Application of incremental Gaussian mixture models for characterization of wind field data
STRUCTURAL HEALTH MONITORING 2013, VOLS 1 AND 2(2013)
摘要
Structural responses and power output of a wind turbine are strongly affected by the wind field acting on the wind turbine. Knowledge about the wind field and its variations is essential not only for designing, but also for cost-efficiently managing wind turbines. Wind field monitoring collects and stores wind field time series data. Over time the amount of data can be overwhelming. Furthermore, the correlation among the wind field statistical features is difficult to capture. Here, we explore the use of online machine learning to study the characteristics of wind fields, while effectively condensing the amount of monitoring data. In particular, incremental Gaussian mixture models (IGMM) are constructed to represent the joint probability density functions for wind field features, whose parameters are continuously updated as new data set is collected. The monitoring data recorded from an operating wind turbine in Germany is employed to test and compare the IGMM with conventional machine learning approach that uses an entire historical data set.
更多查看译文
关键词
incremental gaussian mixture models,wind
AI 理解论文
溯源树
样例
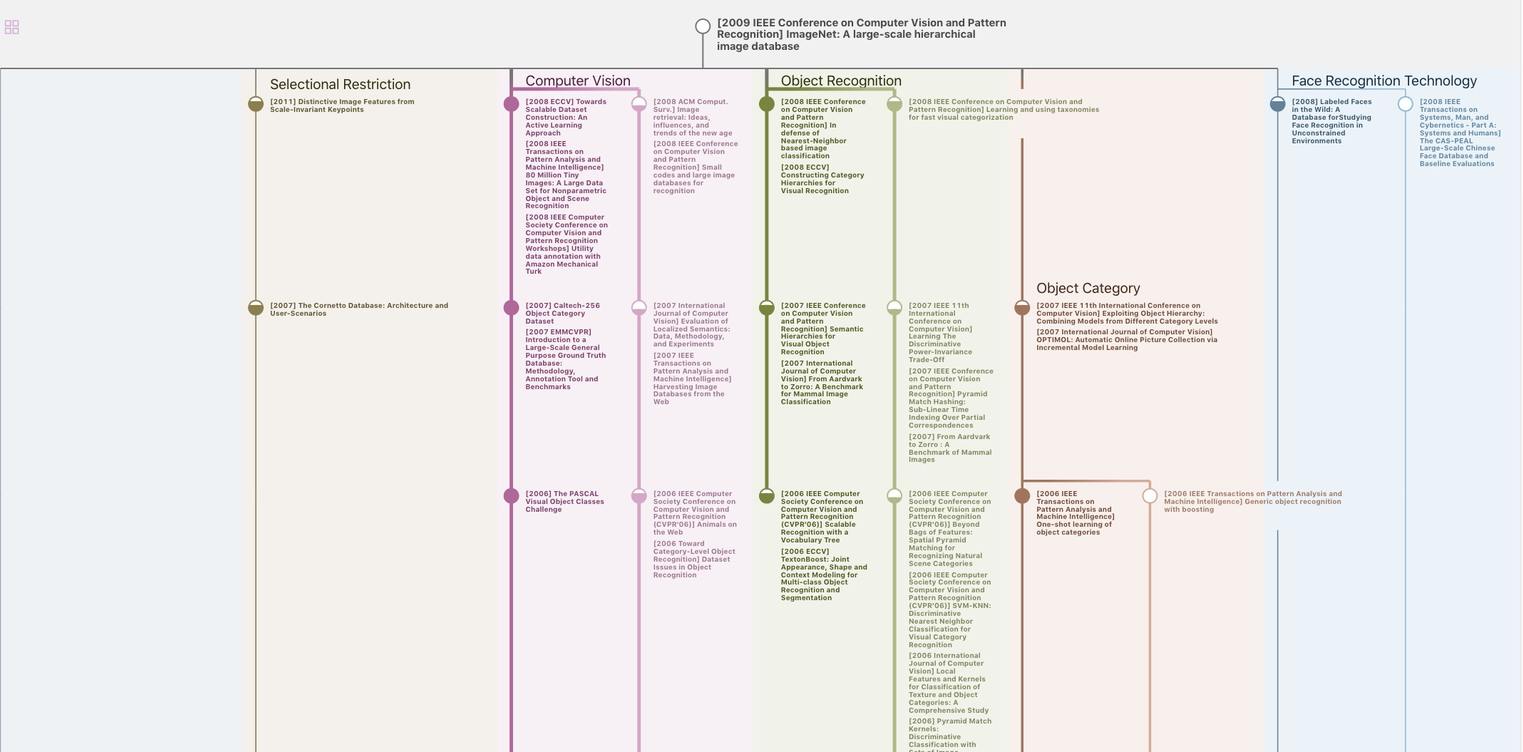
生成溯源树,研究论文发展脉络
Chat Paper
正在生成论文摘要