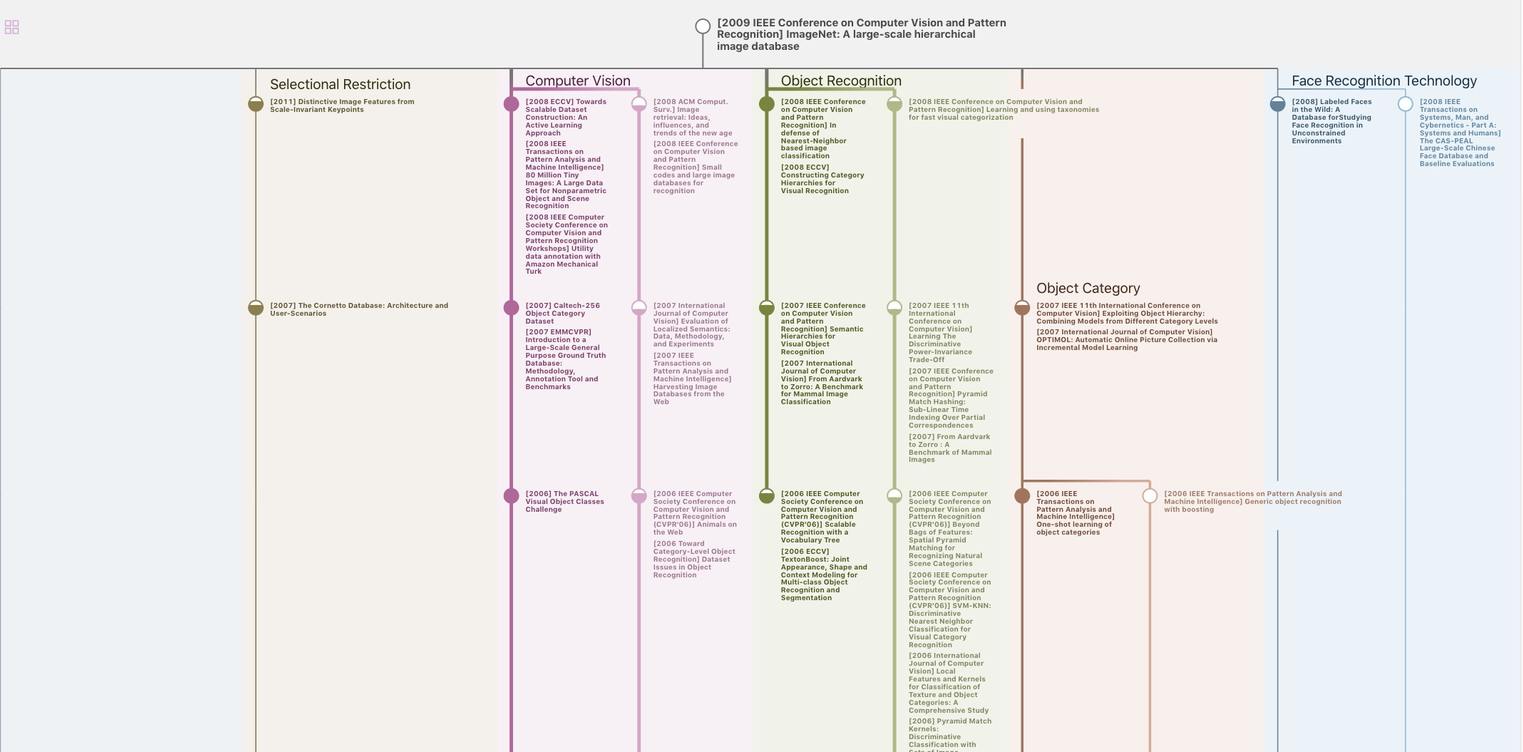
生成溯源树,研究论文发展脉络
Chat Paper
正在生成论文摘要